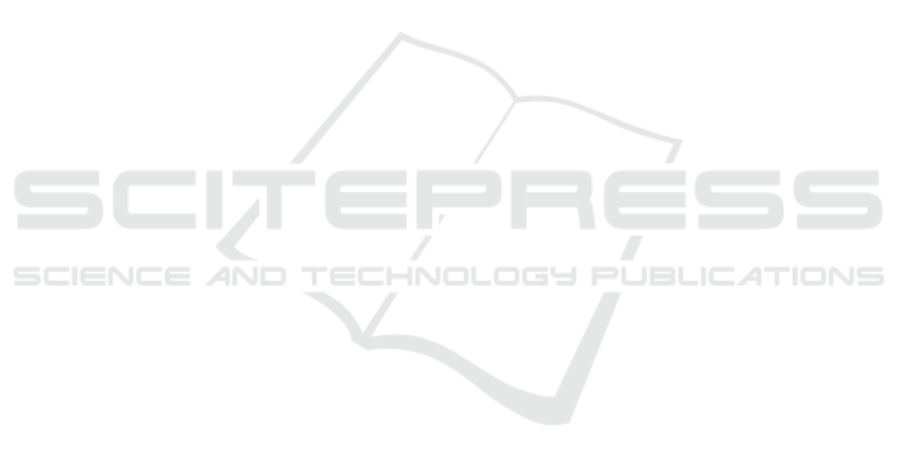
with a one-time specified fixed price which minimi-
zes staff costs (1). As an alternative to these contracts
different kinds of structured energy supply contracts
are offered, which allow buying energy at the future
market in advance and trading at the spot market to
adjust the previously bought energy to the actual de-
mand (2). Thus the company is given the ability to
cope with price and volume risks. For large industrial
enterprises there is also the option to realize their own
portfolio management which offers the most degrees
of freedom but also requires a constant market obser-
vation (3).
One example of such a large enterprise is the Stutt-
gart Airport, which is a partner in the SmartEnergy-
Hub project. Based on this pilot user, this work des-
cribes the complex decision-making problem related
to energy procurement in large infrastructures. Ba-
sed on this, a simulation based decision support sy-
stem is developed to support the infrastructure opera-
tor to answer the following question: Which quantity
of energy should be bought at which time via which
product?
This work is structured as follows. Section 2 gives
an overview of models and approaches, which offer
solutions to the problem of finding optimal procure-
ment strategies either for SMEs, large companies or
energy suppliers. Section 3 takes a closer look at in-
dividual aspects of the procurement problem introdu-
cing a systematic methodology to describe the solu-
tion space. Following this methodology it is explai-
ned how this solution space is shaped in the example
of the Stuttgart Airport. In Section 4, the prototype
implementation of a decision support system based
on a Monte Carlo simulation is described. Section 5
describes how this software component, which is one
part of the SmartEnergyHub architecture, is used to
find appropriate procurement plans. Finally, Section 6
gives a conclusion and an outlook on future work.
2 RELATED WORK
In this section, we examine the areas of energy procu-
rement decisions both from the perspective of consu-
mers, energy suppliers and distributors.
(Kumbartzky and Werners, 2016) focus on op-
timal energy procurement strategies from the per-
spective of SMEs based on a two-stage optimiza-
tion model. Stochastic influences concerning prices
and energy demand are taken into account by the in-
troduction of a finite number of scenarios. For all
scenarios optimal procurement strategies are found
and compared by a minimax relative regret appro-
ach. Contract costs are split up to allow modeling
take-or-pay clauses as well as additional charges for
excess capacities consumed. In a case study the cost
saving potential of structured procurement strategies
are shown.
Monte Carlo simulations are a numerical simula-
tion technique and a wide spread approach for exam-
ple for the derivation of an optimal portfolio as des-
cribed in (Cvitani
´
c et al., 2003) and (Boyle et al.,
2008). Variants of Monte Carlo simulations like the
least squares Monte Carlo is used to determine energy
option value in (Nadarajah et al., 2017).
In (Prokopczuk et al., 2007) a Monte Carlo simu-
lation based model is developed to quantify risks re-
lated to wholesale electricity contracts from the per-
spective of an electricity supplier, who is able to di-
versify unsystematic risk through a large portfolio of
many customers. Within the model the Risk-adjusted-
Return-on-Capital is used as a risk measure. Price
risks are modeled using the SMaPS (Spot Market
Price Simulation) developed in (Burger et al., 2004).
Uncertainties related to the energy demand are de-
rived by correlating spot market prices to individual
load curves thus simulating individual load paths. It
is argued that a supplier will offer contracts at a price
reflecting risk premiums for the hourly spot market
price risk, a risk premium for the volume risk and a
risk premium related to the price-volume correlation.
In (Woo et al., 2004) procurement strategies for
local distribution companies are developed. Such a
company has three possibilities to satisfy customers’
electricity demand namely through self-generation,
spot market transactions and forward-contracting. A
heuristic procedure is presented to minimize expected
procurement costs for a given risk tolerance level re-
sulting in the determination of the energy amount
which will be bought in advance in future-contracts.
Price structures in German energy market are the
subject of (Pietz, 2009) where the presence of risk
premiums in German electricity market with a focus
on month futures is investigated. It is concluded that
there is evidence for positive risk premia, which de-
creases with increasing time-to-delivery. Similarly,
(Daskalakis et al., 2015) investigate electricity risk
premia in the European market and find a correlation
with the volatility of the spot market and carbon diox-
ide futures, concluding that carbon price fluctuations
are a factor in discounts for electricity consumers.
(Hong and Lee, 2013) focus on an energy con-
sumer who can choose among different suppliers to
meet the energy demand. A Monte Carlo simulation
is used to quantify each supplier’s risk and to allo-
cate orders among multiple suppliers. (Bessembinder
and Lemmon, 2002) focus on optimal forward posi-
tions for energy producing and retailing firms using
SMARTGREENS 2017 - 6th International Conference on Smart Cities and Green ICT Systems
78