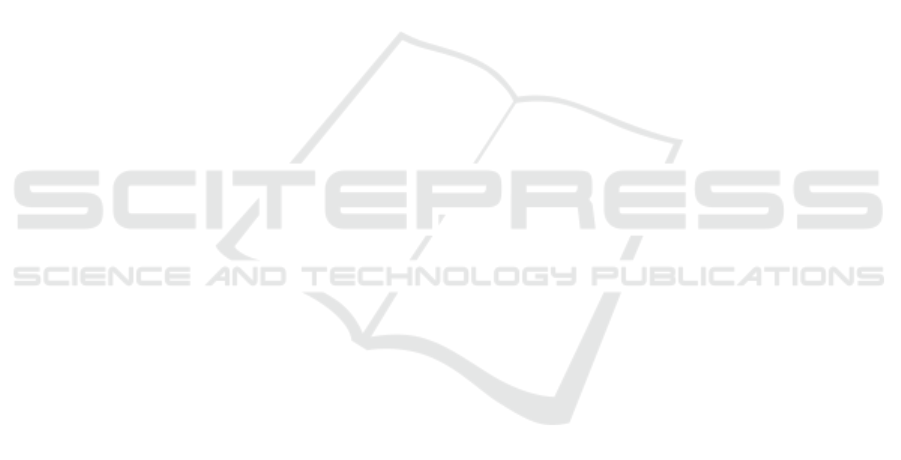
Cook, N. R. (2007). Use and Misuse of the Receiver Operat-
ing Characteristic Curve in Risk Prediction. Circula-
tion, 115(7):928–935.
Duda, R. O., Hart, P. E., and Stork, D. G. (2000). Pattern
Classification (2nd Edition). Wiley-Interscience.
Hand, D. J. (2009). Measuring classifier performance: a
coherent alternative to the area under the ROC curve.
Machine Learning, 77(1):103–123.
Hassan, N. U., Khalid, Y. I., Yuen, C., Huang, S., Pasha,
M. A., Wood, K. L., and Kerk, S. G. (2016). Frame-
work for minimum user participation rate determina-
tion to achieve specific demand response management
objectives in residential smart grids. International Jour-
nal of Electrical Power & Energy Systems, 74:91–103.
Hawarah, L., Ploix, S., and Jacomino, M. (2010). User
behavior prediction in energy consumption in housing
using bayesian networks. In Proc. of the 10th ICAISC,
pages 372–379, Berlin, Heidelberg. Springer-Verlag.
Heierman, III, E. O. and Cook, D. J. (2003). Improving
home automation by discovering regularly occurring
device usage patterns. In Proc. of the Third IEEE
Intern. Conf. on Data Mining, ICDM ’03, pages 537–
540, Washington, DC, USA. IEEE Computer Society.
Kang, Z., Jin, M., and Spanos, C. J. (2014). Modeling
of end-use energy profile: An appliance-data-driven
stochastic approach. In The 40th Annual Conf. of the
IEEE Industrial Electronics Society, Dallas, TX, USA,
pages 5382–5388.
Kelly, J. and Knottenbelt, W. (2015). The UK-DALE
dataset, domestic appliance-level electricity demand
and whole-house demand from five UK homes. Sci.
Data, (2):150007.
Kim, H., Marwah, M., Arlitt, M., Lyon, G., and Han, J.
(2011). Unsupervised Disaggregation of Low Fre-
quency Power Measurements. In Proceedings of the
2011 SIAM, pages 747–758.
Kolter, Z. and Johnson, M. J. (2011). REDD: A public data
set for energy disaggregation research. In Proc. of
SustKDD.
Lachut, D., Banerjee, N., and Rollins, S. (2014). Predictabil-
ity of energy use in homes. In IGCC, pages 1–10.
Lee, S., Ryu, G., Chon, Y., Ha, R., and Cha, H. (2013).
Automatic standby power management using usage
profiling and prediction. IEEE Transactions on Human-
Machine Systems, 43(6):535–546.
