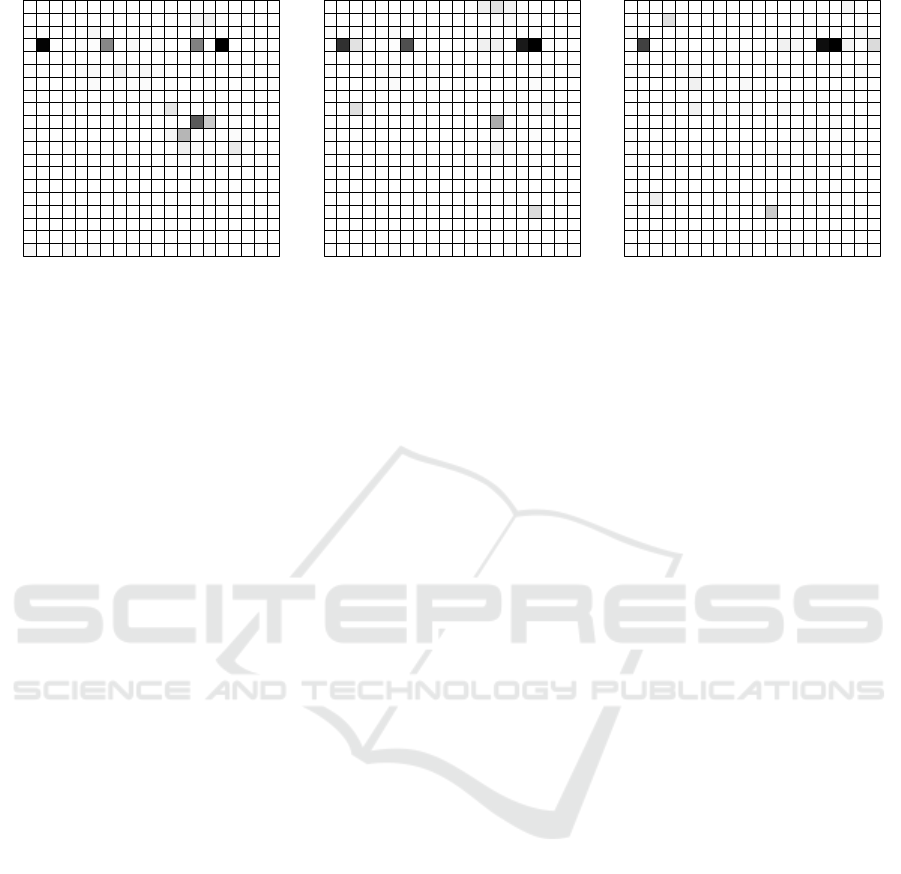
(a) (b) (c)
Figure 5: The reconstruction error of the liquidity matrices at (a) t
8528
, (b) t
8529
, and (c) t
8530
of bank run C estimated by the
linear autoencoder. The intensity of each element indicates the error made by the autoencoder for the corresponding liquidity
flow. The fourth row from the top represents the outgoing liquidity flows of the stressed bank.
REFERENCES
Allen, F. and Gale, D. (2000). Financial Contagion. Journal
of Political Economy, 108:1–33.
Arciero, L., Heijmans, R., Heuver, R., Massarenti, M., Pi-
cillo, C., and Vacirca, F. (2016). How to Measure the
Unsecured Money Market? The Eurosystem’s Imple-
mentation and Validation using TARGET2 Data. In-
ternational Journal of Central Banking, March:247–
280.
Armantier, O. and Copeland, A. (2012). Assessing the
Quality of Furfine-based Algorithms. Federal Reserve
Bank of New York Staff Report, 575.
Berndsen, R. J., Le
´
on, C., and Renneboog, L. (2016). Fi-
nancial Stability in Networks of Financial Institutions
and Market Infrastructures. Journal of Financial Sta-
bility.
Bottou, L. (2004). Stochastic Learning. In Advanced lec-
tures on machine learning, pages 146–168. Springer.
Chandola, V., Banerjee, A., and Kumar, V. (2009).
Anomaly Detection: A Survey. ACM Comput. Surv.,
41(3):15:1–15:58.
Donoho, S. (2004). Early Detection of Insider Trading in
Option Markets. In Proceedings of the tenth ACM
SIGKDD international conference on Knowledge dis-
covery and data mining, pages 420–429. ACM.
Ferdousi, Z. and Maeda, A. (2006). Unsupervised Out-
lier Detection in Time Series Data. In 22nd Inter-
national Conference on Data Engineering Workshops
(ICDEW’06), pages 51–56. IEEE.
Furfine, C. (1999). The Microstructure of the Federal Funds
Market. Financial Markets, Institutions and Instru-
ments, 8:24–44.
Gai, P. and Kapadia, S. (2010). Contagion in financial net-
works. In Proceedings of the Royal Society of London
A: Mathematical, Physical and Engineering Sciences,
page rspa20090410. The Royal Society.
Ghosh, S. and Reilly, D. L. (1994). Credit card fraud de-
tection with a neural-network. In System Sciences,
1994. Proceedings of the Twenty-Seventh Hawaii In-
ternational Conference on, volume 3, pages 621–630.
IEEE.
Hawkins, S., He, H., Williams, G., and Baxter, R. (2002).
Outlier Detection Using Replicator Neural Networks.
In International Conference on Data Warehousing
and Knowledge Discovery, pages 170–180. Springer.
Heijmans, R. and Heuver, R. (2014). Is this Bank Ill? The
Diagnosis of Doctor TARGET2. Journal of Financial
Market Infrastructures, 2(3).
Kim, Y. and Sohn, S. Y. (2012). Stock fraud detection us-
ing peer group analysis. Expert Systems with Applica-
tions, 39(10):8986–8992.
Laine, T., K., K., and & Hellqvist, M. (2013). Simulations
Approaches to Risk, Efficiency, and Liquidity Usage in
Payment Systems. IGI Global.
Leinonen, H. and Soram
¨
aki, K. (2005). Optimising Liq-
uidity Usage and Settlement Speed in Payment Sys-
tems. In Leinonen, H., editor, Liquidity, risks and
speed in payment and settlement systems - a simula-
tion approach., Proceedings from the Bank of Fin-
land Payment and Settlement System Seminars 2005,
pages 115–148.
Le
´
on, C. and P
´
erez, J. (2014). Assessing financial mar-
ket infrastructures’ systemic importance with author-
ity and hub centrality. Journal of Financial Markt In-
frastructures.
Maes, S., Tuyls, K., Vanschoenwinkel, B., and Mander-
ick, B. (2002). Credit Card Fraud Detection Using
Bayesian and Neural Networks. In Proceedings of the
1st international naiso congress on neuro fuzzy tech-
nologies, pages 261–270.
Ng, A. (2011). Sparse autoencoder. CS294A Lecture notes,
72:1–19.
Quah, J. T. and Sriganesh, M. (2008). Real-time credit card
fraud detection using computational intelligence. Ex-
pert systems with applications, 35(4):1721–1732.
Rifai, S., Vincent, P., Muller, X., Glorot, X., and Bengio, Y.
(2011). Contractive Auto-Encoders: Explicit Invari-
ance During Feature Extraction. In Proceedings of
ICEIS 2017 - 19th International Conference on Enterprise Information Systems
440