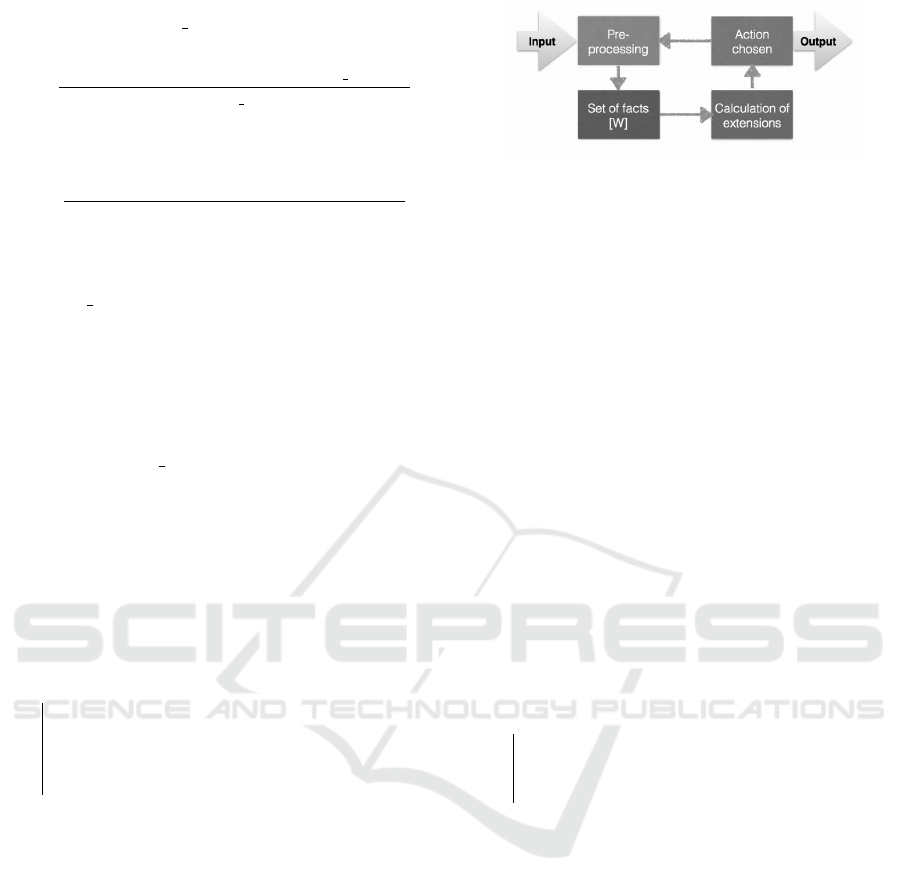
are: E
0
= {glider(srh th,t),motor(o f f ,t),alt(down,t),
var(down,t),batt(good,t)}
d
0
=
alt(down,t) ∧ var(down,t) : glider(srh th,t +1)
glider(srh th,t +1)
(10)
E
1
= {glider(land,t),motor(o f f ,t), alt(down,t),
var(down,t),batt(good,t)}
d
1
=
alt(down,t) ∧ var(down,t) : glider(land,t + 1)
glider(land,t +1)
(11)
We can see that W uses a rule that says
glider(srh th,t + 1) and glider(land,t + 1) are mutu-
ally exclusive. According to this rule, it is not pos-
sible to use d
0
and d
1
at the same time. If d
0
is
used, glider(land,t + 1) is added to the extension,
then mutual exclusive will cancel d
1
. In the same
way, if d
1
is used we cannot use d
0
. We obtain 2
contradictory extensions(solutions). In the first one
there is glider(srh th,t + 1)(The glider can search
a thermal at time t + 1) and the other one there is
glider(land,t + 1)(The glider can land at time t + 1).
In this way, the conflict shown in section 3 is resolved.
Data: E =
/
0 (Set of extensions E is empty)
Result: E = ∪
N
i=0
E
i
Initialization;
CalculExtension(E);
while there is a default (A(X) : C(X))/C(X)
that has not yet been inspected do
Select the default D;
Verify A(X) are true;
Verify C(X) is consistent with W ;
Add C(X) to W ;
end
Backtracking(deleting C(X) added to W );
CalculExtension(E);
Algorithm 1: Calculation of extensions.
In practice, the choose of extension corresponds
a weight to each default considering its importance.
An example could be, to search a thermal is more pri-
ority (more weight) than land(Toulgoat, 2011; Grig-
oris, 1999). These decisions are based on weighted
product model: P(A
K
/A
L
) =
∏
n
j=1
(a
K j
/a
L j
)
w
j
for
K,L = 1, 2, 3, ...,m. Using this method we ponder ex-
tensions then we select the best response.
5 APPLICATION
In this section we present the practical application
and current work. In the Fig. 2 shows the different
parts of our discrete model. On the left side in the
Figure 2: Operating diagram.
Fig. 2, we have the Input such as data, which acces-
sible from electronic devices. Different data are air-
speed, altitude, angles of pitch and roll, etc. Block Set
of facts[W] accepts data for knowledge representa-
tion. Next, Calculation of extensions and Action cho-
sen blocks are the representation of our Algorithm 1.
Finally, we have Output such as an action to apply.
Certainly to the servo-motors to control pitch, roll
and eventually yaw(Fig. 1). These actions will be
applied to a glider(reduced model,Sky Scout version
R2GO). Our glider is equipped with a motor, in case
he needs desperate increase his altitude or find a rising
air. On the other hand, the motor-glider is equipped
with an on-board computer. Prolog has been installed
and sensors have been successfully implemented. The
Algorithm 2 is presented which represents the blocks
of Pre-processing and Set of facts[W], described in
Fig. 2. T
s
is sampling time in seconds.
Data: From electronic device [I]
Result: Set of facts [W ]
Initialization of electronics devices;
while T
s
=1 do
CaptureData(I) from electronics devices;
DescriptionFacts(W) by following our
syntax;
end
Algorithm 2: Knowledge representation.
Data are from the Inertial Measurement
Unit(IMU). An electronics device which collects
angular velocity and linear acceleration data. Usually
a combination of accelerometers and gyroscopes,
sometimes also magnetometers. An IMU works by
detecting changes in rotational attributes like pitch,
roll and yaw, using gyroscopes. On the other hand,
signal processing techniques(Fig. 2) has tremendous
potential in the information fusion theory and in
practical applications. Important part because is the
process of integration of multiple data for knowledge
representation. The acquisition of knowledge is
defined by rules(subsection 4.3). In Fig. 3, rotation
on the X axis is represented, the combination of
accelerometer(circle) and gyroscope(line) data, the
result is a signal(triangle) that we use to describe the
world.
VEHITS 2017 - 3rd International Conference on Vehicle Technology and Intelligent Transport Systems
240