Makonin, S., Ellert, B., Bajic, I. V., and Popowich, F. (2016).
Electricity, water, and natural gas consumption of a res-
idential house in Canada from 2012 to 2014. Scientific
Data, 3(160037):1–12.
Makonin, S. and Popowich, F. (2015). Nonintrusive load
monitoring (NILM) performance evaluation. Energy
Efficiency, 8(4):809–814.
Mohsenian-Rad, A.-H. and Leon-Garcia, A. (2010). Optimal
residential load control with price prediction in real-
time electricity pricing environments. IEEE Trans.
Smart Grid, 1(2):120–133.
Monacchi, A., Egarter, D., Elmenreich, W., D’Alessandro,
S., and Tonello, A. M. (2014). GREEND: An energy
consumption dataset of households in Italy and Austria.
In IEEE Int. Conf. SmartGridComm, pages 511–516.
Parzen, E. (1962). On Estimation of a Probability Den-
sity Function and Mode. The Annals of Mathematical
Statistics, 33(3):1065–1076.
Pecan Street Inc. (2014). Dataport.
https://dataport.pecanstreet.org/.
Powers, D. M. W. (2011). Evaluation: From Precision,
Recall and F-Measure to ROC, Informedness, Marked-
ness & Correlation. Journal of Machine Learning
Technologies, 2(1):37–63.
S.a. (2005a). Benefits of demand response in electricity
markets and recommendations for archiving them. U.S.
Department of Energy.
S.a. (2005b). Demand response program evaluation - Final
report. Quantum Consulting Inc. and Summit Blue
Consulting, LLC Working Group 2 Measurement and
Evaluation Committee, California Edison Company.
Schleich, J. and Klobasa, M. (2013). How much shift in
demand? Findings from a field experiment in Ger-
many. In Lindström, T., editor, Rethink, renew, restart.
ECEEE 2013 Summer Study. Proc., pages 1919–1925.
European Council for an Energy-Efficient Economy.
Stephen, B., Galloway, S., and Burt, G. (2014). Self-learning
load characteristic models for smart appliances. IEEE
Transactions on Smart Grid, 5(5):2432–2439.
Truong, N. C., McInerney, J., Tran-Thanh, L., Costanza,
E., and Ramchurn, S. D. (2013a). Forecasting multi-
appliance usage for smart home energy management.
In Proc. of the Twenty-Third Int. Joint Conf. on Artifi-
cial Intelligence (IJCAI), pages 2908–2914. AAAI.
Truong, N. C., Tran-Thanh, L., Costanza, E., and Ramchurn,
S. D. (2013b). Towards appliance usage prediction
for home energy management. In Proc. of the Fourth
Intern. Conf. on Future Energy Systems, e-Energy ’13,
pages 287–288, New York, NY, USA. ACM.
Uttama Nambi, A. S., Reyes Lua, A., and Prasad, V. R.
(2015). LocED: Location-aware Energy Disaggrega-
tion Framework. In Proc. of the 2nd ACM Int. Conf.
on Embedded Systems for Energy-Efficient Built Envi-
ronments, BuildSys, pages 45–54, New York, USA.
Valverde-Albacete, F. J. and Peláez-Moreno, C. (2014).
100% Classification Accuracy Considered Harmful:
The Normalized Information Transfer Factor Explains
the Accuracy Paradox. PLOS ONE, 9(1):1–10.
Viswanath, S. K., Yuen, C., Tushar, W., Li, W. T., Wen, C. K.,
Hu, K., Chen, C., and Liu, X. (2016). System Design
of Internet-of-Things for Residential Smart Grid. IEEE
Wireless Communications, 23(5):90–98.
Zhu, X. and Davidson, I. (2007). Knowledge Discovery and
Data Mining: Challenges and Realities. Information
Science Reference, Hershey, New York.
SMARTGREENS 2017 - 6th International Conference on Smart Cities and Green ICT Systems
150