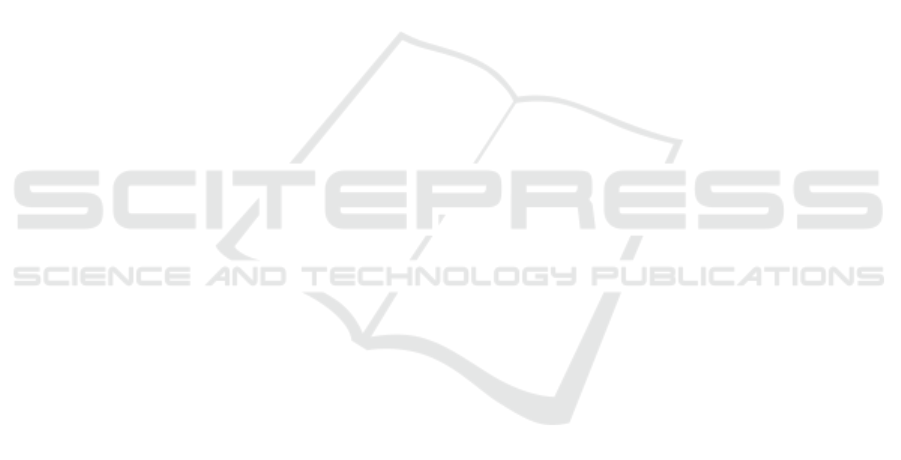
e Ricardo Araujo e Douglas Detoni, C. C. (2015). Mo-
delagem e predic¸
˜
ao de reprovac¸
˜
ao de acad
ˆ
emicos de
cursos de educac¸
˜
ao a dist
ˆ
ancia a partir da contagem
de interac¸
˜
oes. Revista Brasileira de Inform
´
atica na
Educac¸
˜
ao, 23(03):1.
EDUCAUSE Center for Analysis and Research (2014). The
Current Ecosystem of Learning Management Systems
in Higher Education: Student, Faculty, and IT Per-
spectives. Accessado em 27/07/2016.
Garc
´
ıa-Saiz, D. and Zorrilla, M. (2012). A promising classi-
fication method for predicting distance students’ per-
formance. In Educational Data Mining 2012.
Gu
´
ercio, H., P., M., V., S., P., K., and E., B. (2014). An
´
alise
do desempenho estudantil na educac¸
˜
ao a dist
ˆ
ancia ap-
licando t
´
ecnicas de minerac¸
˜
ao de dados. Workshop de
Minerac¸
˜
ao de Dados em Ambientes Virtuais do En-
sino e Aprendizagem, 3.
Hall, M., Frank, E., Holmes, G., Pfahringer, B., Reute-
mann, P., and Witten, I. H. (2009). The weka data
mining software: An update. SIGKDD Explor. Newsl.,
11(1):10–18.
Jindal, R. and Borah, M. D. (2013). A survey on educa-
tional data mining and research trends. International
Journal of Database Management Systems, 5(3):53.
Kotsiantis, S., Patriarcheas, K., and Xenos, M. (2010).
A combinational incremental ensemble of classifiers
as a technique for predicting students’ performance
in distance education. Knowledge-Based Systems,
23(6):529 – 535.
Luan, J. (2002). Data mining and its applications in higher
education. New Directions for Institutional Research,
2002(113):17–36.
Marquez-Vera, C., Morales, C. R., and Soto, S. V. (2013).
Predicting school failure and dropout by using data
mining techniques. IEEE Revista Iberoamericana de
Tecnologias del Aprendizaje, 8(1):7–14.
Moodle.org (2016a). Moodle Philosophy.
https://docs.moodle.org/24/en/Philosophy. Accessed:
2016-10-05.
Moodle.org (2016b). Moodle Statistics.
https://moodle.net/stats/. Accessed: 2016-10-05.
Moore, M. G. (1989). Editorial: Three types of interaction.
American Journal of Distance Education, 3(2):1–7.
Moradi, H., Moradi, S. A., and Kashani, L. (2014). Stu-
dents’ Performance Prediction Using Multi-Channel
Decision Fusion, pages 151–174. Springer Internati-
onal Publishing, Cham.
Neto, F. A. A. and Castro, A. (2015). Elicited and mined
rules for dropout prevention in online courses. In 2015
IEEE Frontiers in Education Conference (FIE), pages
1–7.
Olama, M. M., Thakur, G., McNair, A. W., and Sukumar,
S. R. (2014). Predicting student success using ana-
lytics in course learning management systems. Proc.
SPIE, 9122:91220M–91220M–9.
Pe
˜
na-Ayala, A. (2014). Educational data mining: A survey
and a data mining-based analysis of recent works. Ex-
pert Systems with Applications, 41(4, Part 1):1432 –
1462.
Pierrakeas, C., Xeno, M., Panagiotakopoulos, C., and Ver-
gidis, D. (2004). A comparative study of dropout rates
and causes for two different distance education cour-
ses. The International Review of Research in Open
and Distributed Learning, 5(2).
Romero, C., L
´
opez, M.-I., Luna, J.-M., and Ventura, S.
(2013). Predicting students’ final performance from
participation in on-line discussion forums. Computers
& Education, 68:458 – 472.
Romero, C. and Ventura, S. (2007). Educational data mi-
ning: A survey from 1995 to 2005. Expert Systems
with Applications, 33(1):135 – 146.
Romero, C. and Ventura, S. (2010). Educational data mi-
ning: A review of the state of the art. IEEE Tran-
sactions on Systems, Man, and Cybernetics, Part C
(Applications and Reviews), 40(6):601–618.
Romero, C., Ventura, S., Espejo, P. G., and Herv
´
as, C.
(2008a). Data mining algorithms to classify students.
In Educational Data Mining 2008.
Romero, C., Ventura, S., and Garc
´
ıa, E. (2008b). Data
mining in course management systems: Moodle case
study and tutorial. Computers & Education, 51(1):368
– 384.
Sharma, M. and Mavani, M. (2011). Accuracy comparison
of predictive algorithms of data mining: Application
in education sector. Communications in Computer and
Information Science, 125 CCIS:189–194. cited By 0.
Sisovic, S., Matetic, M., and Bakaric, M. B. (2016). Cluste-
ring of imbalanced moodle data for early alert of stu-
dent failure. In 2016 IEEE 14th International Sympo-
sium on Applied Machine Intelligence and Informatics
(SAMI), pages 165–170.
Sorour, S. E., Goda, K., and Mine, T. (2015). Estimation of
student performance by considering consecutive les-
sons. In 2015 IIAI 4th International Congress on Ad-
vanced Applied Informatics, pages 121–126.
Thakar, P. (2015). Performance analysis and prediction
in educational data mining: A research travelogue.
CoRR, abs/1509.05176.
Yoshida, T., Kou, G., Skowron, A., Cao, J., Hacid, H., and
Zhong, N. (2013). Detection and Presentation of Fai-
lure of Learning from Quiz Responses in Course Ma-
nagement Systems, pages 64–73. Springer Internatio-
nal Publishing, Cham.
Zacharis, N. Z. (2015). A multivariate approach to pre-
dicting student outcomes in web-enabled blended le-
arning courses. The Internet and Higher Education,
27:44 – 53.
Zorrilla, M. and Garcia-Saiz, D. (2014). Meta-learning:
Can it be suitable to automatise the kdd process for
the educational domain? Lecture Notes in Computer
Science (including subseries Lecture Notes in Artifi-
cial Intelligence and Lecture Notes in Bioinformatics),
8537 LNAI:285–292. cited By 0.
CSEDU 2017 - 9th International Conference on Computer Supported Education
346