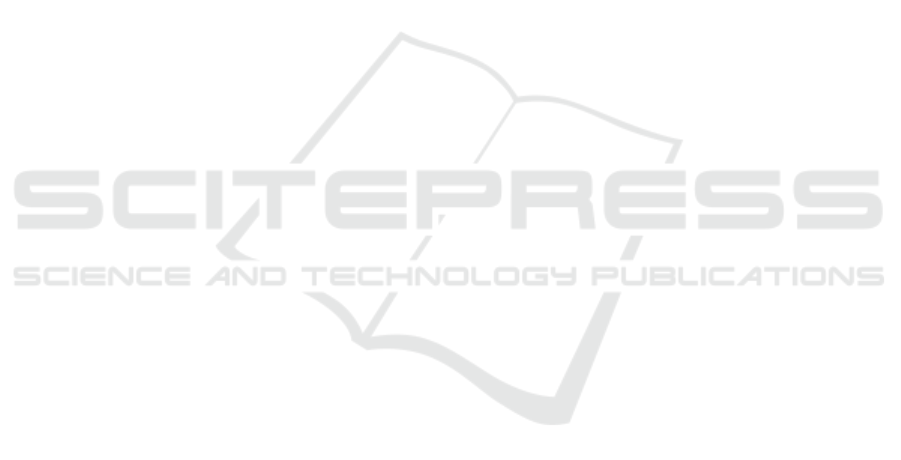
cal and contextual data, simultaneously. It would also
be interesting to evaluate the influence of our tem-
poral based weighting criterion in the supervised ap-
proach to the LP problem. Experiments of our crite-
rion with networks out of the context of co-authorship
would be desirable too. With a larger set of networks,
we also plan to check for statistically significant dif-
ferences among the results obtained by the weighting
criteria.
REFERENCES
Adamic, L. A. and Adar, E. (2003). Friends and neighbors
on the web. Social Networks, 25(3):211 – 230.
Barabasi, A. L., Jeong, H., Neda, Z., and Ravasz, E. (2001).
Evolution of the social network of scientific collabo-
ration. Social Networks, 25:211–230.
Choudhary, P., Mishra, N., Sharma, S., and Patel, R. (2013).
Link score: A novel method for time aware link pre-
diction in social network. In Prasad, D. N. H. and
Nalini, D. N., editors, 2013 Emerging Research in
Computing, Information, Communication and Appli-
cations. Elsevier Science and Technology.
Dunlavy, D. M., Kolda, T. G., and Acar, E. (2011). Tempo-
ral link prediction using matrix and tensor factoriza-
tions. ACM Trans. Knowl. Discov. Data, 5(2):10:1–
10:27.
Granovetter, M. S. (1973). The strength of weak ties. The
American Journal of Sociology.
Hasan, M. and Zaki, M. (2011). A survey of link prediction
in social networks. In Aggarwal, C. C., editor, So-
cial Network Data Analytics, pages 243–275. Springer
US.
Hasan, M. A., Chaoji, V., Salem, S., and Zaki, M. (2006).
Link prediction using supervised learning. In In Proc.
of SDM 06 workshop on Link Analysis, Counterterror-
ism and Security, pages 1–10.
Kuo, T.-T., Yan, R., Huang, Y.-Y., Kung, P.-H., and Lin,
S.-D. (2013). Unsupervised link prediction using ag-
gregative statistics on heterogeneous social networks.
In Proceedings of the 19th ACM SIGKDD Interna-
tional Conference on Knowledge Discovery and Data
Mining, number 19 in KDD ’13, pages 775–783, New
York, NY, USA. ACM. 19/07/2016.
Li, K., Du, N., and Zhang, A. (2012). A link predic-
tion based unsupervised rank aggregation algorithm
for informative gene selection. In Bioinformatics and
Biomedicine (BIBM), 2012 IEEE International Con-
ference on, pages 1–6.
Liben-Nowell, D. and Kleinberg, J. M. (2007). The link-
prediction problem for social networks. JASIST,
58(7):1019–1031.
Lu, L. and Zhou, T. (2010). Link prediction in complex
networks: A survey. CoRR, abs/1010.0725.
L
¨
u, L. and Zhou, T. (2010). Link prediction in weighted
networks: The role of weak ties. EPL (Europhysics
Letters), 89(1):18001.
Munasinghe, L. and Ichise, R. (2012). Time score: A
new feature for link prediction in social networks.
IEICE Transactions on Information and Systems,
E95.D(3):821–828.
Murata, T. and Moriyasu, S. (2007). Link prediction of
social networks based on weighted proximity mea-
sures. In Web Intelligence, IEEE/WIC/ACM Interna-
tional Conference on, pages 85–88.
Soares and Prud
ˆ
encio, R. B. C. (2011). Supervised link
prediction in weighted networks. In Neural Networks
(IJCNN), The 2011 International Joint Conference on,
pages 2281–2288.
Taha, H. (2007). Operations Research: An Introduction.
Pearson/Prentice Hall.
Valverde-Rebaza, J., Valejo, A., Berton, L.,
de Paulo Faleiros, T., and de Andrade Lopes, A.
(2015). A na
¨
Ive bayes model based on overlapping
groups for link prediction in online social networks.
In Proceedings of the 30th Annual ACM Symposium
on Applied Computing, SAC ’15, pages 1136–1141,
New York, NY, USA. ACM.
Wang, P., Xu, B., Wu, Y., and Zhou, X. (2015). Link predic-
tion in social networks: the state-of-the-art. SCIENCE
CHINA Information Sciences, 58(1):1–38.
Zhao, J., Miao, L., Yang, J., Fang, H., Zhang, Q.-M., Nie,
M., Holme, P., and Zhou, T. (2015). Prediction of
links and weights in networks by reliable routes. Sci-
entific reports, 5.
Zhu, B. and Xia, Y. (2016). Link prediction in weighted net-
works: A weighted mutual information model. PLoS
ONE, 11(2):1–13.
Using a Time based Relationship Weighting Criterion to Improve Link Prediction in Social Networks
79