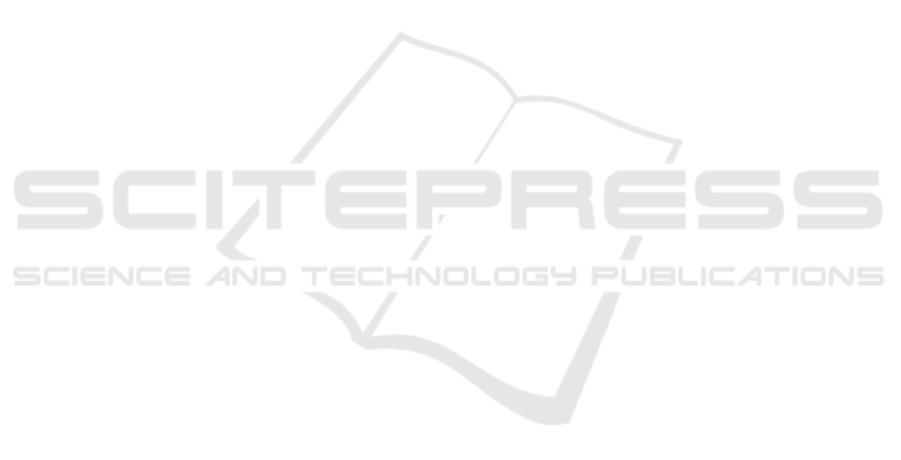
of green energy when not considering the switching
on/off energy costs. It also exhibits good results in
terms of brown energy consumption with a differ-
ence of 4% with the optimal when not considering
the switching on/off energy costs. When consider-
ing the switching on/off energy costs, SAGITTA con-
sumes 10% more brown energy than the theoretical
lower bound, which is the ideal allocation not taking
into account the switching on/off energy costs. We
study the influence of the green energy production on
SAGITTA’s energy gains and show that, in all cases,
it outperforms traditional approaches. The results
also show that SAGITTA can smoothly scale with the
number of data centers belonging to the cloud.
We plan to extend this work by considering the
impact of network devices on the energy consumption
and integrating the ability to dynamically migrate vir-
tual machines from one site to another.
ACKNOWLEDGEMENTS
The authors would like to thank Yunbo Li for the
energy traces of real datacenter servers. The au-
thors would also like to thank Matthieu Simonin and
Nathalie Bertrand for their proofreading of the math-
ematical proofs. This work has been supported by the
Inria exploratory research project COSMIC (Coordi-
nated Optimization of SMart grIds and Clouds).
REFERENCES
Barham, P., Dragovic, B., Fraser, K., Hand, S., Harris, T.,
Ho, A., Neugebauer, R., Pratt, I., and Warfield, A.
(2003). Xen and the Art of Virtualization. In ACM
Symposium on Operating Systems Principles (SOSP),
pages 164–177.
Callau-Zori, M., Samoila, L., Orgerie, A.-C., and Pierre,
G. (2016). An experiment-driven energy consump-
tion model for virtual machine management systems.
Technical Report 8844, Inria.
Camus, B., Galtier, V., Caujolle, M., Chevrier, V.,
Vaubourg, J., Ciarletta, L., and Bourjot, C. (2016a).
Hybrid Co-simulation of FMUs using DEV&DESS
in MECSYCO. In Proceedings of the Symposium on
Theory of Modeling & Simulation - DEVS Integrative
M&S Symposium.
Camus, B., Paris, T., Vaubourg, J., Presse, Y., Bourjot, C.,
Ciarletta, L., and Chevrier, V. (2016b). MECSYCO:
a Multi-agent DEVS Wrapping Platform for the Co-
simulation of Complex Systems. Research report,
LORIA, UMR 7503, Universit
´
e de Lorraine, CNRS,
Vandoeuvre-l
`
es-Nancy ; Inria Nancy - Grand Est
(Villers-l
`
es-Nancy, France).
Deng, W., Liu, F., Jin, H., Li, B., and Li, D. (2014). Har-
nessing renewable energy in cloud datacenters: oppor-
tunities and challenges. IEEE Network, 28(1):48–55.
Fan, X., Weber, W.-D., and Barroso, L. A. (2007). Power
provisioning for a warehouse-sized computer. In ACM
International symposium on Computer architecture
(ISCA), pages 13–23.
Figuerola, S., Lemay, M., Reijs, V., Savoie, M., and St. Ar-
naud, B. (2009). Converged Optical Network Infras-
tructures in Support of Future Internet and Grid Ser-
vices Using IaaS to Reduce GHG Emissions. Journal
of Lightwave Technology, 27(12):1941–1946.
Greenpeace (2011). How dirty is your data? Greenpeace
report.
Katz, R. H. (2009). Tech Titans Building Boom. IEEE
Spectrum, 46(2):40–54.
Koomey, J. (2011). Growth in Data Center Electricity Use
2005 to 2010. Analytics Press.
Li, Y., Orgerie, A.-C., and Menaud, J.-M. (2015). Oppor-
tunistic Scheduling in Clouds Partially Powered by
Green Energy. In IEEE International Conference on
Green Computing and Communications (GreenCom).
Miyoshi, A., Lefurgy, C., Van Hensbergen, E., Rajamony,
R., and Rajkumar, R. (2002). Critical power slope:
understanding the runtime effects of frequency scal-
ing. In ACM International conference on Supercom-
puting (ICS), pages 35–44.
Orgerie, A.-C., Dias de Assunc¸
˜
ao, M., and Lef
`
evre, L.
(2014). A Survey on Techniques for Improving the
Energy Efficiency of Large-Scale Distributed Sys-
tems. ACM Computing Surveys (CSUR), 46(4):47:1–
47:31.
Rais, I., Orgerie, A.-C., and Quinson, M. (2016). Impact
of Shutdown Techniques for Energy-Efficient Cloud
Data Centers. In International Conference on Al-
gorithms and Architectures for Parallel Processing
(ICA3PP), Granada, Spain.
Ren, S., He, Y., and Xu, F. (2012). Provably-Efficient Job
Scheduling for Energy and Fairness in Geographically
Distributed Data Centers. In IEEE International Con-
ference on Distributed Computing Systems (ICDCS),
pages 22–31.
Shehabi, A., Smith, S., Horner, N., Azevedo, I., Brown, R.,
Koomey, J., Masanet, E., Sartor, D., Herrlin, M., and
Lintner, W. (2016). United States Data Center Energy
Usage Report. Technical report, Lawrence Berkeley
National Laboratory.
Snowdon, D., Ruocco, S., and Heiser, G. (2005). Power
Management and Dynamic Voltage Scaling: Myths
and Facts. In Workshop on Power Aware Real-time
Computing.
Talaber, R., Brey, T., and Lamers, L. (2009). Using Virtual-
ization to Improve Data Center Efficiency. Technical
report, The Green Grid.
Tighe, M., Keller, G., Bauer, M., and Lutfiyya, H. (2012).
DCSim: A data centre simulation tool for evaluating
dynamic virtualized resource management. In Work-
shop on Systems Virtualization Management (colo-
cated with CNSM), pages 385–392.
Tripathi, R., Vignesh, S., and Tamarapalli, V. (2016). Op-
timizing Green Energy, Cost, and Availability in Dis-
tributed Data Centers. IEEE Communications Letters.
SMARTGREENS 2017 - 6th International Conference on Smart Cities and Green ICT Systems
58