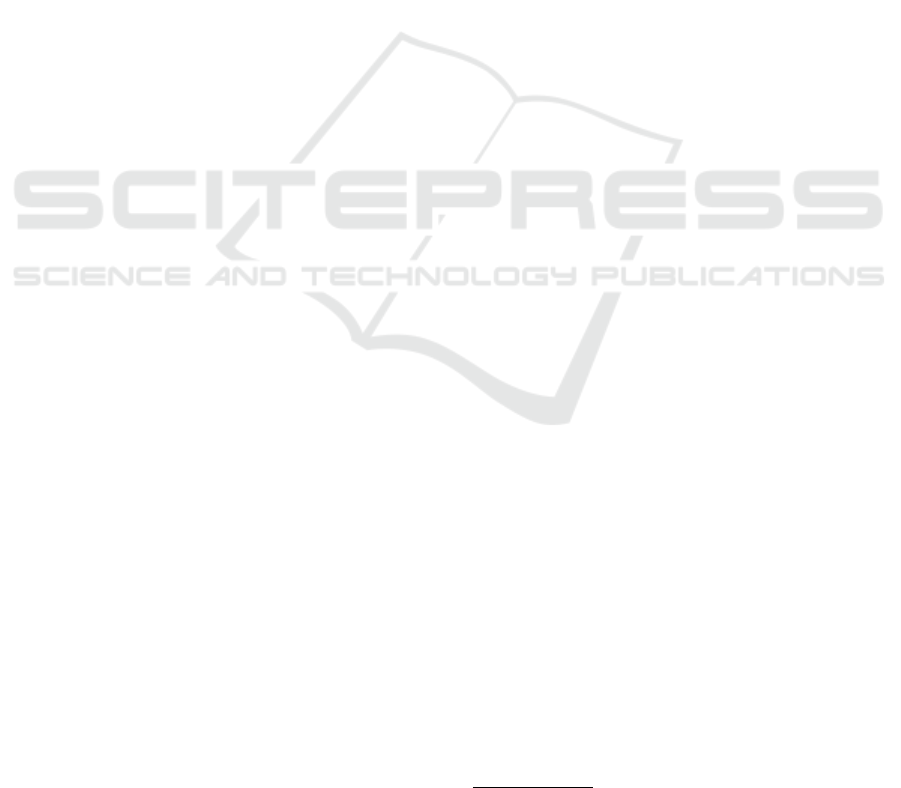
than with DBpedia which taxonomy is a more devel-
oped. DBpedia is very rich in individuals, but a very
flat class taxonomy compared to Wikidata concerning
our Dataset. It also needs to be considered, that be-
cause of ambigious ways column names and values
are defined in the ontology (e.g. abbreviations, short-
ened names etc.) misunderstandings of the human an-
notators about their meanings have been occuring and
could often only partially resolved by extensive web
research on their part.
8 CONCLUSION
In this paper we presented a new approach to inte-
grate geospatial data into the semantic web using a
fully automated way. By focusing on the geome-
try as a new central point for concept detection, we
can build a local ontology structured around this main
concept which allows to gather all information about
it. We encountered that mapping our datasets us-
ing the Wikidata Ontology we can achieve on aver-
age better results than using DBpedia, due to a lack
of a developed class hierarchy in DBPedia. Over-
all the results for our tested datasets seem very close
to at least one of our annotators and we believe that
our results depict one kind of interpretation of the
datasets quite well. However, we as well experi-
enced a lack of detection of several attributes of the
datasets such as double values or meaningful inte-
ger values. In most of the cases such information
is referring to the context of other given columns in
which case with a more sophisticated approach we
can hope to resolve the meaning of the correspond-
ing properties automatically. In more rare cases not
even we as humans can draw a distinctive connec-
tion to the meaning of such columns and therefore we
see little chance for the computer to figure out a so-
lution. We see this research as a basis of discussion
for further automatic integration approaches of het-
erogeneous geospatial data. The approach we have
presented is in our opinion likely to work on many
common geospatial concepts and can therefore lead to
an automated enrichment process for OpenStreetMap
and/or Semantic Web geospatial data in the future.
8.1 Future Work
In our future work we want to explore how we can
better resolve conflicts in our datasets. We therefore
intend to create statistical profiles of typical geome-
tries for the classes we are about to encounter and
therefore create plausability criteria for the relations
between classes representing geometrical objects both
type and possibly area-based. Having discovered typ-
ical relations between typical together occuring ge-
ometry concepts we may as well achieve a more pow-
erful basis for later reasoning experiments. In addi-
tion we may be able to deduce the class of geometries
by their environment once we have been conducting
an in-depth statistical analysis of geometry relations.
This prospect could even be extended to be applied on
moving objects that might be in a dangerzone of some
kind.
ACKNOWLEDGEMENTS
This project was funded by the German Federal Min-
istry of Education and Research
8
.
REFERENCES
Auer, S., Bizer, C., Kobilarov, G., Lehmann, J., Cyganiak,
R., and Ives, Z. (2007). Dbpedia: A nucleus for a web
of open data. Springer.
Bizid, I., Faiz, S., and Boursier, Patriceand Yusuf, J.
C. M. (2014). Advances in Conceptual Modeling:
ER 2013 Workshops, LSAWM, MoBiD, RIGiM, SeC-
oGIS, WISM, DaSeM, SCME, and PhD Symposium,
Hong Kong, China, November 11-13, 2013, Revised
Selected Papers, chapter Integration of Heterogeneous
Spatial Databases for Disaster Management, pages
77–86. Springer International Publishing, Cham.
Cuenca Grau, B. and Jimenez-Ruiz, E. (2011). Logmap:
Logic- based and scalable ontology matching.
Guo, H., Song, G.-f., Ma, L., and WANG, S.-h. (2009).
Design and implementation of address geocoding sys-
tem. Computer Engineering, 35(1):250–251.
Homburg, T., Prudhomme, C., W
¨
urriehausen, F., Karma-
charya, A., Boochs, F., Roxin, A., and Cruz, C.
(2016). Interpreting heterogeneous geospatial data us-
ing semantic web technologies. In International Con-
ference on Computational Science and Its Applica-
tions, pages 240–255. Springer.
Knoblock, C. A., Szekely, P., Ambite, J. L., Goel, A.,
Gupta, S., Lerman, K., Muslea, M., Taheriyan, M.,
and Mallick, P. (2012). Semi-automatically mapping
structured sources into the semantic web. In Extended
Semantic Web Conference, pages 375–390. Springer.
Miller, G. A. (1995). Wordnet: a lexical database for en-
glish. Communications of the ACM, 38(11):39–41.
Navigli, R. and Ponzetto, S. P. (2010). Babelnet: Build-
ing a very large multilingual semantic network. In
Proceedings of the 48th annual meeting of the asso-
ciation for computational linguistics, pages 216–225.
Association for Computational Linguistics.
8
https://www.bmbf.de/en/index.html Project Reference:
03FH032IX4
WEBIST 2017 - 13th International Conference on Web Information Systems and Technologies
114