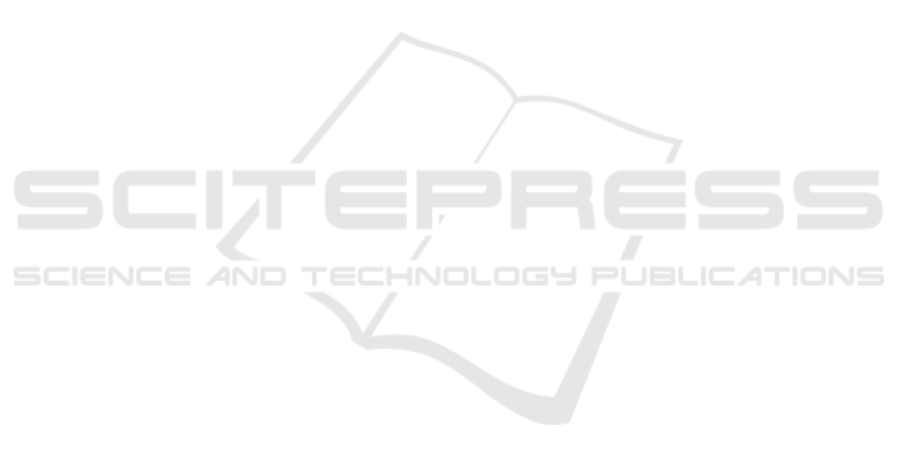
6 CONCLUSION AND OUTLOOK
In this work, we presented a conceptual framework for
identifying resources and capabilities that may be rele-
vant for future executions of business processes. Iden-
tified resources and capabilities are associated with
values representing their degree of relevance to busi-
ness processes. To evaluate our approach, we con-
ducted a survey and compared the collected data in the
survey with the automatically generated results. More-
over, we presented steps for enabling the generation
of recommendations for informal processes and neces-
sary steps involved during the automated generation
of recommended resources and capabilities.
In the future, we will investigate further empirical
evaluation methods for the system presented, such as
the application of recall and precision metrics from
the field of information retrieval. Moreover, we will
develop additional interaction interpreters based on
existing approaches, such as expert finding systems.
We will additionally present the implementation of new
types of resources that are allocated during enactments
of business processes in an ad-hoc fashion based on
emerging requirements of human actors.
ACKNOWLEDGMENTS
This work has been partially supported by Graduate
School of Excellence advanced Manufacturing Engi-
neering (GSaME), DFG Cluster of Excellence in Sim-
ulation Technology (EXC 310/2), and SmartOrchestra
(Research Grant 01MD16001F, BMWi).
REFERENCES
Arias, M. et al. (2016). A framework for recommending
resource allocation based on process mining. In BPM
2015, number 256 in LNBIP. Springer.
Armstrong, J. S. (1978). Long-range Forecasting: From
Crystal Ball to Computer. John Wiley & Sons Inc.
Balog, K., Azzopardi, L., and de Rijke, M. (2006). Formal
models for expert finding in enterprise corpora. In
SIGIR ’06, pages 43–50. ACM.
Begel, A., Khoo, Y. P., and Zimmermann, T. (2010). Code-
book: discovering and exploiting relationships in soft-
ware repositories. In ICSE ’10, volume 1, pages 125–
134. ACM.
Campbell, C. S., Maglio, P. P., Cozzi, A., and Dom, B.
(2003). Expertise identification using email communi-
cations. In CIKM ’03, pages 528–531. ACM.
Di Ciccio, C., Marrella, A., and Russo, A. (2015).
Knowledge-intensive processes: Characteristics, re-
quirements and analysis of contemporary approaches.
JoDS, 4(1):29–57.
Dorn, C. and Dustdar, S. (2011). Supporting dynamic,
people-driven processes through self-learning of mes-
sage flows. In CAiSE 2011, volume 6741 of LNCS,
pages 657–671. Springer.
Dorn, C., Skopik, F., Schall, D., and Dustdar, S. (2011).
Interaction mining and skill-dependent recommenda-
tions for multi-objective team composition. DKE,
70(10):866 – 891.
Dustdar, S. (2004). Caramba—a process-aware collaboration
system supporting ad hoc and collaborative processes
in virtual teams. DPD, 15(1):45–66.
Etzioni, A. (1964). Modern Organizations. Prentice Hall.
Folino, F., Guarascio, M., and Pontieri, L. (2014). Mining
predictive process models out of low-level multidimen-
sional logs. In CAiSE 2014, pages 533–547. Springer.
Folino, F., Guarascio, M., and Pontieri, L. (2015a). Min-
ing multi-variant process models from low-level logs.
In BIS 2015, volume 208 of LNBIP, pages 165–177.
Springer.
Folino, F., Guarascio, M., and Pontieri, L. (2015b). On the
discovery of explainable and accurate behavioral mod-
els for complex lowly-structured business processes.
In ICEIS 2015, pages 206–217. SCITEPRESS.
Herrmann, C. and Kurz, M. (2011). Adaptive case manage-
ment: Supporting knowledge intensive processes with
it systems. In S-BPM ONE 2011, volume 213 of CCIS,
pages 80–97. Springer.
Leymann, F. and Roller, D. (2000). Production Workflow:
Concepts and Techniques. Prentice Hall PTR.
Li, H. and Yamanishi, K. (2003). Topic analysis using a
finite mixture model. IPM, 39(4):521 – 541.
Moody, P., Gruen, D., Muller, M., Tang, J., and Moran, T.
(2006). Business activity patterns: A new model for
collaborative business applications. IBMSJ, 45(4):683–
694.
Schall, D. (2009). Human Interactions in Mixed Systems -
Architecture, Protocols, and Algorithms. PhD thesis,
TU Wien.
Sungur, C., Dorn, C., Dustdar, S., and Leymann, F. (2015a).
Transforming collaboration structures into deployable
informal processes. In ICWE 2015, volume 9114 of
LNCS, pages 231–250. Springer.
Sungur, C. T., Binz, T., Breitenb
¨
ucher, U., and Leymann, F.
(2014). Informal Process Essentials. In EDOC 2014,
pages 200–209. IEEE.
Sungur, C. T., Breitenb
¨
ucher, U., Leymann, F., and Wet-
tinger, J. (2015b). Executing informal processes. In
iiWAS ’15, pages 54:1–54:10. ACM.
Sungur, C. T. et al. (2016). Identifying relevant resources and
relevant capabilities of collaborations - a case study. In
EDOCW 2016, pages 1–4. IEEE.
van der Aalst, W. (2016). Process Mining: Data Science in
Action. Springer.
van der Aalst, W., Pesic, M., and Schonenberg, H. (2009).
Declarative workflows: Balancing between flexibility
and support. CSRD, 23(2):99–113.
van der Aalst, W. and Song, M. (2004). Mining social
networks: Uncovering interaction patterns in business
processes. In BPM 2004, volume 3080 of LNCS, pages
244–260. Springer.
ICEIS 2017 - 19th International Conference on Enterprise Information Systems
306