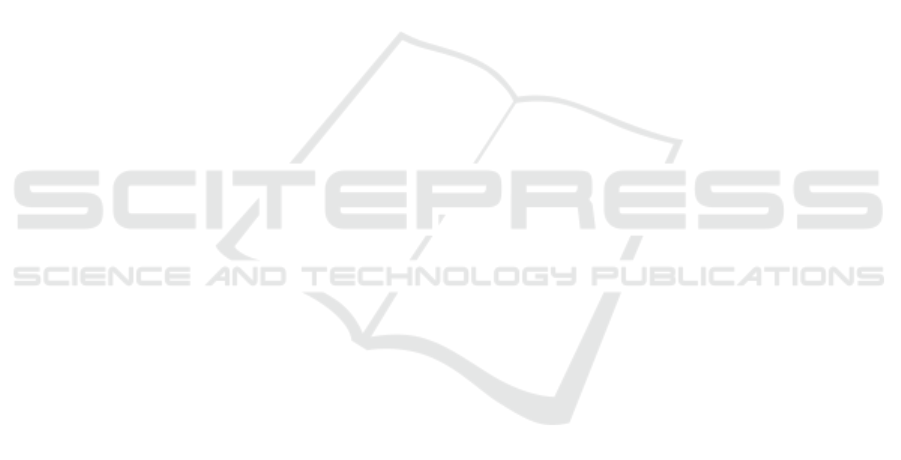
Histogram of Oriented Gradients descriptor. Journal
of Computer and Information Science, 7(3):31–37.
Duda, R., Hart, P., and Stork, D. (2000). Pattern classifica-
tion. Library of Congress Cataloging-in-Publication
Data.
Ekman, P. (1972). Universals and cultural differences in
facial expressions of emotion. University of Nebraska
Press Lincoln, 19.
Happy, S. L. (2015). Automatic Facial Expression
Recognition using Features of Salient Facial Patches.
In IEEE Transactions on Affective Computing, pages
511–518. IEEE.
Huang, X. (2014). Methods for facial expression recogni-
tion with applications in challenging situations. Phd
thesis, University of OULU, INFOTECH OULU.
Kanade, T., Cohn, J., and Tian, Y. (2000). Comprehen-
sive database for facial expression analysis. In Fourth
IEEE International Conference on Automatic Face
and Gesture Recognition, pages 46–53. IEEE.
Khan, R., Meyer, A., Konik, H., and Bouakaz, S. (2013).
Framework for reliable, real-time facial expression
recognition for low resolution images. Journal of Pat-
tern Recognition Letters, 34:1159–1168.
Lin, D. (2006). Facial expression classification using PCA
and Hierarchical Radial Basis Function Network.
Journal of Information Science and Engineering,
22:1033–1046.
Lyons, M., Kamachi, M., and J.Gyoba. The Japanese
Female Facial Expression database (JAFFE).
http://www.kasrl.org/jaffe.html.
Malsburg, C. (1961). Frank Rosenblatt: principles of
Neurodynamics: perceptrons and the theory of brain
mechanisms. Springer Berlin Heidelberg.
Mliki, H., Hammami, M., and Ben-Abdallah, H. (2013).
Mutual information-based facial expression recogni-
tion. In ICMV’13: ixth International Conference on
Machine Vision. Society of Photo-Optical Instrumen-
tation Engineers (SPIE).
Ojala, T., Pietikainen, M., and Harwood, D. (1996). A
comparative study of texture measures with classifica-
tion based on feature distributions. Journal of Pattern
Recognition, 29(1):51–59.
Ojala, T., Pietikainen, M., and Maenpaa, T. (2002). Mul-
tiresolution gray-scale and rotation invariant texture
classification with Local Binary Patterns. IEEE
Transactions on Pattern Analysis and Machine Intel-
ligence, 24(7):971–987.
Ouyang, Y., Sang, N., and Huang, R. (2015). Accurate and
robust facial expressions recognition by fusing multi-
ple sparse representation based classifiers. Journal of
Neurocomputing, 149:71–78.
Priya, G. and Banu, R. (2012). Person independent fa-
cial expression detection using MBWM and multi-
class SVM. International Journal of Computer Ap-
plications, 55(17):52–58.
Saha, A. and Wu, Q. (2010). Facial expression recog-
nition using curvelet based Local Binary Patterns.
In ICASSP’10: International Conference on Acous-
tics Speech and Signal Processing, pages 2470–2473.
IEEE.
S
´
anchez, A., Ruiz, J., Montemayor, A. M. A., Hern
´
andez,
J., and Pantrigo, J. (2011). Differential optical flow ap-
plied to automatic facial expression recognition. Jour-
nal of Neurocomputing, 74(8):1272–1282.
Saragih, J., Lucey, S., and Cohn, J. (2009). Face align-
ment through Subspace Constrained Mean-Shifts. In
ICCV’09: International Conference on Computer Vi-
sion.
Shan, C., Gong, S., and McOwan, P. (2009). A facial ex-
pression recognition based on Local Binary Patterns:
a comprehensive study. Journal of Image and Vision
Computing, 27(6):803–816.
Sirovich, L. and Kirby, M. (1978). Low dimensional proce-
dure for characterization of human faces. Journal of
the Optical Society of America, 4(3):519–524.
Soyel, H. and Demirel, H. (2010). Facial expression recog-
nition based on discriminative Scale Invariant Feature
Transform. IEEE Electronics Letters, 46(5).
S.Zhang, Zhao, X., and Lei, B. (2012). Facial expression
recognition based on Local Binary Patterns and Local
Fisher Discriminant Analysis. WSEAS TRANSAC-
TIONS on SIGNAL PROCESSING, 8:21–31.
Vapnik, V. (1995). The nature of statistical learning theory.
Springer-Verlag New York, Inc. New York, NY, USA.
Viola, P. and Jones, M. (2001). Rapid object detection us-
ing a Boosted Cascade of simple features. In CVPR
2001: International Conference on Computer Vision
and Pattern Recognition, pages 511–518. IEEE.
Visutsak, P. (2013). Emotion classification through lower
facial expressions using Adaptive Support Vector
Machines. Journal of Man, Machine and Technology,
2(1):12–20.
Wallhoff, F. The FG-NET database with facial expres-
sions and emotions. http://cotesys.mmk.e-technik.tu-
muenchen. de/isg/content/feed-database.
Wan, S. and Aggarwal, J. (2014). Spontaneous facial ex-
pression recognition: a robust metric learning ap-
proach. Journal of Pattern Recognition, 47(5):1859–
1868.
Yang, P., Liu, Q., and Metaxas, D. N. (2010). Explor-
ing facial expressions with compositional features.
In CVPR’10: International Conference on Computer
Vision and Pattern Recognition, pages 2638–2644.
IEEE.
Zhang, L., Tjondronegro, D., and V.Chandran (2014).
Facial expression recognition experiments with data
from television broadcasts and the Word Wide Web.
Journal of Image and vision computing, 32:107–119.
ICEIS 2017 - 19th International Conference on Enterprise Information Systems
118