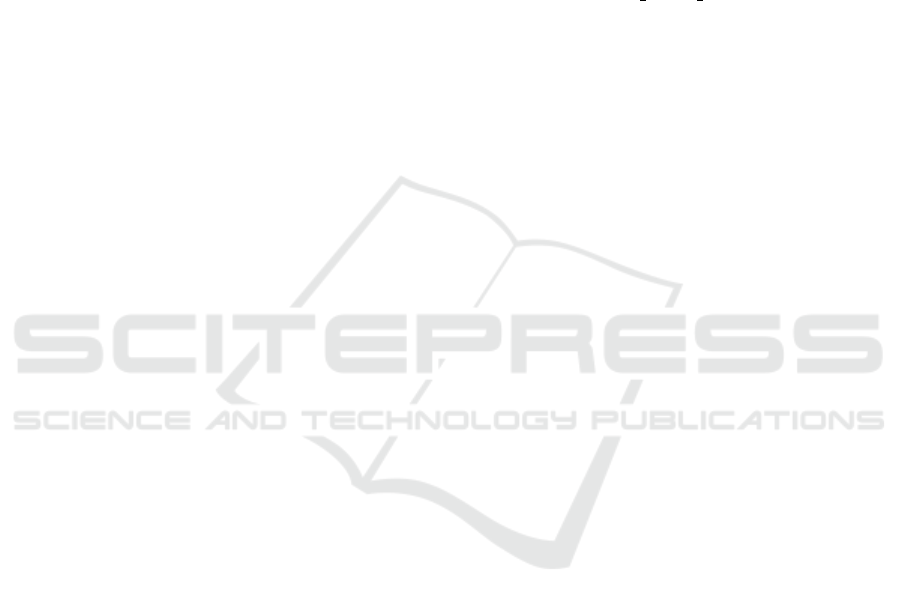
them respectively.
Unfortunately, there is no unique app with the pos-
sibility to have a specific knowledge base for each
subject in order to suggest different recommendations
for the three different groups of subjects.
For this reason, a new mobile health application
is under development in order to take into account
the different results obtained for the different classes
of people examined, e.g. healthy subjects, subjects
with metabolic diseases or subjects with cardiac dis-
eases. The app will be able to monitor body indica-
tors, physiological data, and physical activity infor-
mation by using wearable sensors, be they or not com-
pliant to the Continua Alliance guidelines (Carroll
et al., 2007). Of course, some of these sensors will be
used for long periods, as e.g. those for physical activ-
ity monitoring, therefore they are affected by the typ-
ical problems related to battery charge. Other types
of sensors, instead, will be employed more rarely, as
for example smart digital scales that are typically used
just once in a day.
6 CONCLUSIONS AND FUTURE
WORK
In this paper the correlation between personal and
life-style indicators and the risk of falling has been
investigated.
As indicators we have considered here for each
subject age, body mass index, and information about
physical activity habits, while a subject’s risk of
falling has been estimated by the Mini-BES test
score. Three different groups of subjects have been
taken into account, namely healthy, suffering from
metabolic diseases and suffering from cardiovascular
diseases.
Firstly, explicit linear correlations have been
found for any pair of parameters. Secondly, atten-
tion has been paid to whether or not these correlations
change as the health state of the subjects does.
Finally, some first steps have been moved towards
a system, composed by wearable sensors, a mobile
device, and an app, that would be able to help people
in improving their life-style so as to decrease falling
risk.
In the near future we aim at implementing the
sensor-based system.
Moreover, due to the fact that the data set from
Physionet used in this paper looks highly clustered
with little outliers, e.g. most healthy patients are
around 20 years old, we plan to start a cooperation
phase with the University of Naples ”Federico II” in
which they will provide us with some volunteers with
different ages in order to better balance the database.
Within this cooperation we will supply the system
to the volunteers, so as to test its effectiveness and
usefulness.
ACKNOWLEDGEMENTS
This work has been supported by the project
“eHealthNet: Ecosistema software per la Sanit
`
a Elet-
tronica” (PON03PE 00128 1) financed within the
P.O.N. “Research and Competitiveness” call of the
Italian Ministry for University and Research.
REFERENCES
Carroll, R., Cnossen, R., Schnell, M., and Simons, D.
(2007). Continua: An interoperable personal health-
care ecosystem. IEEE Pervasive Computing, 6(4):90–
94.
Craig, C. L., Marshall, A. L., Sjstrm, M., Bauman, A. E.,
Booth, M. L., Ainsworth, B. E., Pratt, M., Ekelund,
U., Yngve, A., Sallis, J. F., and Oja, P. (2003). In-
ternational physical activity questionnaire: 12-country
reliability and validity. Medicine and science in sports
and exercise, 35(8):13811395.
Faulkner, K. A., Cauley, J. A., Studenski, S. A., Landsittel,
D. P., Cummings, S. R., Ensrud, K. E., Donaldson, M.,
Nevitt, M., of Osteoporotic Fractures Research Group,
S., et al. (2009). Lifestyle predicts falls independent
of physical risk factors. Osteoporosis international,
20(12):2025–2034.
Forastiere, M., De Pietro, G., and Sannino, G. (2016). An
mhealth application for a personalized monitoring of
ones own wellness: Design and development. In Inno-
vation in Medicine and Healthcare 2016, pages 269–
278. Springer.
Franchignoni, F., Horak, F., Godi, M., Nardone, A., and
Giordano, A. (2010). Using psychometric techniques
to improve the balance evaluation systems test: the
mini-bestest. Journal of Rehabilitation Medicine,
42(4):323–331.
Gardner, M. M., Robertson, M. C., and Campbell, A. J.
(2000). Exercise in preventing falls and fall related
injuries in older people: a review of randomised con-
trolled trials. British journal of sports medicine,
34(1):7–17.
Goldberger, A. L., Amaral, L. A., Glass, L., Hausdorff,
J. M., Ivanov, P. C., Mark, R. G., Mietus, J. E., Moody,
G. B., Peng, C.-K., and Stanley, H. E. (2000). Phys-
iobank, physiotoolkit, and physionet components of
a new research resource for complex physiologic sig-
nals. Circulation, 101(23):e215–e220.
Hue, O., Simoneau, M., Marcotte, J., Berrigan, F., Dor
´
e,
J., Marceau, P., Marceau, S., Tremblay, A., and Teas-
dale, N. (2007). Body weight is a strong predictor of
postural stability. Gait & posture, 26(1):32–38.