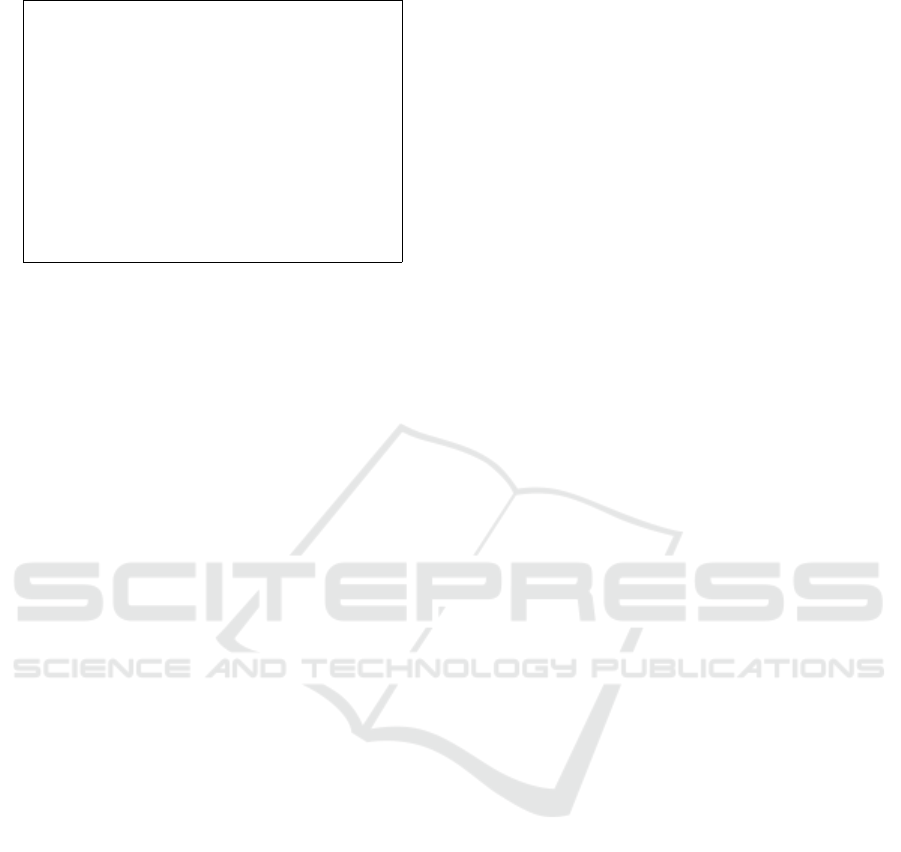
Table 2: Some workshop questions about data.
• Q.UD.1 What are the data sources and data types
used in your current business processes?
• Q.UD.2 What tools/applications are used to deal
with your current business processes?
• Q.UD.3 Are your present business processes per-
forming complex processing on data?
• Q.UD.4 How available is your data? What happens
if data is not available?
• Q.UD.5 Do different users have different access rig-
hts on your data?
• Q.UD.6 Does your data contain sensitive informa-
tion (e.g. personal or company confidential data)?
cause they only focus on a specific function and can-
not provide a good global picture of the problem.
5 CONCLUSIONS
In this paper, we described how we addressed the
challenges and risks of deploying a Big Data solu-
tion within companies willing to adopt them to sup-
port their business development. Based on different
methods and experience reports for the literature, we
came up with a method fitting our needs and con-
tinuing to evolve as we explore more uses cases, while
highlighting a number of lessons learned.
When considering the adoption of Big Data ana-
lytics in organisations, what is crucial is the process
followed to come up with a method that will maxi-
mize the chance of success and will fits the needs of
each specific organisation.
Moving forward, we plan to consolidate our work
based on what we will learn in the next series of pro-
ject case studies. So far, we have also focused more
on the discovery and data understanding phases. We
plan to provide more guidance on the project execu-
tion phase when enough pilot projects have reached
completion or key milestones.
ACKNOWLEDGEMENTS
This research was partly funded by the Walloon Re-
gion through the ”PIT Big Data” project (nr 7481).
We thank our industrial partners for sharing their ca-
ses and contributions to the method assessments.
REFERENCES
Balduino, R. (2007). Introduction to OpenUP.
https://www.eclipse.org/epf/general/OpenUP.pdf.
Bedos, T. (2015). 5 key things to make big data analytics
work in any business. http://www.cio.com.au.
Chen, H.-M., Kazman, R., and Haziyev, S. (2016). Agile
big data analytics development: An architecture-
centric approach. In Proc. HICSS’16, Hawaii, USA.
Corea, F. (2016). Big Data Analytics: A Management Per-
spective. Springer Publishing Company, Inc.
Crowston, K. (2010). A capability maturity model for scien-
tific data management.
do Nascimento, G. S. and de Oliveira, A. A. (2012). An
Agile Knowledge Discovery in Databases Software
Process. Springer Berlin Heidelberg.
Frankov, P., Drahoov, M., and Balco, P. (2016). Agile pro-
ject management approach and its use in big data ma-
nagement. Procedia Computer Science, 83.
Gao, J., Koronios, A., and Selle, S. (2015). Towards A
Process View on Critical Success Factors in Big Data
Analytics Projects. In AMCIS.
Gartner (2016). Investment in big data is up but fewer orga-
nizations plan to invest. http://www.gartner.com.
IBM (2013). Stampede.
http://www.ibmbigdatahub.com/tag/1252.
Kelly, J. and Kaskade, J. (2013). CIOs & Big
Data: What Your IT Team Wants You to Know.
http://blog.infochimps.com/2013/01/24/cios-big-data.
Lau, L. et al. (2014). Requirements for big data analy-
tics supporting decision making: A sensemaking per-
spective. In Mastering data-intensive collaboration
and decision making. Springer Science & Business
Media.
Mariscal, G. et al. (2010). A survey of data mining and kno-
wledge discovery process models and methodologies.
Knowledge Eng. Review, 25(2):137–166.
Mauro, A. D., Greco, M., and Grimaldi, M. (2016). A for-
mal definition of big data based on its essential featu-
res. Library Review, 65(3):122–135.
Nott, C. (2014). Big Data & Analytics Maturity Mo-
del. http://www.ibmbigdatahub.com/blog/big-data-
analytics-maturity-model.
Rot, E. (2015). How Much Data Will You Have in 3 Ye-
ars? http://www.sisense.com/blog/much-data-will-3-
years.
Saltz, J. and Shamshurin, I. (2016). Big Data Team Process
Methodologies: A Literature Review and the Identifi-
cation of Key Factors for a Projects Success. In Proc.
IEEE International Conference on Big Data.
Saltz, J. S. (2015). The need for new processes, methodolo-
gies and tools to support big data teams and improve
big data project effectiveness. In IEEE Int. Conf. on
Big Data, Big Data 2015, Santa Clara, CA, USA.
Scrum Alliance (2016). What is scrum? an
agile framework for completing complex projects.
https://www.scrumalliance.org/why-scrum.
Shearer, C. (2000). The CRISP-DM Model: The New Blu-
eprint for Data Mining. Journal of Data Warehousing,
5(4).
Process Guidance for the Successful Deployment of a Big Data Project: Lessons Learned from Industrial Cases
355