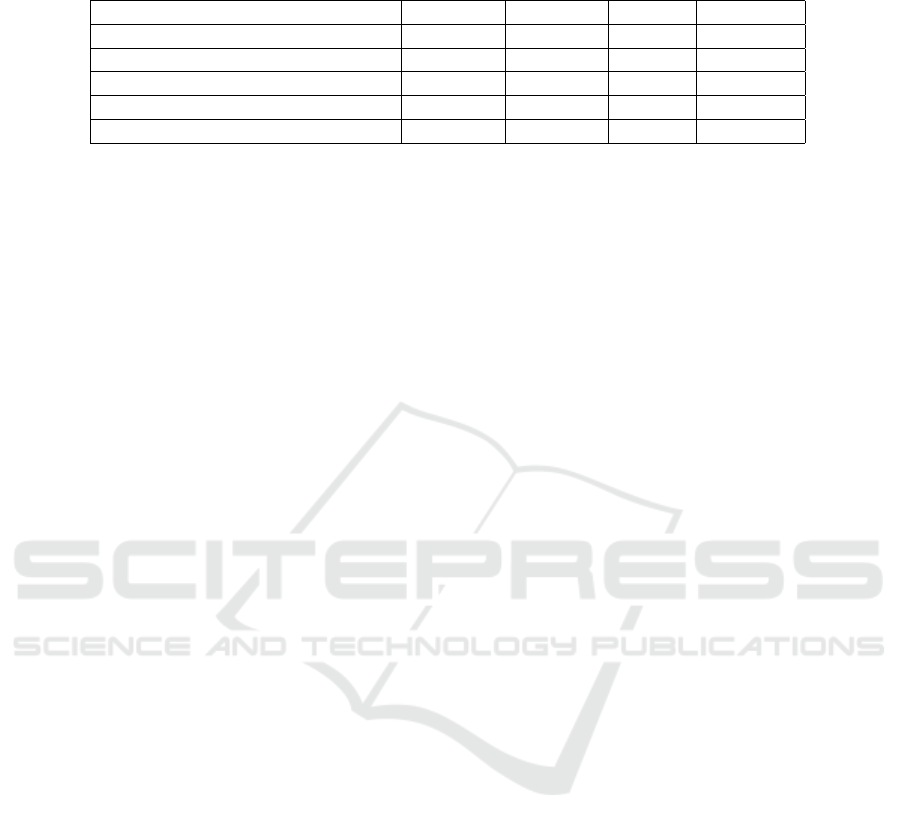
Table 3: Assessment of the quality of the results returned by TAXFRAUDDETECTOR and its competitors when analyzing the
SEFAZ-CE 2011 dataset.
Method Accuracy Precision Recall F
1
Score
TAXFRAUDDETECTOR 81.33% 78.14% 76.08% 77.0962%
(Matos et al., 2015) 51.27% 48.94% 51.11% 50.0014%
SVM using TAXFRAUDDETECTOR 56.26% 53.12% 47.23% 50.0021%
SVM using (Matos et al., 2015) 33.42% 30.07% 39.13% 34.0069%
SVM 87.49% 45.09% 8.22% 13.9112%
analyze them. As such, this scenario represents a typ-
ical complex network, hence a proper analysis of the
underlying topology can offer useful insights into the
network properties.
In the experimental evaluation we show that our
approach achieves F
1
scores of about 54% greater
than (Matos et al., 2015) when considering the dataset
introduced by this work, while it maintains equivalent
F
1
scores when considering the dataset introduced in
(Matos et al., 2015). Furthermore, we show that our
method is able to improve F
1
scores of about 47%
with respect to an SVM-based approach, when con-
sidering the dataset introduced in this work.
As a future line of research, we are considering the
application of other metrics over graph determinants
to further improve the feature selection process; fi-
nally, we plan to explore more issues related to graph
topology, which in turn have the potential to improve
the accuracy of fraud detection.
REFERENCES
Abbott, L. J., Park, Y., and Parker, S. (2000). The effects of
audit committee activity and independence on corpo-
rate fraud. Managerial Finance, 26(11):55–68.
