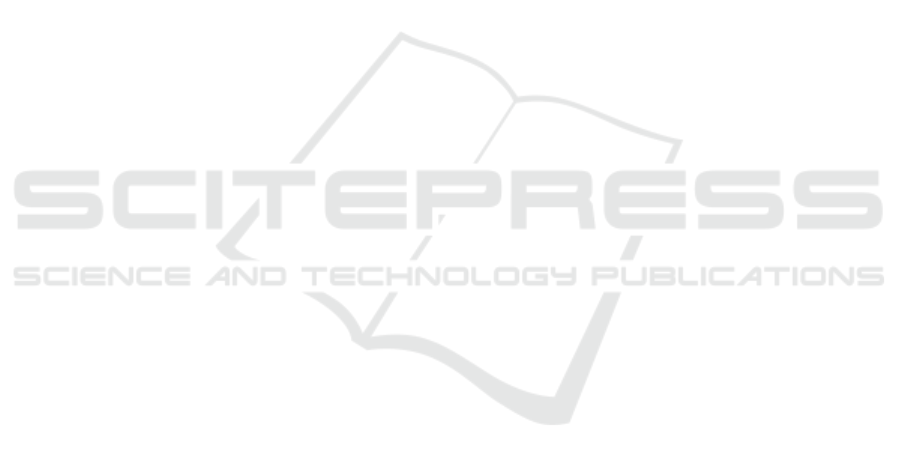
to reactive students in case they lose their attention
to the lessons. The impact of teachers in the
interactive learning process in MOOCs is reduced to
a minimum. Everything should be included in the
learning material.
We discussed our layered ordering of the
learning material to enable students to select their
individual learning paths of different difficulty
degree. But the learning material should include
much more stimuli to attract and keep the attention
of students and stimulate them to learning activities.
We presented a new didactic approach modelling the
learning activities and study interaction students
with the MOOC. The basic idea is that the learning
process of students is fuelled by a study liquid.
Several resources consume this energy liquid and
other resources provide new liquid. When the liquid
drops below some threshold, the learning process
will stop and probably a student drop-out. In face to
face learning it can happen that students lost their
attention and are not able to process the presented
material anymore. But students are not able to leave
the classroom and a didactically gifted teacher is
able to get students back in the learning process.
During MOOCs learning the situation is different.
We stressed that peers are important because they
are able to support students and because of social
interaction in peer groups they take part in the
lessons.
Emotions play an important role in our model.
We realised that students should be attracted by the
learning material, they should like the interaction
and their motivation to follow the MOOCs should
not decrease. Based on our new didactic model we
designed some didactic stimuli to re-activate
students during the learning activities. The
communication of students with the Learning
Management System is modelled by using emotional
buttons, keywords, one-liners and by facial
expression. The recognition of a positive or negative
emotional state of the student will result in special
adaptation in the flow of the learning material.
Students can go back to lower level or more
attractive study activities as movies, simulations etc.
Next future we envision a digital tutor at the end
who is able to supervise and improve the learning
process of students. Such a tutor requires
improvement of the needed technology for e-
learning. We discussed already the option of
multipath, streaming and circular ways of learning.
But unfortunately many public domain tools of e-
learning are still based on a linear approach. But
recent developments around e-learning and MOOCs
are promising.
REFERENCES
Armellini, A., Padilla Rodriguez, B.C. (2016). Are
massive Open Online Courses pedagogically
innovative? Journal of Interactive Online Learning,
Volume 14, Number 1.
Colman, D. (2013). MOOC interrupted: top 10 reasons our
readers didn’t finish a Massive Open Online Courser.
User-Centered Design Strategies for Massive Open
Online Courses (MOOCs). IGI Global.
Desmet, P. (2012). Designing emotions PhD thesis Delft
University of Technology.
Dillon, J., Bosch, N., Chetlur, M. (2016). Student emotion,
co-occurrence and dropout in a MOOC context. in:
Proceedings of the 9th International Conference on
educational data mining. June 29-July 2. Raleigh,
North Carolina. 1-5.
Fitrianie, S., Rothkrantz, L. (2007) A visual
communication language for crisis management.
International Journal of Intelligent Control and
Systems 12, 2.
Fitrianie,S., Wiggers, P., Rothkrantz, L.J.M. (2003). A
multi-modal Eliza using natural language processing
and emotion recognition. in: International Conference
on Text, Speech and Dialogue, 394-399.
Leony, D., Munoz-merino, P.J., Ruiperez-Valiente, J. A.,
Pardo, A. Kloos, C. D., (2015). Detection and
evaluation of emotions in Massive Open Online
courses. Journal of Universal Computer Science, vol.
21, no 5, 638-655.
Niett van der, A. G., Smith, J., Scherder, E. J. A.,
Oosterlaan, J., Hartman, E., & Visscher, C. (2015).
Associations between daily physical activity and
executive functioning in primary school-aged children.
Journal of science and medicine in sport, 18(6), 673-
677.
Rothkrantz, L. Wojdel, A. (2000). A text based talking
face. in: Proceedings Conference on Text, Speech and
Dialogues, pp 231-2140.
Rothkrantz, L., Datcu, D., Chiriacescu, I., Chitu, A.
(2009). Assessment of the emotional states of students
during e-Learning, in: Proceedings of the
International Conference on e-Learning and
Knowledge Society, pp. 77-82.
Rothkrantz, L., Datcu, D. (2010). Assessment of Emotion
States During e-Learning. Communication &
Cognition, 43 (1), 81.
Rothkrantz, L.J.M., (2015). How Social Media Facilitate
Learning Communities and Peer Groups around
MOOCS. International Journal of Human Capital and
Information Technology Professionals, 6, 1-13.
Rothkrantz, L. (2016a). Dropout rates of regular courses
and MOOCs, in: 8th International Conference on
Computer Supported Education. 21-23 April, Rome.
Rothkrantz, L. (2016b). Didactic Model for e-Learning
and Regular Courses. in: International Conference on
e-Learning. 8-9 September, Bratislava. pp. 156-161.
Tak-ming Wong, B. (2016). Factors leading to effective
teaching of MOOCs. Asian Association of Open
Universities Journal, Vol. 11 Iss 1 pp. 105 – 118.
Tettegah, S. Y., McCreery, M.P.. (EDS), (2016).
Emotions, Technology, and Learning. Elsevier.
Weiss, J., Nolan, J., Hunsinger, J., Trifonas, P., (2006).
The international handbook of virtual learning
environments, vols. I & II, Springer, The Netherlands.
CSEDU 2017 - 9th International Conference on Computer Supported Education
512