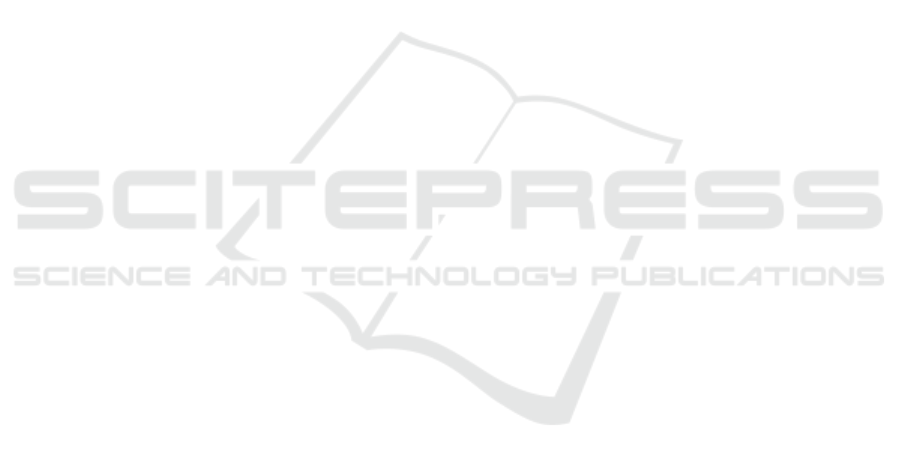
random selections are performed, until forming a set
of normal users that significantly improves (by at least
10%) the accuracy of the recommendations of the sin-
gle GSU, in the limit of 500 trials. Such an improve-
ment is reached for 62% of these GSU. Several at-
tempts have been conducted to characterize the sets
of normal users that improve the recommendations
provided to those GSU. For now, no clear link has
emerged.
To summarize, the SingleGSU model is able to
significantly improve (by more than 10%) the ac-
curacy of the recommendations provided by a stan-
dard MF model, for more than 82% of the selected
GSU. This indicates that it is possible to increase the
accuracy of the recommendations provided to GSU
through a MF technique. The experiments conducted
tend to show that one reason why GSU do not receive
accurate recommendations with standard MF models
may lie in the users used in the factorization process.
5 DISCUSSION AND
CONCLUSION
This work aimed at studying the possibility to de-
sign a MF-based model that improves the accuracy of
the recommendations that standard MF-based models
provide to grey sheep users (GSU).
To reach this goal, we relied on the idea that when
training a model, the ratings of GSU have to be con-
sidered differently than those of normal users. We
proposed three models that either exploit only the rat-
ings of GSU (the GSU Only model), or weight differ-
ently the ratings of GSU and those of normal users
(the WeightedGSU model) or model each GSU inde-
pendently (the SingleGSU model).
The SingleGSU model, that forms a model for
each GSU independently, improves the accuracy of
the recommendations provided to GSU. This model
exploits the ratings of one GSU (the user the model
is dedicated to) as well as those of a subset of normal
users (no rating about other GSU are included). When
normal users are selected according to their similarity
to the GSU, 72% of GSU actually get more accurate
recommendations, which is highly significant. Fur-
thermore, 52% of GSU have an improvement larger
than 10%. This rate even reaches 82% if an additional
way to select normal users is used.
To summarize, we wondered if some MF-models
could improve the accuracy of the recommendations
provided to GSU. Actually, the MF-based SingleGSU
model significantly improves this accuracy, even by
more than 10% for most of GSU.
These results need to be extended to study to what
extent this improvement can be even more improved.
This may rely on a modification of the correlation
measure used to select the normal users to be used in
the SingleGSU model, or designing a completely new
MF technique. In addition, as noticed in section 3,
designing one model per GSU is not practicable, es-
pecially if the number of GSU reaches several thou-
sands of users. So, we would like to study if some
GSU could be grouped together to reduce the number
of models to be developed.
So far, the experiments conducted did not allow to
identify how to select the set of normal users to use,
given one GSU. Some GSU benefit from the selection
of the most similar users, others do not. Being able to
identify which users benefit from these similar users
will also be highly interesting.
REFERENCES
Bellog
´
ın, A., Said, A., and de Vries, A. (2014). The magic
barrier of recommender systems - no magic, just rat-
ings. In Proc. of the 22nd Conf. on User Modelling,
Adaptation and Personalization (UMAP).
Bobadilla, J., Ortega, F., and Hernando, A. (2012). A col-
laborative filtering similarity measure based on singu-
larities. Inf. Process. Manage., 48:204–217.
Castagnos, S., Brun, A., and Boyer, A. (2013). When di-
versity is needed... but not expected! In IMMM 2013,
The Third Int. Conf. on Advances in Information Min-
ing and Management.
Claypool, M., Gokhale, A., and Miranda, T. (1999). Com-
bining content-based and collaborative filters in an on-
line newspaper. In Proceedings of the SIGIR Work-
shop on Recommender Systems: Algorithms and Eval-
uation.
Del Prete, L. and Capra, L. (2010). differs: A mobile rec-
ommender service. In Proc. of the 2010 Eleventh Int.
Conf. on Mobile Data Management, MDM ’10, pages
21–26, Washington, USA. IEEE Computer Society.
Ghazanfar, M. and Prugel-Bennett, A. (2011). Fulfilling the
needs of gray-sheep users in recommender systems, a
clustering solution. In 2011 Int. Conf. on Information
Systems and Computational Intelligence.
Ghazanfar, M. A. and A., P.-B. (2014). Leveraging cluster-
ing approaches to solve the gray-sheep users problem
in recommender systems. Expert Systems with Appli-
cations, 41:3261–3275.
Goldberg, D., Nichols, D., Oki, B., and Terry, D. (1992).
Using collaborative filtering to weave an information
tapestry. Communications of the ACM, 35:61–70.
Gras, B., Brun, A., and Anne, B. (2016). Identifying Grey
Sheep Users in Collaborative Filtering: a Distribution-
Based Technique. In ACM UMAP, page 9, Halifax,
Canada.
Gras, B., Brun, A., and Boyer, A. (2015). Identifying users
with atypical preferences to anticipate inaccurate rec-
ommendations. In Proceedings of the 11th Interna-
Can Matrix Factorization Improve the Accuracy of Recommendations Provided to Grey Sheep Users?
95