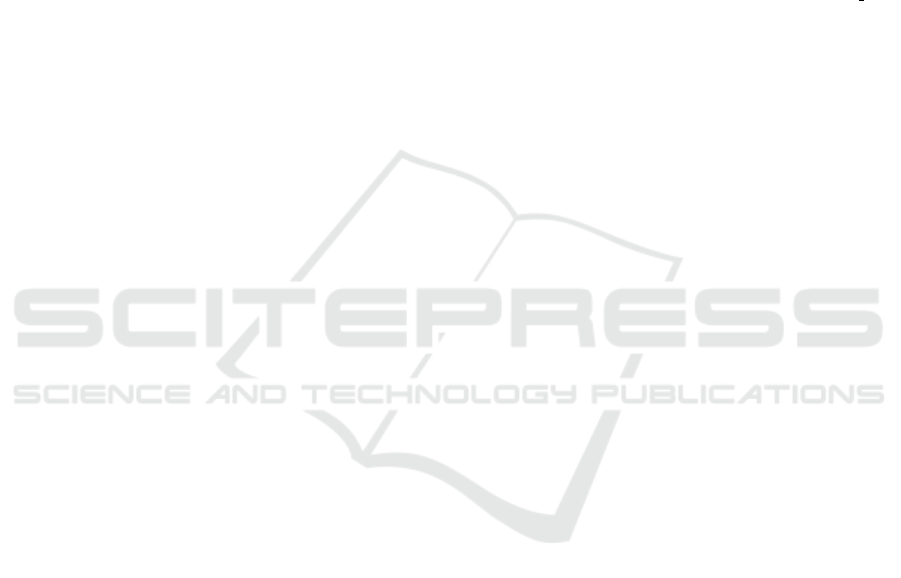
UAI’98, pages 43–52, San Francisco, CA, USA. Mor-
gan Kaufmann Publishers Inc.
Burke, R. (2002). Hybrid Recommender Systems: Sur-
vey and Experiments. User Model User-Adap Inter,
12(4):331–370.
Burke, R. (2007). Hybrid Web Recommender Systems. In
Brusilovsky, P., Kobsa, A., and Nejdl, W., editors, The
Adaptive Web, number 4321 in Lecture Notes in Com-
puter Science, pages 377–408. Springer Berlin Hei-
delberg.
Efron, B. and Tibshirani, R. (1986). Bootstrap Meth-
ods for Standard Errors, Confidence Intervals, and
Other Measures of Statistical Accuracy. Statist. Sci.,
1(1):54–75.
Gantner, Z., Rendle, S., Freudenthaler, C., and Schmidt-
Thieme, L. (2011). MyMediaLite: A Free Recom-
mender System Library. In Proceedings of the Fifth
ACM Conference on Recommender Systems, RecSys
’11, pages 305–308, New York, NY, USA. ACM.
Geng, B., Li, L., Jiao, L., Gong, M., Cai, Q., and Wu,
Y. (2015). NNIA-RS: A multi-objective optimization
based recommender system. Physica A: Statistical
Mechanics and its Applications, 424:383–397.
Goldberg, K., Roeder, T., Gupta, D., and Perkins, C. (2001).
Eigentaste: A Constant Time Collaborative Filtering
Algorithm. Inf. Retr., 4(2):133–151.
Gunawardana, A. and Shani, G. (2009). A Survey of Accu-
racy Evaluation Metrics of Recommendation Tasks. J.
Mach. Learn. Res., 10:2935–2962.
Herlocker, J. L., Konstan, J. A., Borchers, A., and Riedl,
J. (1999). An Algorithmic Framework for Performing
Collaborative Filtering. In Proceedings of the 22Nd
Annual International ACM SIGIR Conference on Re-
search and Development in Information Retrieval, SI-
GIR ’99, pages 230–237, New York, NY, USA. ACM.
Herlocker, J. L., Konstan, J. A., Terveen, L. G., and Riedl,
J. T. (2004). Evaluating Collaborative Filtering Re-
commender Systems. ACM Trans. Inf. Syst., 22(1):5–
53.
