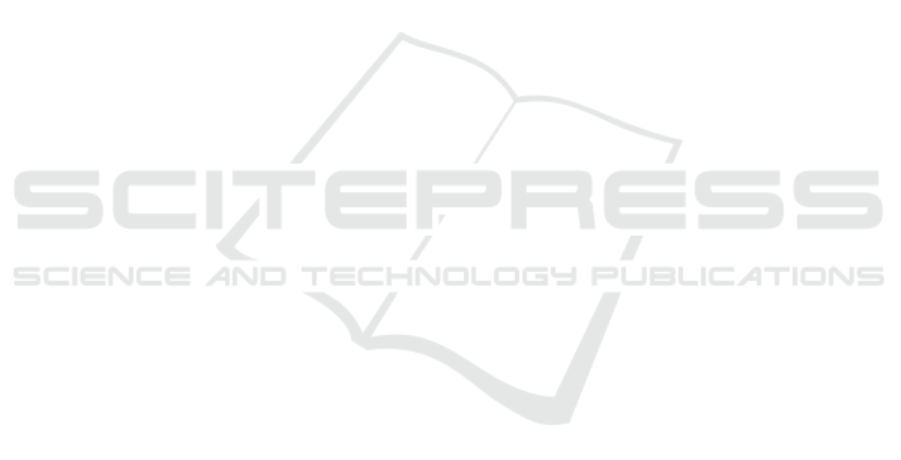
tion retrieval point of view to provide searchable in-
formation acting as a query to retrieve spam accounts.
Our work uses the simple meta-data of a particular set
of users posted tweets associated with a topic (hash-
tag) to predict spammy naming patterns as a search-
able information. Our work can be leveraged by Twit-
ter community to search for spam accounts and also
for Twitter based applications that work on large col-
lection of tweets. As our work is the first in this direc-
tion, we intend to extend the method to predict search-
able information also from tweets as well, with work-
ing on improving the retrieval metrics of the current
method.
REFERENCES
Abascal-Mena, R., Lema, R., and S
`
edes, F. (2014). From
tweet to graph: Social network analysis for seman-
tic information extraction. In IEEE 8th International
Conference on Research Challenges in Information
Science, RCIS 2014, Marrakech, Morocco, May 28-
30, 2014, pages 1–10.
Abascal-Mena, R., Lema, R., and S
`
edes, F. (2015). De-
tecting sociosemantic communities by applying social
network analysis in tweets. Social Network Analysis
and Mining, 5(1):1–17.
Abascal-Mena, R., Lema, R., and S
`
edes, F. (2015). De-
tecting sociosemantic communities by applying social
network analysis in tweets. Social Netw. Analys. Min-
ing, 5(1):38:1–38:17.
Amleshwaram, A. A., Reddy, N., Yadav, S., Gu, G., and
Yang, C. (2013). Cats: Characterizing automation
of twitter spammers. In Communication Systems
and Networks (COMSNETS), 2013 Fifth International
Conference on, pages 1–10. IEEE.
Benevenuto, F., Magno, G., Rodrigues, T., and Almeida, V.
(2010). Detecting spammers on twitter. In In Collab-
oration, Electronic messaging, Anti-Abuse and Spam
Conference (CEAS, page 12.
Cao, C. and Caverlee, J. (2015). Detecting spam urls in
social media via behavioral analysis. In Advances in
Information Retrieval, pages 703–714. Springer.
Chu, Z., Gianvecchio, S., Wang, H., and Jajodia, S. (2012a).
Detecting automation of twitter accounts: Are you a
human, bot, or cyborg? Dependable and Secure Com-
puting, IEEE Transactions on, 9(6):811–824.
Chu, Z., Widjaja, I., and Wang, H. (2012b). Detecting social
spam campaigns on twitter. In Applied Cryptography
and Network Security, pages 455–472. Springer.
