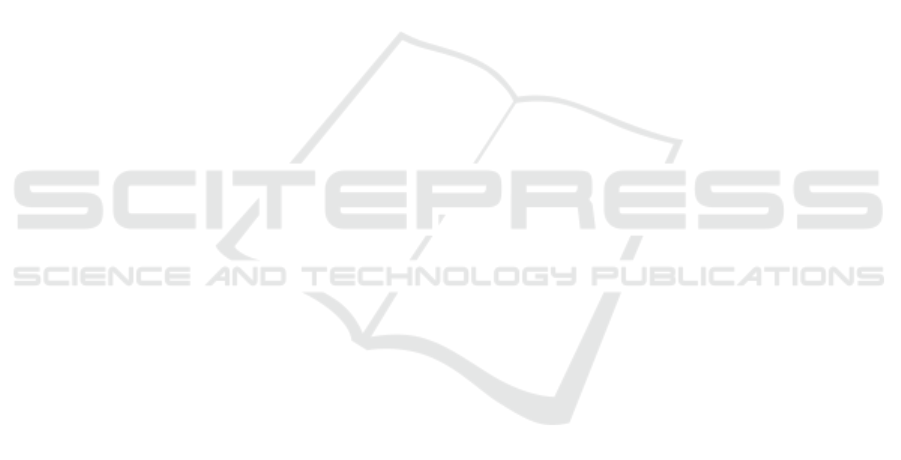
Cell Trajectory Clustering: Towards the Automated Identification of
Morphogenetic Fields in Animal Embryogenesis
Juan Raphael Diaz Simões
1,2
, Paul Bourgine
1,3
, Denis Grebenkov
2
and Nadine Peyriéras
1
1
BioEmergences USR3695, CNRS, Université Paris-Saclay, 91198 Gif-sur-Yvette Cedex, France
2
CNRS, Université Paris-Saclay, Route de Saclay, 92128 Palaiseau Cedex, France
3
Complex Systems Institute Paris Île-de-France UPS3611, CNRS, 113 rue Nationale, 75013 Paris, France
Keywords:
Animal Embryogenesis, Cell Lineage, Clustering, Path Integrals.
Abstract:
The recent availability of complete cell lineages from live imaging data opens the way to novel methodologies
for the automated analysis of cell dynamics in animal embryogenesis. We propose a method for the calcula-
tion of measure-based dissimilarities between cells. These dissimilarity measures allow the use of clustering
algorithms for the inference of time-persistent patterns. The method is applied to the digital cell lineages
reconstructed from live zebrafish embryos imaged from 6 to 13 hours post fertilization. We show that the
position and velocity of cells are sufficient to identify relevant morphological features including bilateral sym-
metry and coherent cell domains. The method is flexible enough to readily integrate larger sets of measures
opening the way to the automated identification of morphogenetic fields.
1 INTRODUCTION
Embryogenesis involves the formation of boundaries
between cell populations, which leads to the individu-
ation of cell compartments (Fagotto, 2014). Cells gat-
hered in the same compartment are expected to have
behavioral similarities, depending on the animal spe-
cies and the spatio temporal morphogenetic sequence.
The formation of morphogenetic fields and compart-
ments being a progressive phenomenon, similarities
in cell behaviors should be present at early stages.
Furthermore, behavioral similarities should be passed
from mother to daughters along the cell lineage.
In this context, the proposed similarity (or dissimi-
larity) measure of cell behavior should have the follo-
wing properties:
• It should be flexible enough to deal with different
sets of measures, different animal species and dif-
ferent periods of the organism development.
• It should consider the cell as a structure with a
temporal coherence.
• It should take into account the relative persistence
of cell characteristics along their lineage.
We propose a method to measure dissimilarities
between cells based on their behaviors along the cell
lineage. The dissimilarity calculation method is in-
spired from path integrals in quantum mechanics and
stochastic processes (Feynman, 1965) and is valid for
measures taken at the level of single cells throughout
the duration of their cell cycle.
This measure is further used for the automatic
clustering of cell trajectories.
The method is applied to the analysis of digital
cell lineage trees reconstructed from live zebrafish
embryos imaged in 3D+time. We show that a mi-
nimal set of parameters can lead to clusters highlig-
hting morphogenetic features from the organism bi-
lateral symmetry to finer cellular domains consistent
with the cell compartments that shape the presump-
tive organs.
2 ALGORITHM DESCRIPTION
The algorithm can be decomposed into the following
steps:
1. Extracting measures from input data. In the
case presented here, the data consisted in the
cell lineage reconstructed from partial 3D+time
imaging of developing zebrafish embryos (Faure
et al., 2016). The digital cell lineage provides the
cell positions in space and time. Velocities are cal-
culated using a discrete derivative similar to low-
pass filters (Holoborodko, 2008). This choice is
justified by the fact that the noise on the trajecto-
ries is unknown;
746
Simões, J., Bourgine, P., Grebenkov, D. and Peyriéras, N.
Cell Trajectory Clustering: Towards the Automated Identification of Morphogenetic Fields in Animal Embryogenesis.
DOI: 10.5220/0006259407460752
In Proceedings of the 6th International Conference on Pattern Recognition Applications and Methods (ICPRAM 2017), pages 746-752
ISBN: 978-989-758-222-6
Copyright
c
2017 by SCITEPRESS – Science and Technology Publications, Lda. All rights reserved