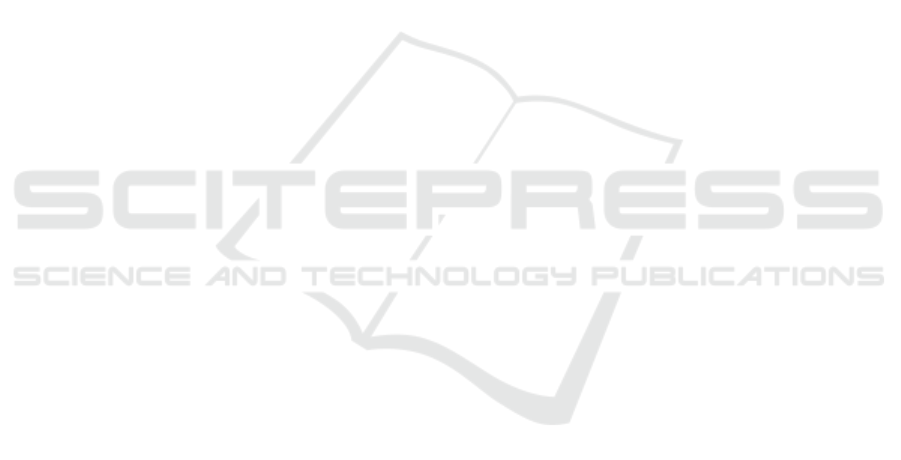
Our new bootstrapping approach also gives rise to
the several challenges:
Bootstrapping Processes. We need research to un-
derstand and model bootstrapping processes, similar
to our example, in order to obtain a more complete
picture of the techniques and services that are avail-
able. Also, most of the services described in Sec-
tion 2.1 are open challenges mainly due to the inher-
ent complexity of indoor localization: existing sen-
sors (both in phones and specialized devices) fail to
effectively propagate a discrete signal patterns in in-
door space, making simple triangulation-based tech-
niques infeasible. Additionally, robust heading direc-
tion identification independent of the phone’s pose re-
mains an open challenge (Zhou et al., 2012).
Intermediate Targets/Artifacts. We need to
understand what can be useful intermediate tar-
gets/artifacts, which are both feasible w.r.t mapping
techniques and also enable useful services. More-
over, protocols need to be emerged to enable informa-
tion exchange through APIs between the different ser-
vices. Importantly, we need to manage the uncertainty
inherent to both sensor reading and human users, fil-
ter out outliers, and in general work with noisy data.
Trust models to manage ambiguous information ex-
tracted from multiple users need to be emerged. Ex-
isting indoor data models have to be enhanced in order
to cope with such incomplete, ambiguous or inaccu-
rate models.
Process Customization. We need research to under-
stand when and how to apply different bootstrapping
processes to specific buildings. This can also lead to
easier or automatic customization of bootstrapping to
specific classes of buildings.
ACKNOWLEDGMENTS
This work is part of the TUM Living Lab Connected
Mobility project and has been funded by the Bay-
erisches Staatsministerium f
¨
ur Wirtschaft und Me-
dien, Energie und Technologie.
REFERENCES
(2012). ISO/TS 12911:2012 - Framework for building in-
formation modelling (BIM) guidance.
(2013). ISO 16739:2013 - Industry Foundation Classes
(IFC) for data sharing in the construction and facility
management industries.
(2016). OGC IndoorGML version 1.0.2.
http://www.opengeospatial.org/standards/indoorgml.
Alzantot, M. and Youssef, M. (2012). CrowdInside: Auto-
matic Construction of Indoor Floorplans. In SIGSPA-
TIAL ’12, pages 99–108. ACM.
Eaglin, T., Subramanian, K., and Payton, J. (2013). 3D
modeling by the masses: A mobile app for modeling
buildings. In Proc. of PERCOM ’13 Workshops, pages
315–317. IEEE.
El-Hakim, S. F. and Boulanger, P. (1999). Mobile system
for indoor 3-d mapping and creating virtual environ-
ments. US Patent 6,009,359.
Gao, R., Zhao, M., Ye, T., Ye, F., Wang, Y., Bian, K., Wang,
T., and Li, X. (2014). Jigsaw: indoor floor plan recon-
struction via mobile crowdsensing. In Proc. of Mobi-
Com ’14, pages 249–260. ACM.
Henry, P., Krainin, M., Herbst, E., Ren, X., and Fox, D.
(2012). RGB-D mapping: Using Kinect-style depth
cameras for dense 3D modeling of indoor environ-
ments. Int. J. Robot. Res., 31(5):647–663.
Karlsson, N., Di Bernardo, E., Ostrowski, J., Goncalves,
L., Pirjanian, P., and Munich, M. E. (2005). The vs-
lam algorithm for robust localization and mapping. In
Robotics and Automation, 2005. ICRA 2005. Proceed-
ings of the 2005 IEEE International Conference on,
pages 24–29. IEEE.
Kourogi, M. and Kurata, T. (2014). A method of pedes-
trian dead reckoning for smartphones using frequency
domain analysis on patterns of acceleration and angu-
lar velocity. In Proc. of PLANS ’14, pages 164–168.
IEEE.
Liu, H., Shi, R., Zhu, L., and Jing, C. (2014). Conver-
sion of model file information from IFC to GML. In
IGARSS’14, pages 3133–3136. IEEE.
Mautz, R. (2012). Indoor positioning technologies. ETH
Zurich, Department of Civil, Environmental and Geo-
matic Engineering.
Nguyen, P. et al. (2015). User-friendly activity recogni-
tion using SVM classifier and informative features. In
IPIN’15, pages 1–8.
Roy, N., Wang, H., and Roy Choudhury, R. (2014). I am a
smartphone and i can tell my user’s walking direction.
pages 329–342. ACM Press.
Zhou, P., Zheng, Y., Li, Z., Li, M., and Shen, G. (2012).
Iodetector: A generic service for indoor outdoor de-
tection. In SenSys ’12, SenSys ’12, pages 113–126.
ACM.
Adaptive Bootstrapping for Crowdsourced Indoor Maps
289