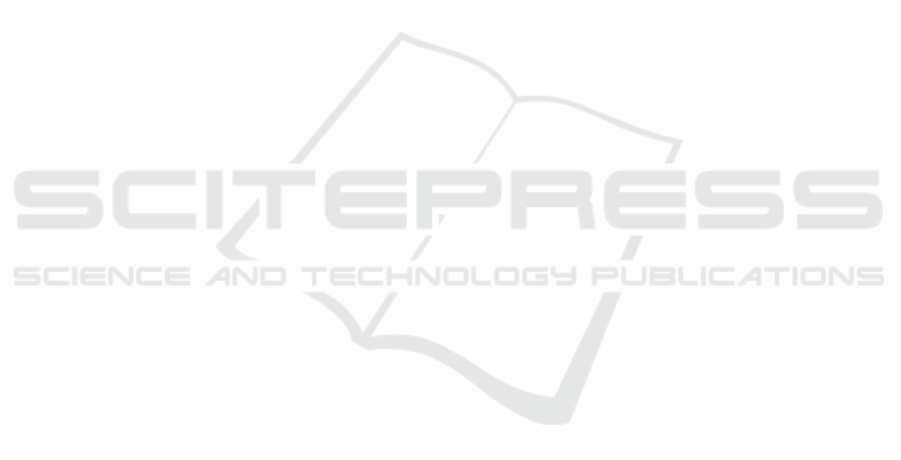
day-ahead forecasting. The resulting system is use-
ful for set point recommendation and load estimation,
where it could forecast the electricity consumed by
ACs given a specfic control input and the weather
conditions during the period of consideration.
The current temperature sensor can only output
integer values. Future work will be to try out other
temperature sensors with higher accuracy and investi-
gate the impact on prediction results. Also, regres-
sion trees pruning techniques could be investigated
as a safeguard against model overfitting during the
training process. The next step is to integrate the sys-
tem into an automated demand response agent, min-
imizing the power consumption of households with
regards to price signals and human comfort.
ACKNOWLEDGEMENT
This work is supported in part by the project funded
by National Research Foundation (NRF) via the
Green Buildings Innovation Cluster (GBIC), adminis-
tered by Building and Construction Authority (BCA),
and in part by the SUTD-MIT International Design
Centre (idc; idc.sutd.edu.sg).
REFERENCES
Afram, A. and Janabi-Sharifi, F. (2015a). Black-box mod-
eling of residential hvac system and comparison of
gray-box and black-box modeling methods. Energy
and Buildings, pages 121–149.
Afram, A. and Janabi-Sharifi, F. (2015b). Gray-box model-
ing and validation of residential hvac system for con-
trol system design. Applied Energy, pages 134–150.
Behl, M., Smarra, F., and Mangharam, R. (2016). Dr-
advisor: A data-driven demand response recom-
mender system. Applied Energy, pages 30–46.
Chan, S.-C., Tsui, K. M., Wu, H., Hou, Y., Wu, Y.-C., and
Wu, F. F. (2012). Load/price forecasting and man-
aging demand response for smart grids: Methodolo-
gies and challenges. IEEE signal processing maga-
zine, pages 68–85.
Ding, Y. M., Hong, S. H., and Li, X. H. (2014). A de-
mand response energy management scheme for indus-
trial facilities in smart grid. IEEE Transactions on In-
dustrial Informatics, pages 2257–2269.
Guyon, I. and Elisseeff, A. (2003). An introduction to vari-
able and feature selection. Journal of machine learn-
ing research, pages 1157–1182.
Horowitz, S., Mauch, B., and Sowell, F. (2014). Forecast-
ing residential air conditioning loads. Applied Energy,
132:47–55.
Jain, M., Singh, A., and Chandan, V. (2016). Non-intrusive
estimation and prediction of residential ac energy con-
sumption. In 2016 IEEE International Conference
on Pervasive Computing and Communications (Per-
Com), pages 1–9. IEEE.
Kalkan, N., Young, E., and Celiktas, A. (2012). Solar ther-
mal air conditioning technology reducing the footprint
of solar thermal air conditioning. Renewable and Sus-
tainable Energy Reviews, pages 6352–6383.
Kavousian, A., Rajagopal, R., and Fischer, M. (2013). De-
terminants of residential electricity consumption: Us-
ing smart meter data to examine the effect of climate,
building characteristics, appliance stock, and occu-
pants’ behavior. Energy, pages 184–194.
Li, J., Poulton, G., Platt, G., Wall, J., and James, G. (2010).
Dynamic zone modelling for hvac system control. In-
ternational Journal of Modelling, Identification and
Control, pages 5–14.
Li, W.-T., Gubba, S. R., Tushar, W., Yuen, C., Hassan,
N. U., Poor, H. V., Wood, K. L., and Wen, C.-K.
(2017). Data driven electricity management for resi-
dential air conditioning systems: An experimental ap-
proach. IEEE Transactions on Emerging Topics in
Computing.
Loh, W.-Y. (2011). Classification and regression trees.
Wiley Interdisciplinary Reviews: Data Mining and
Knowledge Discovery, pages 14–23.
Lork, C., Batchu, R., Yuen, C., and Pindoriya, N. M. (2017).
How many watts: A data driven approach to aggre-
gated residential air-conditioning load forecasting. In
13th Workshop on Context and Activity Modeling and
Recognition (CoMoRea’17), pages 285–290. IEEE.
Lu, N. (2012). An evaluation of the hvac load potential for
providing load balancing service. IEEE Transactions
on Smart Grid, pages 1263–1270.
Qin, X., Lysecky, S., and Sprinkle, J. (2015). A data-driven
linear approximation of hvac utilization for predictive
control and optimization. IEEE Transactions on Con-
trol Systems Technology, pages 778–786.
Shao, S., Shi, W., Li, X., and Chen, H. (2004). Performance
representation of variable-speed compressor for in-
verter air conditioners based on experimental data. In-
ternational journal of refrigeration, pages 805–815.
Tang, F., Kusiak, A., and Wei, X. (2014). Modeling and
short-term prediction of hvac system with a clustering
algorithm. Energy and Buildings, pages 310–321.
Xuan, Z., Qing-dian, L., Guo-qiang, L., Jun-wei, Y., Jian-
cheng, Y., Lie-quan, L., and Wei, H. (2015). Multi-
variable time series forecasting for thermal load of air-
conditioning system on svr. In Control Conference
(CCC), 2015 34th Chinese, pages 8276–8280. IEEE.
Yin, R., Kara, E. C., Li, Y., DeForest, N., Wang, K., Yong,
T., and Stadler, M. (2016). Quantifying flexibility of
commercial and residential loads for demand response
using setpoint changes. Applied Energy, pages 149–
164.
Zhang, P. (1993). Model selection via multifold cross vali-
dation. The Annals of Statistics, pages 299–313.
SMARTGREENS 2017 - 6th International Conference on Smart Cities and Green ICT Systems
76