Hurley, N. and Rickard, S. (2009). Comparing Measures of
Sparsity. IEEE Trans. Inf. Theor., 55(10):4723–4741.
Jannach, D., Zanker, M., Felfernig, A., and Friedrich, G.
(2010). Recommender Systems: An Introduction.
Cambridge University Press, New York.
L
´
opez-Ib
´
a
˜
nez, M., Dubois-Lacoste, J., P
´
erez C
´
aceres, L.,
Birattari, M., and St
¨
utzle, T. (2016). The irace pack-
age: Iterated racing for automatic algorithm configu-
ration. Operations Research Perspectives, 3:43–58.
McCandless, M., Hatcher, E., and Gospodnetic, O. (2010).
Lucene in Action, Second Edition: Covers Apache
Lucene 3.0. Manning Publications Co., Greenwich,
CT, USA.
McNee, S. M., Riedl, J., and Konstan, J. A. (2006). Being
Accurate is Not Enough: How Accuracy Metrics Have
Hurt Recommender Systems. In CHI ’06 Extended
Abstracts on Human Factors in Computing Systems,
CHI EA ’06, pages 1097–1101, New York, NY, USA.
ACM.
Pedregosa, F., Varoquaux, G., Gramfort, A., Michel, V.,
Thirion, B., Grisel, O., Blondel, M., Louppe, G., Pret-
tenhofer, P., Weiss, R., Dubourg, V., Vanderplas, J.,
Passos, A., Cournapeau, D., Brucher, M., Perrot, M.,
and Duchesnay, E. (2012). Scikit-learn: Machine
Learning in Python. arXiv:1201.0490 [cs]. arXiv:
1201.0490.
Ribeiro, M. T., Ziviani, N., Moura, E. S. D., Hata, I.,
Lacerda, A., and Veloso, A. (2014). Multiobjective
Pareto-Efficient Approaches for Recommender Sys-
tems. ACM Trans. Intell. Syst. Technol., 5(4):53:1–
53:20.
Ricci, F., Rokach, L., and Shapira, B. (2011). Introduc-
tion to Recommender Systems Handbook. In Ricci,
F., Rokach, L., Shapira, B., and Kantor, P. B., edi-
tors, Recommender Systems Handbook, pages 1–35.
Springer US. DOI: 10.1007/978-0-387-85820-3 1.
Sill, J., Takacs, G., Mackey, L., and Lin, D. (2009). Feature-
Weighted Linear Stacking. arXiv:0911.0460 [cs].
arXiv: 0911.0460.
Vargas, S. and Castells, P. (2011). Rank and Relevance in
Novelty and Diversity Metrics for Recommender Sys-
tems. In Proceedings of the Fifth ACM Conference on
Recommender Systems, RecSys ’11, pages 109–116,
New York, NY, USA. ACM.
Wang, S., Gong, M., Li, H., and Yang, J. (2016). Multi-
objective optimization for long tail recommendation.
Knowledge-Based Systems, 104:145–155.
Zhang, M. and Hurley, N. (2008). Avoiding Monotony: Im-
proving the Diversity of Recommendation Lists. In
Proceedings of the 2008 ACM Conference on Recom-
mender Systems, RecSys ’08, pages 123–130, New
York, NY, USA. ACM.
Ziegler, C.-N., McNee, S. M., Konstan, J. A., and
Lausen, G. (2005). Improving Recommendation Lists
Through Topic Diversification. In Proceedings of the
14th International Conference on World Wide Web,
WWW ’05, pages 22–32, New York, NY, USA. ACM.
Zuo, Y., Gong, M., Zeng, J., Ma, L., and Jiao, L. (2015).
Personalized Recommendation Based on Evolution-
ary Multi-Objective Optimization [Research Fron-
tier]. IEEE Computational Intelligence Magazine,
10(1):52–62.
A Multicriteria Evaluation of Hybrid Recommender Systems: On the Usefulness of Input Data Characteristics
633