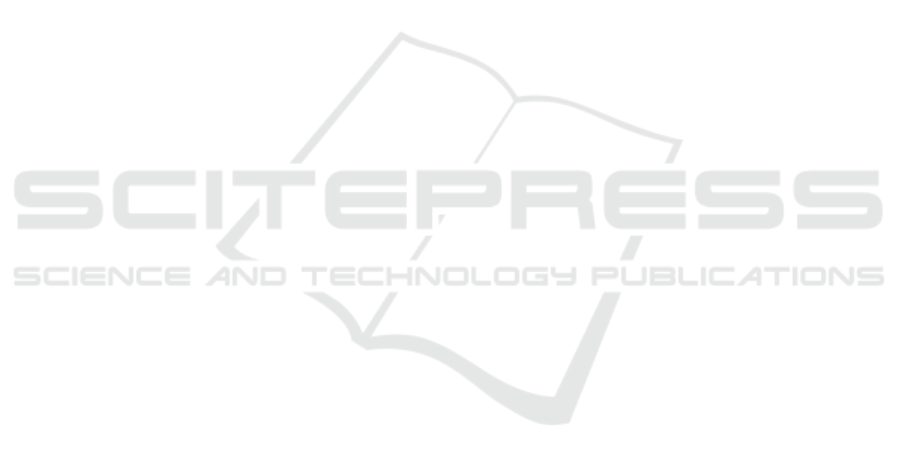
lens and is directed downwards. Therefore, it has
only recorded data from the road ahead of the vehi-
cle. This means that no data from the sky is available
and consequently can not be trained. Furthermore,
the training and test data set consists of only about
0.66% of data annotated as an obstacle. Accordingly,
the trained model is not capable of classifying obsta-
cles. This is also indicated in figure 5a where Gaus-
sian Naive Bayes is not capable of classifying obsta-
cles. The Random Forest classifier, performed best
with a precision of 70% and a recall of 56% on the
obstacle class. This suggests that 70% of the data
identified as an obstacle was actually an obstacle and
56% of all obstacles were also classified. This is quite
remarkable considering the available data.
The classes drivable and rough produced better
classification rates. This is because solid ground,
which is composed of asphalt or stones, was regarded
as being drivable. And meadows, fields, bushes and
grasses were labeled as rough. So the rough surface
consists almost exclusively of elements, which con-
tain a high proportion of chlorophyll. Elements with
chlorophyll are easily separated from elements with a
low content of chlorophyll, since chlorophyll has its
strongest absorption at about 675 nm and the absorp-
tion decreases sharply afterwards, which can be also
seen in our reconstructed spectrum in figure 4b.
6 CONCLUSION
The experiments carried out imply a Random Forest
classifier to be reliable for hyperspectral classifica-
tion in combination with the snapshot hyperspectral
cameras. The Random Forest classifier delivers de-
cent results for the NIR camera as well as for the VIS
camera. Based on the captured hyperspectral data we
were able to precisely distinguish road or drivable ar-
eas from non-drivable areas like rough or obstacles,
which could greatly enhance terrain classification per-
formance.
Furthermore, a Random Forest can be trained in a
short time in comparison to the other methods. Due
to its structure, it can be parallelized very well and ac-
celerated effectively. Another interesting result is that
the balance of the training is vital for the quality of
the classification. These promising results are a first
showcase for the capabilities of the novel sensor sys-
tem and its suitability for terrain classification, e.g. in
autonomous driving. In order to improve the pixel-
wise classification, we plan to combine it with a con-
ditional random field and to additionally add spatial
and laser data to achieve an improved classification.
ACKNOWLEDGEMENTS
This work was partially funded by Wehrtechnische
Dienststelle 41 (WTD), Koblenz, Germany.
REFERENCES
Camps-Valls, G. and Bruzzone, L. (2005). Kernel-based
methods for hyperspectral image classification. IEEE
Transactions on Geoscience and Remote Sensing,
43(6):1351–1362.
Camps-Valls, G., Gomez-Chova, L., Mu
˜
noz-Mar
´
ı, J., Vila-
Franc
´
es, J., and Calpe-Maravilla, J. (2006). Com-
posite kernels for hyperspectral image classifica-
tion. IEEE Geoscience and Remote Sensing Letters,
3(1):93–97.
Camps-Valls, G., Tuia, D., G
´
omez-Chova, L., Jim
´
enez, S.,
and Malo, J. (2011). Remote sensing image process-
ing. Synthesis Lectures on Image, Video, and Multi-
media Processing, 5(1):1–192.
Cavigelli, L., Bernath, D., Magno, M., and Benini, L.
(2016). Computationally efficient target classifica-
tion in multispectral image data with deep neural net-
works. In SPIE Security+ Defence, pages 99970L–
99970L. International Society for Optics and Photon-
ics.
Chan, T. F., Golub, G. H., and LeVeque, R. J. (1982). Up-
dating formulae and a pairwise algorithm for comput-
ing sample variances. In COMPSTAT 1982 5th Sym-
posium held at Toulouse 1982, pages 30–41. Springer.
Cheriyadat, A. and Bruce, L. M. (2003). Why princi-
pal component analysis is not an appropriate fea-
ture extraction method for hyperspectral data. In
Geoscience and Remote Sensing Symposium, 2003.
IGARSS’03. Proceedings. 2003 IEEE International,
volume 6, pages 3420–3422. IEEE.
Degraux, K., Cambareri, V., Jacques, L., Geelen, B.,
Blanch, C., and Lafruit, G. (2015). Generalized in-
painting method for hyperspectral image acquisition.
In Image Processing (ICIP), 2015 IEEE International
Conference on, pages 315–319. IEEE.
Fauvel, M., Benediktsson, J. A., Chanussot, J., and Sveins-
son, J. R. (2008). Spectral and spatial classification of
hyperspectral data using svms and morphological pro-
files. IEEE Transactions on Geoscience and Remote
Sensing, 46(11):3804–3814.
Geelen, B., Tack, N., and Lambrechts, A. (2014). A
compact snapshot multispectral imager with a mono-
lithically integrated per-pixel filter mosaic. In Spie
Moems-Mems, pages 89740L–89740L. International
Society for Optics and Photonics.
Hughes, G. (1968). On the mean accuracy of statistical pat-
tern recognizers. IEEE transactions on information
theory, 14(1):55–63.
Kriegler, F., Malila, W., Nalepka, R., and Richardson, W.
(1969). Preprocessing transformations and their ef-
fects on multispectral recognition. In Remote Sensing
of Environment, VI, volume 1, page 97.
Hyperspectral Terrain Classification for Ground Vehicles
423