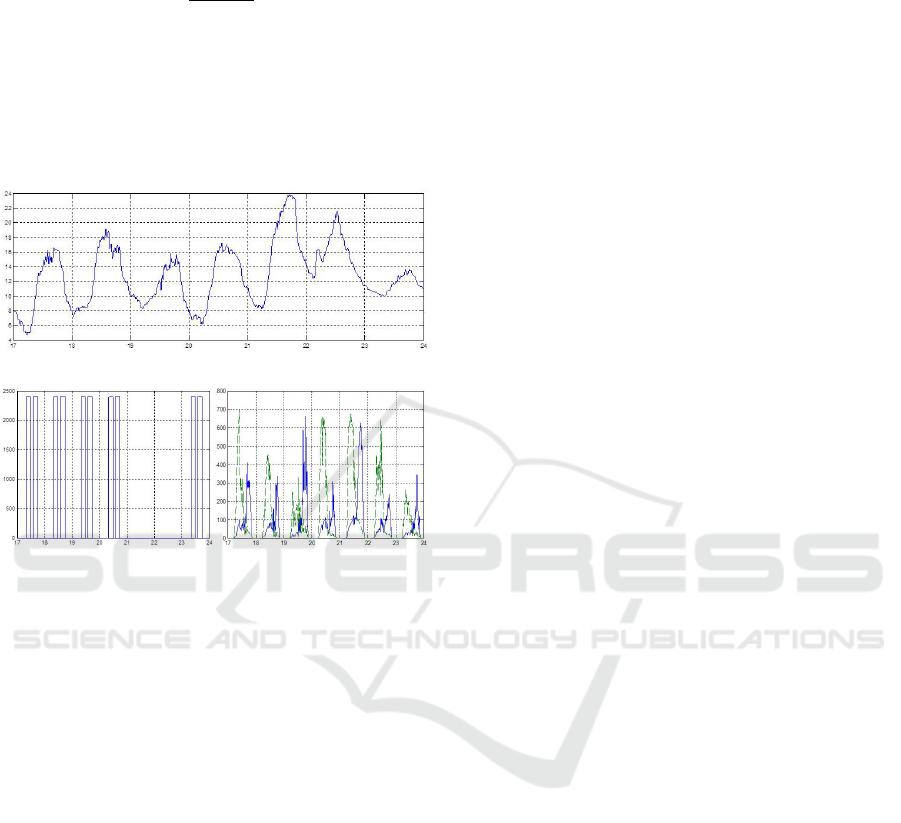
licited (HP in our case) in on-off (hot-cold) control
in order to maintain the temperature of the rooms
1
and
5
close to y
re f
=
y
min
+y
max
2
= 20.5
◦
C. This type
of scenario is highly energy-consuming (see Figure
13c compared to Figure 12c). Similarly, a low predic-
tion horizon N makes the control more pre-emptive,
resulting in a slight increase in the cost of energy in
kW h. N equal to 30 samples (equivalent to 150 min-
utes) seems to be the best adapted in view of the abil-
ity of cooling/warming of the two HP.
Figure 14: Outside temperature (17/05 - 23/05/2016).
Figure 15: (a) Occ
k
, (b) Solar energy (W ) Sol
west
k
(green)
and Sol
east
k
(blue).
5 CONCLUSION
In this paper, a whole simulator of the thermal be-
haviour of a building platform has been established.
In a relatively high order (72), this simulator inte-
grates the behaviours of the set of the partitions wall
dividers/walls/windows of the platform rooms and all
energy sources (solar radiation, temperature, rooms’
occupancy, ventilation (CMV), heat pumps). Based
on a multi model representation of the building and
on an energy cost function in kWh, a predictive con-
trol has been successfully implemented to ensure the
thermal comfort of the platform rooms with a mini-
mum of energy consumption.
ACKNOWLEDGEMENTS
This work was supported by the 7th Framework Pro-
gramme FP7-NMP-608981 ”Energy IN TIME” and
has financial support from the Contrat de Plan Etat-
R
´
egion (CPER) 2015-2020, project ”Mat
´
eriaux, En-
ergie, Proc
´
ed
´
es”.
REFERENCES
Achterbosch, G. G. J., Jong, P. P. G. D., Krist-Spit, C. E.,
Meulen, S. F. V. D., and Verberne, J. (1985). The de-
velopment of a convenient thermal dynamic building
model. Energy and Buildings, 8:183–196.
Agbi, C. (2014). Scalable and Robust Designs of Model -
Based Control Strategies for Energy - Efficient Build-
ings. Dissertations, Carnegie Mellon University -
http://repository.cmu.edu/dissertations/333.
Antonic, O. (1998). Modelling daily topographic solar radi-
ation without site-specific hourly radiation data. Eco-
logical modelling, 113(1-3):31–40.
ASHRAE (2013). ASHRAE Handbook - Fundamentals (SI
Edition). American Society of Heating, Refrigerating
and Air-Conditioning Engineers, Inc.
Atam, E. and Helsen, L. (2016). Control-oriented thermal
modeling of multizone buildings: Methods and issues:
Intelligent control of a building system. IEEE Control
Systems, 36(3):86–111.
Camacho, E. F. and Bordons, C. (2007). Model predictive
control. Advanced textbooks in control and signal pro-
cessing. Springer-Verlag London.
Goyal, S., Ingley, H. A., and Barooah, P. (2013).
Occupancy-based zone-climate control for energy-
efficient buildings: Complexity vs. performance. Ap-
plied Energy, 106:209–221.
Lapusan, C., Balan, R., Hancu, O., and Plesa, A. (2016).
Development of a multi-room building thermody-
namic model using simscape library. Energy Proce-
dia, 85:320–328.
Le, K., Bourdais, R., and Gueguen, H. (2014). Optimal
control of shading system using hybrid model predic-
tive control. In 2014 European Control Conference
(ECC), pages 134–139, Strasbourg, France.
Lofberg, J. (2004). Yalmip: a toolbox for modeling and
optimization in matlab. In 2004 IEEE International
Symposium on Computer Aided Control Systems De-
sign (CACSD), pages 284–289, Taipei, Taiwan.
Ma, Y., Borrelli, F., Hencey, B., Coffey, B., Bengea, S.,
and Haves, P. (2011). Model predictive control for the
operation of building cooling systems. IEEE Transac-
tions on Control Systems Technology, 20(3):796–803.
Oldewurtel, F., Parisio, A., Jones, C. N., Gyalistras, D., Gw-
erder, M., Stauch, V., Lehmann, B., and Morari, M.
(2012). Use of model predictive control and weather
forecasts for energy efficient building climate control.
Energy and Buildings, 45:15–27.
Privara, S., Siroky, J., Ferkl, L., and Cigler, J. (2011). Model
predictive control of a building heating system: The
first experience. Energy and Buildings, 43(2-3):564–
572.
Scherer, H., Pasamontes, M., Guzman, J., Alvarez, J., Cam-
ponogara, E., and Normey-Rico, J. (2014). Effi-
cient building energy management using distributed
model predictive control. Journal of Process Control,
24(6):740–749.
SMARTGREENS 2017 - 6th International Conference on Smart Cities and Green ICT Systems
234