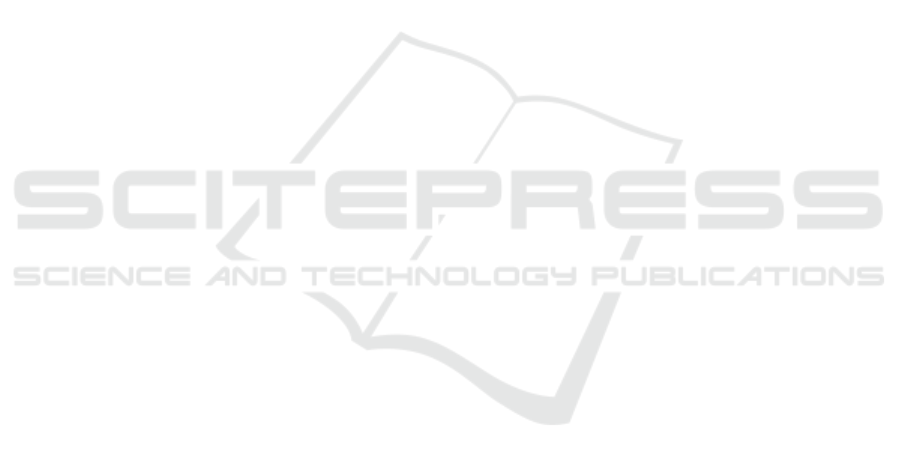
by our process. Our interpretation should be seen as a
possible extension of other Visual Analytics pipelines
and not as a replacement, because this approach offers
the most extensive user integration we could find dur-
ing extensive literature research. As a consequence,
this approach depends crucially on the user and the
associated hazards like biased view or background
knowledge and therefore the users’ compelling influ-
ence on the results. Furthermore, the cooperation be-
tween domain experts determining the analysis pro-
cess themselves and the excluded IT department is not
expected to be straightforward.
In our future work, we will investigate the differ-
ent steps based on our process in an overarching ar-
chitecture as well as different concepts to reduce the
conflict potential between domain experts and IT de-
partments.
REFERENCES
Alpar, P. and Schulz, M. (2016). Self-Service Business In-
telligence. Business & Information Systems Engineer-
ing, 58(2):151–155.
Bertini, E. and Lalanne, D. (2009). Surveying the com-
plementary role of automatic data analysis and visu-
alization in knowledge discovery. In Proceedings of
the ACM SIGKDD Workshop on Visual Analytics and
Knowledge Discovery: Integrating Automated Anal-
ysis with Interactive Exploration, pages 12–20, New
York, USA. ACM Press.
B
¨
ogl, M., Aigner, W., Filzmoser, P., Lammarsch, T.,
Miksch, S., and Rind, A. (2013). Visual Analytics
for Model Selection in Time Series Analysis. IEEE
Transactions on Visualization and Computer Graph-
ics, 19(12):2237–2246.
Card, S. K., Mackinlay, J. D., and Shneiderman, B. (1999).
Information Visualization. In Card, S. K., Mackinlay,
J. D., and Shneiderman, B., editors, Readings In Infor-
mation Visualization: Using Vision To Think, pages 1–
34. Morgan Kaufmann Publishers Inc., San Francisco,
CA, USA.
Chiticariu, L., Kolaitis, P. G., and Popa, L. (2008). Interac-
tive generation of integrated schemas. In Proceedings
of the 2008 ACM SIGMOD International Conference
on Management of Data, pages 833–846. ACM.
Cypher, A., editor (1993). Watch What I Do – Programming
by Demonstration. MIT Press, Cambridge, MA, USA.
Daniel, F. and Matera, M. (2014). Mashups. Concepts,
Models and Architectures. Springer, Berlin, Heidel-
berg.
Eckerson, W. W. (2009). Self-Service BI. Checklist Report,
TDWI Research.
EMC Corporation (2014). Digital Universe Invaded By
Sensors. Press Release.
Endert, A., Hossain, M. S., Ramakrishnan, N., North, C.,
Fiaux, P., and Andrews, C. (2014). The human is the
loop: new directions for visual analytics. Journal of
Intelligent Information Systems, 43(3):411–435.
Fayyad, U., Piatetsky-Shapiro, G., and Smyth, P. (1996).
The KDD Process for Extracting Useful Knowledge
from Volumes of Data. Communications of the ACM,
39(11):27–34.
Gantz, J. and Reinsel, D. (2012). THE DIGITAL UNI-
VERSE IN 2020: Big Data, Bigger Digital Shadows,
and Biggest Growth in the Far East. International Data
Corporation (IDC).
Hirmer, P. and Behringer, M. (2017). FlexMash 2.0 – Flex-
ible Modeling and Execution of Data Mashups. In
Daniel, F. and Gaedke, M., editors, Rapid Mashup De-
velopment Tools, pages 10–29. Springer International
Publishing, Cham.
Hirmer, P. and Mitschang, B. (2016). FlexMash – Flex-
ible Data Mashups Based on Pattern-Based Model
Transformation. In Daniel, F. and Pautasso, C., edi-
tors, Rapid Mashup Development Tools, pages 12–30.
Springer International Publishing, Cham.
Hirmer, P., Reimann, P., Wieland, M., and Mitschang, B.
(2015). Extended Techniques for Flexible Model-
ing and Execution of Data Mashups. In Helfert, M.,
Holzinger, A., Belo, O., and Francalanci, C., editors,
Proceedings of 4th International Conference on Data
Management Technologies and Applications, pages
111–122. SciTePress.
Imhoff, C. and White, C. (2011). Self-Service Business In-
telligence. Best Practices Report, TDWI Research.
Kandel, S., Heer, J., Plaisant, C., Kennedy, J., van Ham,
F., Riche, N. H., Weaver, C., Lee, B., Brodbeck, D.,
and Buono, P. (2011a). Research directions in data
wrangling: Visualizations and transformations for us-
able and credible data. Information Visualization,
10(4):271–288.
Kandel, S., Paepcke, A., Hellerstein, J., and Heer, J.
(2011b). Wrangler: Interactive Visual Specification
of Data Transformation Scripts. In Proceedings of the
SIGCHI Conference on Human Factors in Comput-
ing Systems, pages 3363–3372. ACM, New York, NY,
USA.
Keim, D. A., Andrienko, G., Fekete, J.-D., G
¨
org, C.,
Kohlhammer, J., and Melanc¸on, G. (2008). Visual
Analytics: Definition, Process, and Challenges. In
Kerren, A., Stasko, J. T., Fekete, J.-D., and North,
C., editors, Information Visualization, pages 154–175.
Springer, Berlin, Heidelberg.
Keim, D. A., Kohlhammer, J., Mansmann, F., May, T., and
Wanner, F. (2010). Visual Analytics. In Keim, D.,
Kohlhammer, J., Ellis, G., and Mansmann, F., editors,
Mastering The Information Age, pages 7–18. Euro-
graphics Association, Goslar.
Keim, D. A., Mansmann, F., Schneidewind, J., and Ziegler,
H. (2006). Challenges in Visual Data Analysis. In
Proceedings of the International Conference on Infor-
mation Visualisation, pages 9–16. IEEE.
Kemper, H.-G., Baars, H., and Mehanna, W. (2010). Busi-
ness Intelligence – Grundlagen und praktische An-
wendungen. Eine Einf
¨
uhrung in die IT-basierte Man-
agementunterst
¨
utzung. Vieweg+Teubner, Wiesbaden.
Towards Interactive Data Processing and Analytics - Putting the Human in the Center of the Loop
95