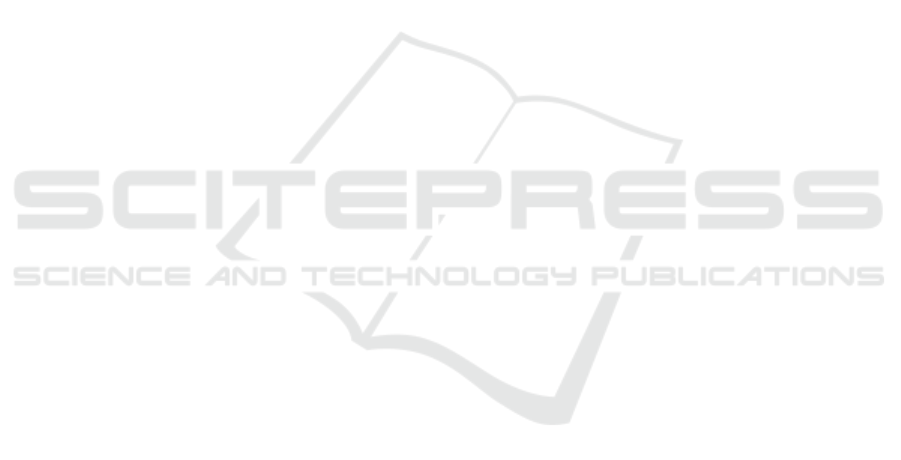
ACKNOWLEDGEMENTS
The authors thank all participants for their patience
and openness to share opinions. Furthermore, the
authors want to thank Valentina Kneip for research
assistance. This work was funded by the Excellence
Initiative of the German State and Federal Govern-
ment (Project Urban Future Outline).
REFERENCES
Aghajan, H., Augusto, J. C., Wu, C., McCullagh, P., &
Walkden, J.-A. 2007. Distributed vision-based acci-
dent management for assisted living. In International
conference on Smart homes and health telematics, pp.
196–205, Springer.
Ajzen, I., Fishbein, M. 1977. Attitude-behavior relations:
A theoretical analysis and review of empirical re-
search. Psychological Bulletin, 84(5), 888–918.
https://doi.org/10.1037/0033-2909.84.5.888.
Arning, K., Ziefle, M., 2015. “Get that Camera Out of My
House!” Conjoint Measurement of Preferences for
Video-Based Healthcare Monitoring Systems in Pri-
vate and Public Places. In Inclusive Smart Cities and
e-Health, pp. 152–164. Springer.
Barrett, D., 2013. One surveillance camera for every 11
people in Britain, says CCTV survey. Telegraph. 10
th
July 2013.
Bowyer, K. W., 2004. Face recognition technology: secu-
rity versus privacy. IEEE Technology and Society
Magazine, 23(1), 9–19.
https://doi.org/10.1109/MTAS.200 4.1273467.
Cardinaux, F., Bhowmik, D., Abhayaratne, C., Hawley,
M. S., 2011. Video based technology for ambient as-
sisted living: A review of the literature. Journal of
Ambient Intelligence and Smart Environments, 3(3),
253–269.
Chen, B.-W., Chen, C.-Y., & Wang, J.-F., 2013. Smart
homecare surveillance system: Behavior identification
based on state-transition support vector machines and
sound directivity pattern analysis. IEEE Transactions
on Systems, Man, and Cybernetics: Systems, 43(6),
1279–1289.
Dailey, K., 2013. The rise of CCTV surveillance in the
US. BBC News. 29
th
April 2013.
Davis, F. D., Bagozzi, R. P., Warshaw, P. R., 1989. User
acceptance of computer technology: a comparison of
two theoretical models. Management Science, 982–
1003.
Dey, S., Chakraborty, A., Naskar, S., Misra, P., 2012.
Smart city surveillance: Leveraging benefits of cloud
data stores. In 37th Conference on Local Computer
Networks Workshops (LCN Workshops), 2012, pp.
868–876. IEEE.
Filipponi, L., Vitaletti, A., Landi, G., Memeo, V., Laura,
G., Pucci, P., 2010. Smart city: An event driven archi-
tecture for monitoring public spaces with heterogene-
ous sensors. In Fourth International Conference on
Sensor Technologies and Applications
(SENSORCOMM), 2010, pp. 281–286. IEEE.
Fleck, S., Strasser, W., 2008. Smart camera based moni-
toring system and its application to assisted living.
Proceedings of the IEEE, 96(10), 1698–1714.
Fyfe, N., 2004. Zero tolerance, maximum surveillance?
Deviance, difference and crime control in the late
modern city. The Emancipatory City, 40–56.
Gurley, R. J., Lum, N., Sande, M., Lo, B., Katz, M. H.,
1996. Persons Found in Their Homes Helpless or
Dead. New England Journal of Medicine, 334(26),
1710–1716.
https://doi.org/10.1056/NEJM199606273342606.
Himmel, S., Ziefle, M., Arning, K. (2013). From Living
Space to Urban Quarter: Acceptance of ICT Monitor-
ing Solutions in an Ageing Society. In Human-
Computer Interaction. Users and Contexts of Use, pp.
49–58. Springer.
Kim, J. E., Boulos, G., Yackovich, J., Barth, T., Beckel,
C., Mosse, D., 2012. Seamless Integration of Hetero-
geneous Devices and Access Control in Smart Homes.
In 2012 Eighth International Conference on Intelligent
Environments, pp. 206–213.
https://doi.org/10.1109/IE.2012.57.
Klack, L., Schmitz-Rode, T., Wilkowska, W., Kasugai, K.,
Heidrich, F., Ziefle, M., 2011. Integrated Home Moni-
toring and Compliance Optimization for Patients with
Mechanical Circulatory Support Devices. Annals of
Biomedical Engineering, 39(12), 2911.
https://doi.org/10. 1007/s10439-011-0407-1.
Koh, Y., Mohan, A., Wang, G., Xu, H., Malik, A., Lu, Y.
H., & Ebert, D. S. (2016, May). Improve safety using
public network cameras. In Technologies for Home-
land Security (HST), 2016 IEEE Symposium on (pp.
1-5). IEEE.
La Vigne, N. G., Lowry, S. S., Markman, J. A., & Dwyer,
A. M. (2011). Evaluating the use of public surveil-
lance cameras for crime control and prevention. Wash-
ington, DC: US Department of Justice, Office of
Community Oriented Policing Services. Urban Insti-
tute, Justice Policy Center.
Leonhardt, S. (2006). Personal Healthcare Devices. In S.
Mukherjee, R. M. Aarts, R. Roovers, F. Widder-
shoven, & M. Ouwerkerk (Eds.), AmIware Hardware
Technology Drivers of Ambient Intelligence, pp. 349–
370. Springer Netherlands.
Luce, R. D., Tukey, J. W., 1964. Simultaneous conjoint
measurement: A new type of fundamental measure-
ment. Journal of Mathematical Psychology, 1(1), 1–
27.
Memon, M., Wagner, S. R., Pedersen, C. F., Beevi, F. H.
A., & Hansen, F. O., 2014. Ambient assisted living
healthcare frameworks, platforms, standards, and qual-
ity attributes. Sensors, 14(3), 4312–4341.
Orme, B. K., 2010. Getting started with conjoint analysis:
strategies for product design and pricing research, 77–
89.
Patton, J. W., 2000. Protecting privacy in public? Surveil-
lance technologies and the value of public places. Eth-
Where, Wherefore, and How? - Contrasting Two Surveillance Contexts According to Acceptance
97