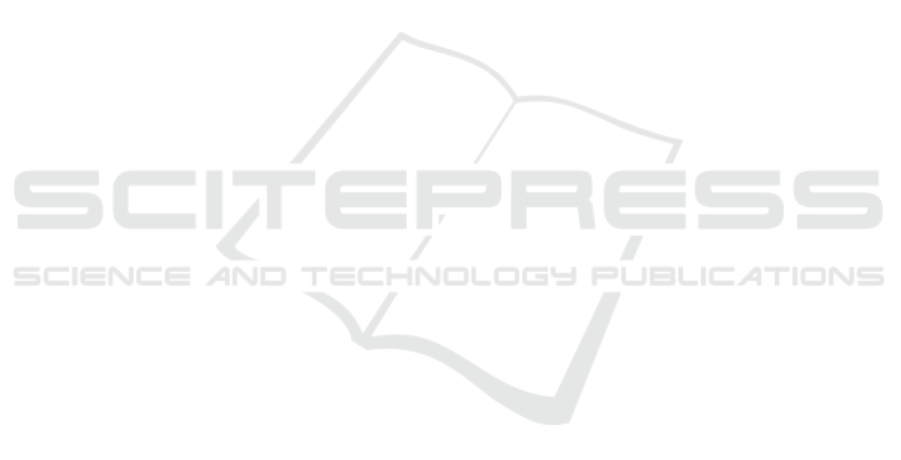
2004, volume 8, pages 622–629. Institute of Electrical
and Electronics Engineers Inc.
Bertini, E. and Santucci, G. (2006a). Give chance a
chance: modeling density to enhance scatter plot qua-
lity through random data sampling. Information Visu-
alization, 5(2):95–110.
Bertini, E. and Santucci, G. (2006b). Visual quality metrics.
In Proceedings of the 2006 AVI workshop on BEyond
time and errors: novel evaluation methods for infor-
mation visualization, pages 1–5. ACM.
Cottam, J. A. (2011). Design and implementation of a
stream-based visualization language. PhD thesis, In-
diana University.
Cottam, J. A., Lumsdaine, A., and Wang, P. (2014). Ab-
stract rendering: Out-of-core rendering for informa-
tion visualization. In Wong, P. C., Kao, D. L., Hao,
M. C., and Chen, C., editors, Conference on Visuali-
zation and Data Analysis, page 90170K. SPIE.
Fekete, J.-D. and Primet, R. (2016). Progressive analytics:
A computation paradigm for exploratory data analy-
sis. arXiv preprint arXiv:1607.05162.
Fisher, D., Popov, I., Drucker, S., et al. (2012). Trust me,
i’m partially right: incremental visualization lets ana-
lysts explore large datasets faster. In Proceedings of
the SIGCHI Conference on Human Factors in Com-
puting Systems, pages 1673–1682. ACM.
Forman, G. (2003). An extensive empirical study of fea-
ture selection metrics for text classification. Journal
of machine learning research, 3(Mar):1289–1305.
Frey, S., Sadlo, F., Ma, K.-L., and Ertl, T. (2014). Inte-
ractive progressive visualization with space-time error
control. IEEE TVCG, 20(12):2397–2406.
Glueck, M., Khan, A., and Wigdor, D. (2014). Dive in!
Enabling progressive loading for real-time navigation
of data visualizations. In CHI’14, pages 561–570.
ACM.
Harrison, L., Yang, F., Franconeri, S., and Chang, R.
(2014). Ranking visualizations of correlation using
weber’s law. IEEE transactions on visualization and
computer graphics, 20(12):1943–1952.
Heer, J. and Robertson, G. (2007). Animated transitions in
statistical data graphics. IEEE transactions on visua-
lization and computer graphics, 13(6):1240–1247.
Joy, K. I. (2009). Massive data visualization: A survey. In
M
¨
oller, T., Hamann, B., and Russel, R. D., editors,
Mathematical Foundations of Scientific Visualization,
Computer Graphics, and Massive Data Exploration,
pages 285–302. Springer.
Mulder, J. D., van Wijk, J. J., and van Liere, R. (1999). A
survey of computational steering environments. Fu-
ture Generation Computer Systems, 15(1):119–129.
Piringer, H., Tominski, C., Muigg, P., and Berger, W.
(2009). A multi-threading architecture to support inte-
ractive visual exploration. IEEE TVCG, 15(6):1113–
1120.
Rensink, R. A. and Baldridge, G. (2010). The perception
of correlation in scatterplots. In Computer Graphics
Forum, volume 29, pages 1203–1210. Wiley Online
Library.
Rosenbaum, R. and Schumann, H. (2009). Progressive re-
finement: More than a means to overcome limited
bandwidth. In VDA’09, page 72430I. SPIE.
Schulz, H.-J., Angelini, M., Santucci, G., and Schumann,
H. (2016). An enhanced visualization process mo-
del for incremental visualization. IEEE transactions
on visualization and computer graphics, 22(7):1830–
1842.
Stolper, C. D., Perer, A., and Gotz, D. (2014). Progressive
visual analytics: User-driven visual exploration of in-
progress analytics. IEEE TVCG, 20(12):1653–1662.
Szafir, D. A., Haroz, S., Gleicher, M., and Franconeri, S.
(2016). Four types of ensemble coding in data visua-
lizations. Journal of Vision, 16(5):11.
Tatu, A., Bak, P., Bertini, E., Keim, D., and Schneidewind,
J. (2010). Visual quality metrics and human percep-
tion: an initial study on 2d projections of large multi-
dimensional data. In Proceedings of the International
Conference on Advanced Visual Interfaces, pages 49–
56. ACM.
Tufte, E. R. and Graves-Morris, P. (1983). The visual dis-
play of quantitative information, volume 2. Graphics
press Cheshire, CT.
Turkay, C., Kaya, E., Balcisoy, S., and Hauser, H. (2017).
Designing progressive and interactive analytics pro-
cesses for high-dimensional data analysis. IEEE Tran-
sactions on Visualization and Computer Graphics,
23(1):131–140.
Vo, H. T., Comba, J. L. D., Geveci, B., and Silva, C. T.
(2011). Streaming-enabled parallel data flow frame-
work in the Visualization ToolKit. IEEE Computing
in Science and Engineering, 13(5):72–83.
Ware, C. (2012). Information visualization: perception for
design. Elsevier.
Wong, P. C., Foote, H., Adams, D., Cowley, W., Leung,
L. R., and Thomas, J. (2004). Visualizing data stre-
ams. In Kovalerchuk, B. and Schwing, J., editors,
Visual and Spatial Analysis: Advances in Data Mi-
ning, Reasoning, and Problem Solving, pages 265–
291. Springer.
Zheng, S., Song, R., and Wen, J.-R. (2007). Template-
independent news extraction based on visual consis-
tency. In Proceedings of the National Conference on
Artificial Intelligence, volume 22, page 1507. Menlo
Park, CA; Cambridge, MA; London; AAAI Press;
MIT Press; 1999.
On Visual Stability and Visual Consistency for Progressive Visual Analytics
341