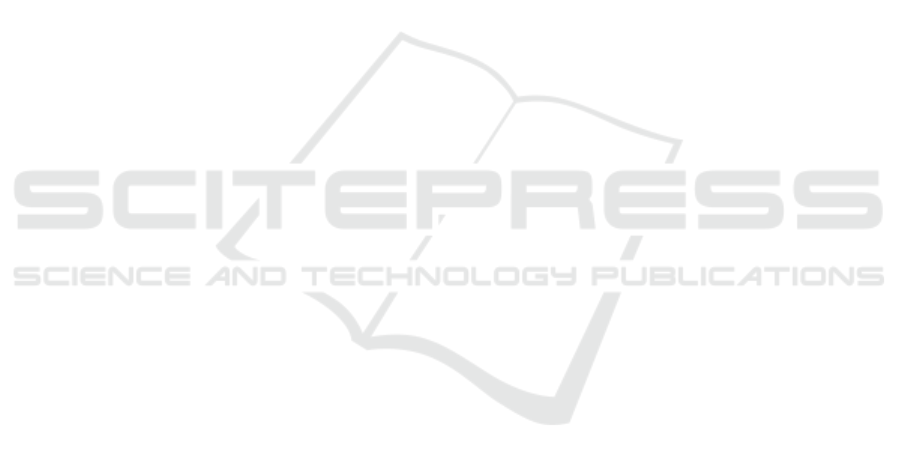
the assumed interests of the user. Also, IPAs usually
take environmental information, such as the location,
into account for recommendations of e.g. restaurants.
This gives the IPAs a rough awareness of the con-
text of the interaction, at least in the impression of
the user.
Proactive Behavior. Proactive behavior is often
combined with adaptation to the user or the environ-
ment. The IPA can use data of its user in order to
actively recommend content or services to the user.
Some example of Google’s Now are: The service can
infer from moving behavior and other information,
where its user works and offers navigation for the fas-
test way home on its dashboard when work is finished
(or it inferred, that work should have finished). Also,
it can find boarding cards in the user’s emails and
present it proactively (in the dashboard) on boarding
time. Proactive behavior is usually the expression of
an agenda or intend and therefore probably a feature
that adds strongly to the perceived human-likeness of
IPAs. But proactive behavior implies that the initi-
ative for an action comes from the system, thereby
taking away some control over the situation from the
user. This makes proactive behavior especially criti-
cal for highly reactant users, as explained later on.
Complex Feature Set. Sophisticated IPAs are not
only recommendation and conversation services, they
also feature a wide set of other functions. Thereby,
they act as an interface to other apps of the smart
phone or even devices of the smart home with which
they are connected. E.g. the feature set of Siri inclu-
des managing calendar entries, writing emails, wri-
ting notes, open calls, control smart home devices
and answer all kinds of requests from fact queries to
singing songs. The complex set of feature makes it
unpractical to thoroughly evaluate such a service in
terms of its usability properties, because all functions
would have to be evaluated under reliable conditions.
Also, it becomes impossible or at least not reasona-
ble for the user to grasp the complete functionality of
such a service. This is even amplified by the fact that
IPAs usually do not work locally but are web-based
services and new functionalities can be implemented,
changed or removed without knowledge of the user.
The uncertainty about the abilities of a service adds
to the human-likeness of interaction because the user
is not able to fully understand the limitations of that
service. This might imply an intelligent interaction
partner to the human.
Hierarchy. Humans are used to be in a supreme po-
sition towards the devices that they use. In the tra-
ditional interaction paradigm, humans are taking the
initiative and control the machines that they interact
with. In modern IPAs and some other services and
devices, this paradigm is not fully applicable, any-
more. Such services or devices sometimes take the
initiative themselves, or autonomously adapt to chan-
ges in the situation. They express a certain level of
autonomy, which might reduce the hierarchy gap bet-
ween the service or device and the user.
The above stated features of IPAs and some other
services and devices are reasons that make a broad,
systematic usability evaluation with traditional met-
hods a hard task. The results will be difficult to in-
terpret and reliability will be poor, because users will
react very diverse to many of those features. Just as
in human-human interaction, factors that are not mere
performance indices, such as behavioral patterns of
the service or social presence of an IPA might play an
increasing role alongside increasing human-likeness.
For example, a study with elderly people observed
that users’ reactions towards persuasive attempts are
more positive if the persuasive agent showed social
behavior, such as humans do (Looije et al., 2010).
On the other hand it was observed that highly cons-
cientiousness people like agents that show social be-
havior less, compared to other people (Looije et al.,
2010). In order to gain a more complete and meaning-
ful overview of such a system for both, sumative and
formative evaluation, new metrics are needed that can
access affective user states and personality traits and
thereby help to build heuristics about a user’s prefe-
rences. In this paper, it is argued, that such heuristics
can lead to more acceptance among users.
There are multiple character traits and affective
states that potentially play a role in the interaction be-
tween humans and human-like devices and services.
Among those, probably the big five personality traits
(Goldberg, 1993), that were used by Looije et al. to
investigate users’ preferences for agent based intelli-
gent assistants (Looije et al., 2010), are the most com-
monly known. For this work, however, reactance as a
personality trait is in focus. This is due to reactance
going along with diminished acceptance (Dillard and
Shen, 2005; Ehrenbrink et al., 2016a), what proba-
bly makes reactance one of the more important and
interesting factors for HCI developers. Further, the
discussion will mostly include reactance as a persona-
lity trait, in contrast to reactance as an affective state.
Personality traits can be used to create a personality
profile of a user which would need to be created only
once and would rarely require actualization. The as-
sessment of users’ state reactance would require con-
stant or very frequent measurements and can be con-
sidered as impractical for the lack of appropriate me-
The Benefits of Considering Psychological Reactance as Usersâ
˘
A
´
Z Personality Trait in HCI Research - Profiling Users with Hongâ
˘
A
´
Zs
Psychological Reactance Scale
159