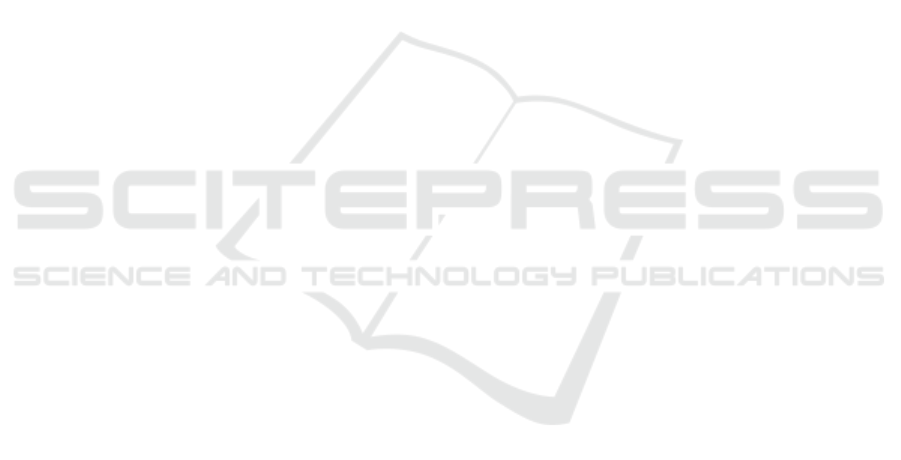
Derrode, S. and Pieczynski, W. (2004). Signal and image
segmentation using pairwise Markov chains. Signal
Processing, IEEE Transactions on, 52(9):2477–2489.
Forney Jr, G. D. (1973). The Viterbi algorithm. Proceedings
of the IEEE, 61(3):268–278.
Foucher, S., Germain, M., Boucher, J.-M., and Benie, G. B.
(2002). Multisource classification using ICM and
Dempster-Shafer theory. IEEE Transactions on In-
strumentation and Measurement, 51(2):277–281.
Fouque, L., Appriou, A., and Pieczynski, W. (2000). An
evidential Markovian model for data fusion and unsu-
pervised image classification. In Information Fusion,
2000. FUSION 2000. Proceedings of the Third Inter-
national Conference on, volume 1, pages TUB4–25.
IEEE.
Gan, L., Wu, Y., Liu, M., Zhang, P., Ji, H., and Wang,
F. (2012). Triplet Markov fields with edge location
for fast unsupervised multi-class segmentation of syn-
thetic aperture radar images. IET image processing,
6(7):831–838.
Habbouchi, A., Boudaren, M. E. Y., A
¨
ıssani, A., and
Pieczynski, W. (2016). Unsupervised segmentation
of markov random fields corrupted by nonstationary
noise. IEEE Signal Processing Letters, 23(11):1607–
1611.
Lanchantin, P., Lapuyade-Lahorgue, J., and Pieczynski,
W. (2011). Unsupervised segmentation of randomly
switching data hidden with non-Gaussian correlated
noise. Signal Processing, 91(2):163–175.
Lanchantin, P. and Pieczynski, W. (2005). Unsupervised
restoration of hidden nonstationary Markov chains us-
ing evidential priors. Signal Processing, IEEE Trans-
actions on, 53(8):3091–3098.
Lapuyade-Lahorgue, J. and Pieczynski, W. (2006). Un-
supervised segmentation of hidden semi-Markov non
stationary chains. In Bayesian Inference and Max-
imum Entropy Methods in Science and Engineer-
ing(AIP Conference Proceedings Volume 872), vol-
ume 872, pages 347–354. Citeseer.
Le H
´
egarat-Mascle, S., Bloch, I., and Vidal-Madjar, D.
(1998). Introduction of neighborhood information in
evidence theory and application to data fusion of radar
and optical images with partial cloud cover. Pattern
recognition, 31(11):1811–1823.
Liu, G., Li, M., Wu, Y., Zhang, P., Jia, L., and Liu, H.