Agrawal, R., Srikant, R., et al. (1994). Fast algorithms for
mining association rules. In Proc. 20th int. conf. very
large data bases, VLDB, volume 1215, pages 487–
499.
Bhattacharyya, S., Jha, S., Tharakunnel, K., and West-
land, J. C. (2011). Data mining for credit card fraud:
A comparative study. Decision Support Systems,
50(3):602–613.
Bland, J. M. and Altman, D. G. (1996). Statistics notes:
measurement error. Bmj, 313(7059):744.
Boccaletti, S., Latora, V., Moreno, Y., Chavez, M., and
Hwang, D.-U. (2006). Complex networks: Structure
and dynamics. Physics reports, 424(4):175–308.
David Meyer (2017). Support Vector Machines. FH Tech-
nikum Wien, Austria.
Fanning, K. M. and Cogger, K. O. (1998). Neural network
detection of management fraud using published finan-
cial data. International Journal of Intelligent Systems
in Accounting, Finance & Management, 7(1):21–41.
Glancy, F. H. and Yadav, S. B. (2011). A computational
model for financial reporting fraud detection. Deci-
sion Support Systems, 50(3):595–601.
Golub, G. H. and Reinsch, C. (1970). Singular value de-
composition and least squares solutions. Numerische
mathematik, 14(5):403–420.
Kirkos, E., Spathis, C., and Manolopoulos, Y. (2007). Data
mining techniques for the detection of fraudulent fi-
nancial statements. Expert systems with applications,
32(4):995–1003.
Kohavi, R. et al. (1995). A study of cross-validation and
bootstrap for accuracy estimation and model selection.
In Ijcai, volume 14, pages 1137–1145.
Li, S.-H., Yen, D. C., Lu, W.-H., and Wang, C. (2012).
Identifying the signs of fraudulent accounts using data
mining techniques. Computers in Human Behavior,
28(3):1002–1013.
Matos, T., de Macedo, J. A. F., and Monteiro, J. M. (2015).
An empirical method for discovering tax fraudsters: A
real case study of brazilian fiscal evasion. In Proceed-
ings of the 19th International Database Engineering
& Applications Symposium, pages 41–48. ACM.
Montgomery, D. C. (2007). Introduction to statistical qual-
ity control. John Wiley & Sons.
Ngai, E., Hu, Y., Wong, Y., Chen, Y., and Sun, X. (2011).
The application of data mining techniques in finan-
cial fraud detection: A classification framework and
an academic review of literature. Decision Support
Systems, 50(3):559–569.
Phua, C., Lee, V., Smith, K., and Gayler, R. (2010). A
comprehensive survey of data mining-based fraud de-
tection research. arXiv preprint arXiv:1009.6119.
R Core Team (2016). R: A Language and Environment for
Statistical Computing - version 0.99.903. R Founda-
tion for Statistical Computing, Vienna, Austria.
Ravisankar, P., Ravi, V., Rao, G. R., and Bose, I. (2011).
Detection of financial statement fraud and feature se-
lection using data mining techniques. Decision Sup-
port Systems, 50(2):491–500.
S
´
anchez, D., Vila, M., Cerda, L., and Serrano, J.-M. (2009).
Association rules applied to credit card fraud detec-
tion. Expert Systems with Applications, 36(2):3630–
3640.
Shavers, C., Li, R., and Lebby, G. (2006). An svm-based
approach to face detection. In 2006 Proceeding of
the Thirty-Eighth Southeastern Symposium on System
Theory, pages 362–366. IEEE.
Sonegometro (2016 (Retrieved December 14,
2016)). Tax Evasion in Brazil. http://
www.quantocustaobrasil.com.br.
Tan, P.-N., Steinbach, M., and Kumar, V. (2005). Introduc-
tion to Data Mining, (First Edition). Addison-Wesley
Longman Publishing Co., Inc., Boston, MA, USA.
An Accurate Tax Fraud Classifier with Feature Selection based on Complex Network Node Centrality Measure
151