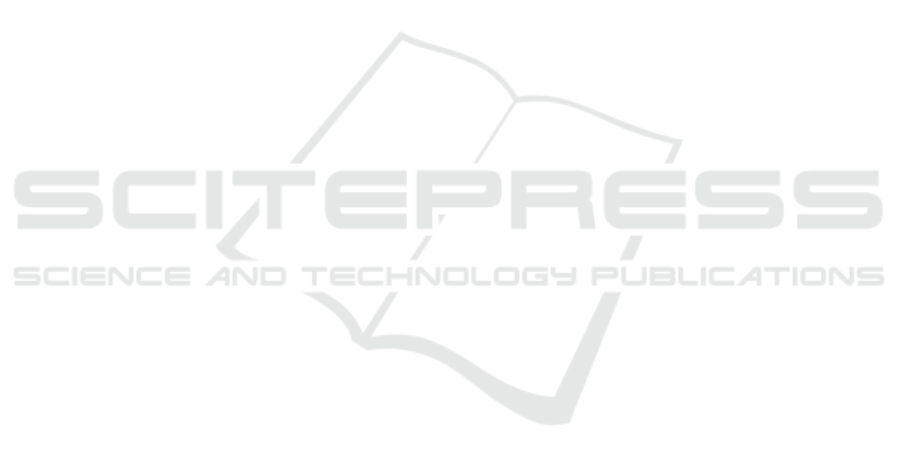
ture for wireless transmission of electricity for res-
idential needs. Within the wIshood, the energy is
generated, stored and dispatched to the households.
We envision, an intensive deployment of IoT devices,
cloud computing and a wireless power transmission
of scale. This paper outlined the architectural founda-
tion and algorithms to address the challenges of such
an ecosystem.
Future work intends to simulate components of
the wIshood architecture. We plan to develop two al-
gorithms. Firstly, a reduction of household data to
stream to the Information layer. Secondly, a Recur-
rent Neural Network for energy demand forecasting.
REFERENCES
Akcin, M., Kaygusuz, A., Karabiber, A., Alagoz, S.,
Alagoz, B. B., and Keles, C. (2016). Opportunities
for energy efficiency in smart cities. In 4th Int. Istan-
bul Smart Grid Cong. and Fair (ICSG), pages 1–5.
Bashir, M. R. and Gill, A. Q. (2016). Towards an iot big
data analytics framework: Smart buildings systems. In
2016 IEEE 18th Int. Conf. on High Performance Com-
puting and Comms; IEEE 14th Int. Conf. on Smart
City; IEEE 2nd Int. Conf. on Data Science and Sys-
tems (HPCC/SmartCity/DSS), pages 1325–1332.
Beckel, C., Kleiminger, W., Cicchetti, R., Staake, T., and
Santini, S. (2014). The eco data set and the perfor-
mance of non-intrusive load monitoring algorithms. In
Proceedings of the 1st ACM Conference on Embedded
Systems for Energy-Efficient Buildings, BuildSys ’14,
pages 80–89, New York, NY, USA. ACM.
Bellavista, P., Cardone, G., Corradi, A., and Foschini, L.
(2013). Convergence of manet and wsn in iot urban
scenarios. IEEE Sensors Jounral, 13(10):3558–3567.
Cakmak, R. and Altas, I. H. (2016). Scheduling of domestic
shiftable loads via cuckoo search optimization algo-
rithm. In 2016 4th International Istanbul Smart Grid
Congress and Fair (ICSG), pages 1–4.
Feenaghty, M. and Dahle, R. (2016). A compact and high
quality factor archimedean coil geometry for wireless
power transfer. In 2016 IEEE Wireless Power Transfer
Conference (WPTC), pages 1–3.
Hou, L., Zhao, S., Xiong, X., Zheng, K., Chatzimisios, P.,
Hossain, M. S., and Chen, W. (2016). Internet of
things cloud: Architecture and implementation. IEEE
Communications Magazine, 54(12):32–39.
Imura, T., Yasuda, T., Oshima, K., Nayuki, T., Sato, M.,
and Oshima, A. (2016). Wireless power transfer for
electric vehicle at the kilohertz band. IEEJ Trans. on
Electrical and Electronic Engineering, 11:S91–S99.
Jian, M. S., Fang, Y. C., Tong, R. W., and Lin, Y. H.
(2016). Wireless green energy power transmission
system based on assembly method and pivoting an-
tenna module. In 2016 International Conference on
Applied System Innovation (ICASI), pages 1–4.
Jo, H. C. and Jin, H. W. (2015). Adaptive periodic commu-
nication over mqtt for large-scale cyber-physical sys-
tems. In 2015 IEEE 3rd Int. Conf. on Cyber-Physical
Systems, Networks, and Applications, pages 66–69.
Khan, A. A., Rehmani, M. H., and Rachedi, A. (2016).
When cognitive radio meets the internet of things? In
2016 Int. Wireless Communications and Mobile Com-
puting Conference (IWCMC), pages 469–474.
Kyriazopoulou, C. (2015). Smart city technologies and ar-
chitectures: A literature review. In 2015 International
Conference on Smart Cities and Green ICT Systems
(SMARTGREENS), pages 1–12.
Liu, Y., Yuen, C., Huang, S., Hassan, N. U., Wang, X.,
and Xie, S. (2014). Peak-to-average ratio constrained
demand-side management with consumer’s prefer-
ence in residential smart grid. IEEE Journal of Se-
lected Topics in Signal Processing, 8(6):1084–1097.
Ma, H., Li, X., Sun, L., Xu, H., and Yang, L. (2016).
Design of high-efficiency microwave wireless power
transmission system. Microwave and Optical Tech-
nology Letters, 58(7):1704–1707.
McLean, J. and Sutton, R. (2016). Electric field breakdown
in wireless power transfer systems due to ferrite di-
electric polarizability. In 2016 IEEE Wireless Power
Transfer Conference (WPTC), pages 1–4.
PervasiveNation (2016). Ireland’s Internet of Things
Testbed. https://connectcentre.ie/pervasive-nation/.
Accessed: 19-Dec-2016.
Pingle, Y., Dalvi, S. N., Chaudhari, S. R., and Bhatkar, P.
(2016). Electricity measuring iot device. In 2016 3rd
Int. Conf. on Computing for Sustainable Global De-
velopment (INDIACom), pages 1423–1426.
Samarakoon, S., Bennis, M., Saad, W., Debbah, M., and
Latva-aho, M. (2016). Ultra dense small cell net-
works: Turning density into energy efficiency. IEEE
J. Sel. Areas Commun., 34(5):1267–1280.
Shinohara, N. (2014). Wireless Power Transfer via Ra-
diowaves. Wiley, New Jersey.
Tobar, A. C., Banna, H. U., and Koch-Ciobotaru, C. (2014).
Scope of electrical distribution system architecture
considering the integration of renewable energy in
large and small scale. In IEEE PES Innovative Smart
Grid Technologies, Europe, pages 1–7.
Yoon, J. H., Baldick, R., and Novoselac, A. (2014). Dy-
namic demand response controller based on real-time
retail price for residential buildings. IEEE Transac-
tions on Smart Grid, 5(1):121–129.
Zhu, L., Yan, Z., Lee, W. J., Yang, X., Fu, Y., and Cao,
W. (2015). Direct load control in microgrids to en-
hance the performance of integrated resources plan-
ning. IEEE Trans. Ind. Appl., 51(5):3553–3560.
SMARTGREENS 2017 - 6th International Conference on Smart Cities and Green ICT Systems
322