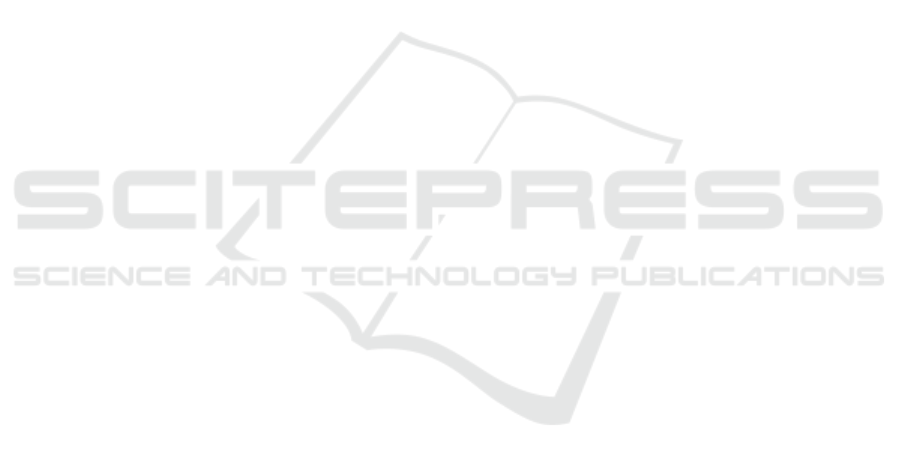
experts showed us that one does not have to be trained
in DR or advanced statistics to understand DR results.
Even though we conducted this study with only three
participants, we consider it as representative. In fu-
ture work, we plan to extend our prototype with more
advanced interaction concepts such as touch, the se-
lection of non-circular regions, and the integration of
different data sources. We encountered that LEAs
adapt rapidly to and bring forward current research.
Furthermore, we plan to extend our study to domains
such as finance or health care, also considering dif-
ferent DR approaches. Still, it is difficult to identify
experts who work with the data and analyze it, but
have not applied machine learning or advanced statis-
tics yet.
7 CONCLUSION
In this paper, we conducted a study to investigate
whether domain experts, untrained in advanced statis-
tics, can interpret the 2D depiction of DR results.
Several approaches to improve the understanding of
multivariate data for domain experts have been pub-
lished in recent years. However, and to the best of
our knowledge, proposed approaches have not been
evaluated with domain specific data together with un-
trained domain experts. Our study shows that the do-
main experts of a LEA effectively adapt to abstract
representations of the data if they are familiar with
the tasks and the type of data.
ACKNOWLEDGEMENTS
We like to thank the LEA data analysts for their par-
ticipation in the study and their feedback during the
sessions. This work was partly supported by the EU
project Visual Analytics for Sense-making in Crimi-
nal Intelligence Analysis (VALCRI) under grant num-
ber FP7-SEC-2013-608142 and the German Research
Foundation (DFG) within projects A03 and C01 of
SFB/Transregio 161.
REFERENCES
Agency, N. P. I. (2008). Practice advice on analysis. Tech-
nical report, Association of Chief Police Officers by
the National Policing Improvement Agency.
Alsallakh, B., Aigner, W., Miksch, S., and Gr
¨
oller, M. E.
(2012). Reinventing the contingency wheel: Scalable
visual analytics of large categorical data. IEEE Trans.
Vis. Comput. Graph., 18(12):2849–2858.
Andreas Buja, Dianne Cook, D. F. S. (1996). Interactive
high-dimensional data visualization. Journal of Com-
putational and Graphical Statistics, 5(1):78–99.
Benz
´
ecri, J. (1973). L’analyse des donn
´
ees: L’analyse des
correspondances. L’analyse des donn
´
ees: lec¸ons sur
l’analyse factorielle et la reconnaissance des formes et
travaux. Dunod.
Bernard, J., Steiger, M., Widmer, S., L
¨
ucke-Tieke, H., May,
T., and Kohlhammer, J. (2014). Visual-interactive
exploration of interesting multivariate relations in
mixed research data sets. Comput. Graph. Forum,
33(3):291–300.
Boren, T. and Ramey, J. (2000). Thinking aloud: Reconcil-
ing theory and practice. IEEE transactions on profes-
sional communication, 43(3):261–278.
Brehmer, M. and Munzner, T. (2013). A multi-level ty-
pology of abstract visualization tasks. IEEE Trans.
Visualization and Computer Graphics (TVCG) (Proc.
InfoVis), 19(12):2376–2385.
Brown, E. T., Liu, J., Brodley, C. E., and Chang, R.
(2012). Dis-function: Learning distance functions in-
teractively. In 2012 IEEE Conference on Visual An-
alytics Science and Technology, VAST 2012, Seattle,
WA, USA, October 14-19, 2012, pages 83–92.
Cox, T. and Cox, A. (2000). Multidimensional Scaling, Sec-
ond Edition. Chapman & Hall/CRC Monographs on
Statistics & Applied Probability. CRC Press.
D
¨
ork, M., Carpendale, M. S. T., and Williamson, C. (2012).
Visualizing explicit and implicit relations of com-
plex information spaces. Information Visualization,
11(1):5–21.
Ellis, G. P. and Dix, A. J. (2006). An explorative analysis
of user evaluation studies in information visualisation.
In Proceedings of the 2006 AVI Workshop on BEyond
time and errors: novel evaluation methods for infor-
mation visualization, BELIV 2006, Venice, Italy, May
23, 2006, pages 1–7.
Fernstad, S. J., Shaw, J., and Johansson, J. (2013). Quality-
based guidance for exploratory dimensionality reduc-
tion. Information Visualization, 12(1):44–64.
Gower, J. C. (1971). A general coefficient of similarity and
some of its properties. Biometrics, 27(4):857–871.
Graham, R. L., Knuth, D. E., and Patashnik, O. (1994).
Concrete Mathematics: A Foundation for Computer
Science. Addison-Wesley Longman Publishing Co.,
Inc., Boston, MA, USA, 2nd edition.
Hinneburg, A., Aggarwal, C. C., and Keim, D. A. (2000).
What is the nearest neighbor in high dimensional
spaces? In VLDB 2000, Proceedings of 26th Interna-
tional Conference on Very Large Data Bases, Septem-
ber 10-14, 2000, Cairo, Egypt, pages 506–515.
Ingram, S., Munzner, T., Irvine, V., Tory, M., Bergner, S.,
and M
¨
oller, T. (2010). Dimstiller: Workflows for di-
mensional analysis and reduction. In Proceedings of
the IEEE Conference on Visual Analytics Science and
Technology, IEEE VAST 2010, Salt Lake City, Utah,
USA, 24-29 October 2010, part of VisWeek 2010,
pages 3–10.
Inselberg, A. (1985). The plane with parallel coordinates.
The Visual Computer, 1(2):69–91.
IVAPP 2017 - International Conference on Information Visualization Theory and Applications
174