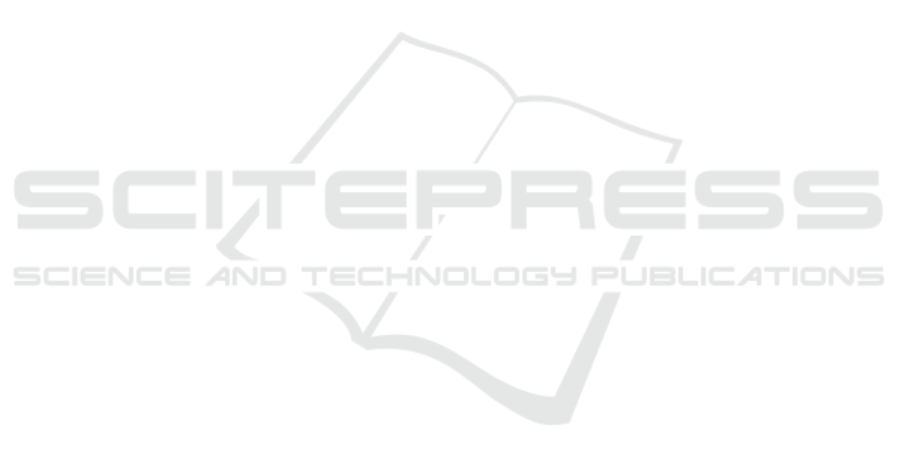
egy for the evaluation of relevant attributes using cri-
teria related to the data and by means of metadata
related to the data sources. Another differential of
our work to the Chen et al. is that we do not need
a training set. The definition of a training set can be
a difficult task, specially in scenarios containing large
volumes of data. Recently, some studies have been
proposed in order to facilitate this task (Bianco et al.,
2015).
7 CONCLUSIONS
In this work, we propose a strategy for selection of
relevant attributes for the Entity Resolution process.
This strategy consists of the following two steps: (i)
Individual Relevance Analysis and (ii) Global Rele-
vance Analysis. In the former, we analyze data fea-
tures, such as repetition and density, to measure the
individual relevance of an attribute. In the later, we
refine results from earlier stages to weight the rele-
vance of each attribute based on quality criteria of the
data sources considered in the Entity Resolution.
For the purposes of evaluating the proposed strat-
egy, we performed several experiments using the
CORA dataset. These experiments have demon-
strated that the groups of attributes selected by our
strategy provide the best result for the Entity Resolu-
tion process, resulting in the validation of our hypoth-
esis. In addition, we have made experiments with the
Febrl dataset obtaining similar results.
As future work, we intend to include other criteria
in the attribute selection process, such as the suscepti-
bility of an attribute to contain errors (e.g. surname),
the attribute dynamism, i.e., if the attribute contains
values that may change over time (e.g. age). We be-
lieve that such characteristics can also be helpful for
the selection of relevant attributes in the Entity Reso-
lution process.
REFERENCES
Bianco, G. D., de Matos Galante, R., Gonalves, M. A.,
Canuto, S. D., and Heuser, C. A. (2015). A practi-
cal and effective sampling selection strategy for large
scale deduplication. IEEE Trans. Knowl. Data Eng.,
27(9):2305–2319.
Canalle, G. K. (2016). Uma estratgia para seleo de atributos
relevantes no processo de resoluo de entidades.
Caruccio, L., Deufemia, V., and Polese, G. (2016). Relaxed
functional dependencies - a survey of approaches.
IEEE Trans. Knowl. Data Eng., 28(1):147–165.
Chen, J., Jin, C., Zhang, R., and Zhou, A. (2012). A learning
method for entity matching. In In Proceedings of 10th
International Workshop on Quality in Databases, East
China Normal University, China.
Christen, P. (2012). Data Matching. Springer, Heidelberg.
Dash, M., Choi, K., Scheuermann, P., and Liu, H. (2002).
Feature selection for clustering - a filter solution. In
ICDM, pages 115–122. IEEE Computer Society.
de Carvalho, M. G., Laender, A. H. F., Goncalves, M. A.,
and da Silva, A. S. (2010). A genetic programming
approach to record deduplication. IEEE Transactions
on Knowledge and Data Engineering, 99(PrePrints).
Dong, X. L. and Srivastava, D. (2015). Big Data Integra-
tion. Synthesis Lectures on Data Management. Mor-
gan & Claypool Publishers.
Draisbach, U. and Naumann, F. (2010). Dude: The dupli-
cate detection toolkit. In In Proceedings of the Inter-
national Workshop on Quality in Databases (QDB).
Fan, W., Jia, X., Li, J., and Ma, S. (2009). Reasoning about
record matching rules. PVLDB, 2(1):407–418.
Gruenheid, A., Dong, X. L., and Srivastava, D. (2014). In-
cremental record linkage. PVLDB, 7(9):697–708.
Gu, L., Baxter, R., Vickers, D., and Rainsford, C. (2003).
Record linkage: Current practice and future direc-
tions. Technical report, CSIRO Mathematical and In-
formation Sciences.
Jouve, P.-E. and Nicoloyannis, N. (2005). A filter feature se-
lection method for clustering. In Hacid, M.-S., Mur-
ray, N. V., Ras, Z. W., and Tsumoto, S., editors, IS-
MIS, volume 3488 of Lecture Notes in Computer Sci-
ence, pages 583–593. Springer.
Kopcke, H. and Rahm, E. (2010). Frameworks for en-
tity matching: A comparison. Data Knowl. Eng.,
69(2):197–210.
Li, Y., Lu, B.-L., and Wu, Z.-F. (2006). A hybrid method
of unsupervised feature selection based on ranking. In
ICPR (2), pages 687–690. IEEE Computer Society.
Mihaila, G. A., Raschid, L., and Vidal, M.-E. (2000). Using
quality of data metadata for source selection and rank-
ing. In WebDB (Informal Proceedings), pages 93–98.
Oliveira, M. I. d. S., Lscio, B., and Gama, K. (2015). An-
lise de desempenho de catlogo de produtores de dados
para internet das coisas baseado em sensorml e nosql.
XIV Workshop em Desempenho de Sistemas Computa-
cionais e de Comunicao.
Sarawagi, S. and Bhamidipaty, A. (2002). Interactive dedu-
plication using active learning. In KDD ’02: Proceed-
ings of the eighth ACM SIGKDD international confer-
ence on Knowledge discovery and data mining, pages
269–278, New York, NY, USA. ACM.
Su, W., Wang, J., Lochovsky, F. H., and Society, I. C.
(2010). Record Matching over Query Results from
Multiple Web Databases. IEEE Transactions on
Knowledge and Data Engineering, 22(4):578–589.
Wang, R. Y. and Strong, D. M. (1996). Beyond accuracy:
What data quality means to data consumers. J. Man-
age. Inf. Syst., 12(4):5–33.
ICEIS 2017 - 19th International Conference on Enterprise Information Systems
88