Freeman, D. M. (2013). Using naive bayes to detect
spammy names in social networks. In Proceedings
of the 2013 ACM Workshop on Artificial Intelligence
and Security, AISec ’13, pages 3–12, New York, NY,
USA. ACM.
Hu, X., Tang, J., and Liu, H. (2014). Online social spammer
detection. In AAAI, pages 59–65.
Hu, X., Tang, J., Zhang, Y., and Liu, H. (2013). Social
spammer detection in microblogging. In IJCAI, vol-
ume 13, pages 2633–2639. Citeseer.
Lee, K., Caverlee, J., and Webb, S. (2010). Uncovering so-
cial spammers: Social honeypots + machine learning.
In Proceedings of the 33rd International ACM SIGIR
Conference on Research and Development in Informa-
tion Retrieval, SIGIR ’10, pages 435–442, New York,
NY, USA. ACM.
Manning, C. D., Raghavan, P., and Sch
¨
utze, H. (2008). In-
troduction to Information Retrieval. Cambridge Uni-
versity Press, New York, NY, USA.
Martinez-Romo, J. and Araujo, L. (2013). Detecting mali-
cious tweets in trending topics using a statistical anal-
ysis of language. Expert Systems with Applications,
40(8):2992–3000.
McCord, M. and Chuah, M. (2011). Spam detection on
twitter using traditional classifiers. In Proceedings
of the 8th International Conference on Autonomic
and Trusted Computing, ATC’11, pages 175–186.
Springer-Verlag.
Meda, C., Bisio, F., Gastaldo, P., and Zunino, R. (2014). A
machine learning approach for twitter spammers de-
tection. In 2014 International Carnahan Conference
on Security Technology (ICCST), pages 1–6. IEEE.
Mezghani, M., On-at, S., P
´
eninou, A., Canut, M., Zayani,
C. A., Amous, I., and S
`
edes, F. (2015). A case study
on the influence of the user profile enrichment on buzz
propagation in social media: Experiments on deli-
cious. In New Trends in Databases and Information
Systems - ADBIS 2015 Short Papers and Workshops,
BigDap, DCSA, GID, MEBIS, OAIS, SW4CH, WIS-
ARD, Poitiers, France, September 8-11, 2015. Pro-
ceedings, pages 567–577.
Mezghani, M., Zayani, C. A., Amous, I., P
´
eninou, A., and
S
`
edes, F. (2014). Dynamic enrichment of social users’
interests. In IEEE 8th International Conference on Re-
search Challenges in Information Science, RCIS 2014,
Marrakech, Morocco, May 28-30, 2014, pages 1–11.
On-at, S., Quirin, A., P
´
eninou, A., Baptiste-Jessel, N.,
Canut, M., and S
`
edes, F. (2016). Taking into account
the evolution of users social profile: Experiments on
twitter and some learned lessons. In Tenth IEEE Inter-
national Conference on Research Challenges in Infor-
mation Science, RCIS 2016, Grenoble, France, June
1-3, 2016, pages 1–12.
Santos, I., Miambres-Marcos, I., Laorden, C., Galn-
Garca, P., Santamara-Ibirika, A., and Bringas, P. G.
(2014). Twitter content-based spam filtering. In
International Joint Conference SOCO’13-CISIS’13-
ICEUTE’13, pages 449–458. Springer.
Stringhini, G., Kruegel, C., and Vigna, G. (2010). Detecting
spammers on social networks. In Proceedings of the
26th Annual Computer Security Applications Confer-
ence, ACSAC ’10, pages 1–9, New York, NY, USA.
ACM.
Twitter (2016). The twitter rules. https://support.
twitter.com/articles/18311#. [Online; accessed 1-
March-2016].
Wang, A. H. (2010). Don’t follow me: Spam detection
in twitter. In Security and Cryptography (SECRYPT),
Information Quality in Social Networks: Predicting Spammy Naming Patterns for Retrieving Twitter Spam Accounts
621