(2014). Polsar image classification based on wishart
TMF with specific auxiliary field. Geoscience and Re-
mote Sensing Letters, IEEE, 11(7):1230–1234.
McLachlan, G. and Krishnan, T. (2007). The EM algorithm
and extensions, volume 382. John Wiley & Sons, New
Jersey.
Park, J., Chebbah, M., Jendoubi, S., and Martin, A. (2014).
Second-order belief hidden markov models. In Belief
Functions: Theory and Applications, pages 284–293.
Springer.
Pieczynski, W. (2003). Pairwise Markov chains. Pat-
tern Analysis and Machine Intelligence, IEEE Trans-
actions on, 25(5):634–639.
Pieczynski, W. (2007). Multisensor triplet Markov chains
and theory of evidence. International Journal of Ap-
proximate Reasoning, 45(1):1–16.
Pieczynski, W. and Benboudjema, D. (2006). Multisensor
triplet Markov fields and theory of evidence. Image
and Vision Computing, 24(1):61–69.
Pieczynski, W., Hulard, C., and Veit, T. (2003). Triplet
Markov chains in hidden signal restoration. In Inter-
national Symposium on Remote Sensing, pages 58–68.
International Society for Optics and Photonics.
Rabiner, L. (1989). A tutorial on hidden Markov models
and selected applications in speech recognition. Pro-
ceedings of the IEEE, 77(2):257–286.
Ramasso, E. (2009). Contribution of belief functions to
hidden Markov models with an application to fault
diagnosis. In Proceedings of the IEEE International
Workshop on Machine Learning for Signal Process-
ing., pages 1–6.
Reineking, T. (2011). Particle filtering in the Dempster–
Shafer theory. International Journal of Approximate
Reasoning, 52(8):1124–1135.
Shafer, G. (1976). A mathematical theory of evidence, vol-
ume 1. Princeton university press Princeton, Prince-
ton.
Soubaras, H. (2010). On evidential Markov chains. In Foun-
dations of reasoning under uncertainty, pages 247–
264. Springer, Berlin Heidelberg.
Tupin, F., Bloch, I., and Ma
ˆ
ıtre, H. (1999). A first step
toward automatic interpretation of SAR images using
evidential fusion of several structure detectors. Geo-
science and Remote Sensing, IEEE Transactions on,
37(3):1327–1343.
Wang, F., Wu, Y., Zhang, Q., Zhang, P., Li, M., and Lu,
Y. (2013). Unsupervised change detection on SAR
images using triplet Markov field model. Geoscience
and Remote Sensing Letters, IEEE, 10(4):697–701.
Wu, Y., Zhang, P., Li, M., Zhang, Q., Wang, F., and
Jia, L. (2013). SAR image multiclass segmentation
using a multiscale and multidirection triplet markov
fields model in nonsubsampled contourlet transform
domain. Information Fusion, 14(4):441–449.
Yoji, Y., Tetsuya, M., Yoji, U., and Yukitaka, S. (2003).
A method for preventing accidents due to human ac-
tion slip utilizing HMM-based Dempster-Shafer the-
ory. In Robotics and Automation, 2003. Proceed-
ings. ICRA’03. IEEE International Conference on,
volume 1, pages 1490–1496. IEEE.
Zhang, P., Li, M., Wu, Y., Gan, L., Liu, M., Wang, F., and
Liu, G. (2012a). Unsupervised multi-class segmenta-
tion of SAR images using fuzzy triplet Markov fields
model. Pattern Recognition, 45(11):4018–4033.
Zhang, P., Li, M., Wu, Y., Liu, M., Wang, F., and
Gan, L. (2012b). SAR image multiclass segmenta-
tion using a multiscale TMF model in wavelet do-
main. Geoscience and Remote Sensing Letters, IEEE,
9(6):1099–1103.
ICEIS 2017 - 19th International Conference on Enterprise Information Systems
414