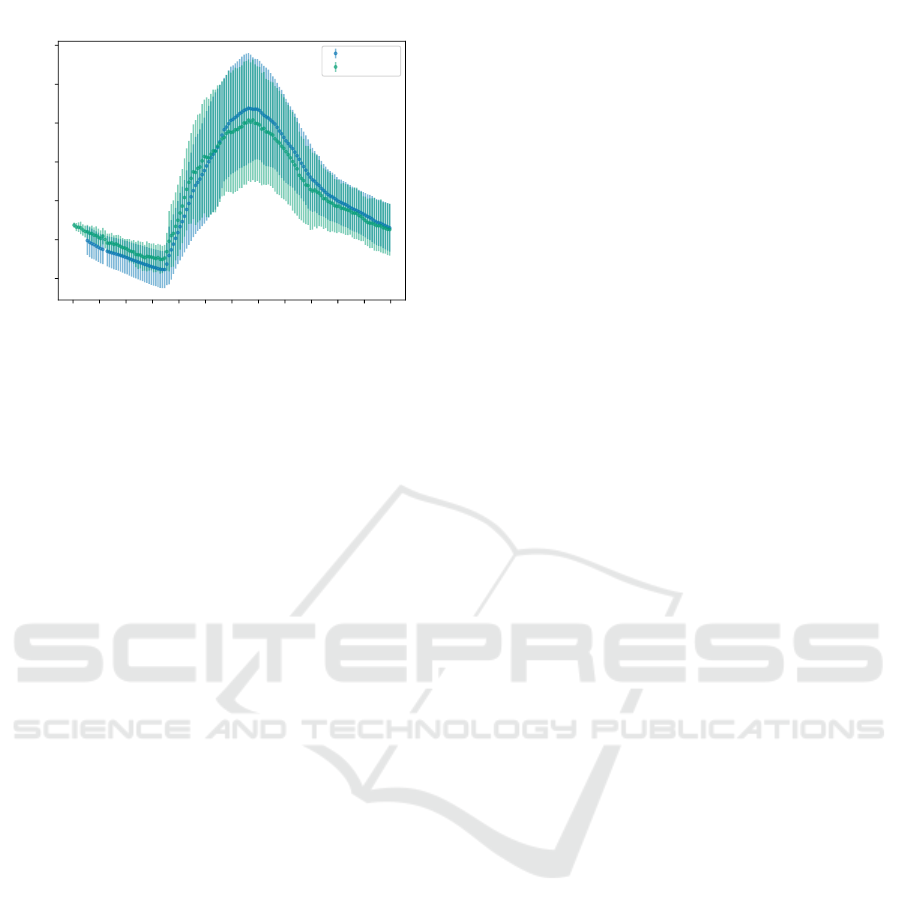
0 2 4 6 8 10 12 14 16 18 20 22 24
Time (h)
18
20
22
24
26
28
30
Temperature (
◦
C)
Real
Generated
Figure 3: Real and generated streams plotted along with the
deviation measured at each point.
6 CONCLUSIONS
We believe that our context organization model can
be further improved by incorporating stream simila-
rity metrics. While there are several academic works
based on stream prediction and mining (Krempl et al.,
2014), the same can not be said about stream simila-
rity. Further work needs to be done to assert some
ideas expressed on this paper, but our stream charac-
terization model appear to be a viable option.
Meanwhile, the ability to generate streams resem-
bling a given set of learning ones, can be useful in
many situations. For instance, to generate large synt-
hetic datasets where otherwise there is no specific ge-
nerator available. Our general purpose generator has
another big advantage. Improves the repeatability and
validity of IoT/M2M and context-aware platforms.
Currently these platforms use advanced machine lear-
ning algorithms to improve and optimize several pro-
cesses. Having the ability to test them for a long time
in a controlled environment is extremely important.
In future publication we will present an improved
version of our stream characterization model and how
to incorporate it into our context organization model.
ACKNOWLEDGEMENTS
This work was partially supported by European
Regional Development Fund (ERDF) under grant
agreement No. 7678 (Ref. POCI-01-0247-FEDER-
007678) entitled “SGH - SMART GREEN HOME”,
and research grant SFRH/BD/94270/2013.
REFERENCES
Abowd, G. D., Dey, A. K., Brown, P. J., Davies, N., Smith,
M., and Steggles, P. (1999). Towards a better under-
standing of context and context-awareness. In Proc.
of the 1st international symposium on Handheld and
Ubiquitous Computing, pages 304–307.
Antunes, M., Gomes, D., and Aguiar, R. (2015). Semantic
features for context organization. In Future Internet of
Things and Cloud (FiCloud), 2015 3rd International
Conference on, pages 87–92. IEEE.
Antunes, M., Gomes, D., and Aguiar, R. (2016a). Lear-
ning semantic features from web services. In Future
Internet of Things and Cloud (FiCloud), 2016 4rd In-
ternational Conference on. IEEE.
Antunes, M., Gomes, D., and Aguiar, R. L. (2016b). Sca-
lable semantic aware context storage. Future Genera-
tion Computer Systems, 56:675–683.
Bacon, J., Bejan, A., Beresford, A., Evans, D., Gibbens, R.,
and Moody, K. (2011). Using real-time road traffic
data to evaluate congestion. In Jones, C. and Lloyd,
J., editors, Dependable and Historic Computing, vo-
lume 6875 of Lecture Notes in Computer Science, pa-
ges 93–117. Springer Berlin Heidelberg.
Chen, K.-C. and Lien, S.-Y. (2014). Machine-to-machine
communications: Technologies and challenges. Ad
Hoc Networks, 18:3–23.
Dey, A. K. (2001). Understanding and using context. Per-
sonal and Ubiquitous Computing, 5(1):4–7.
Eriksson, J., Girod, L., Hull, B., Newton, R., Madden,
S., and Balakrishnan, H. (2008). The pothole patrol:
Using a mobile sensor network for road surface moni-
toring. In Proceedings of the 6th International Confe-
rence on Mobile Systems,Applications, and Services,
pages 29–39.
Krempl, G.,
ˇ
Zliobaite, I., Brzezi
´
nski, D., H
¨
ullermeier, E.,
Last, M., Lemaire, V., Noack, T., Shaker, A., Sievi, S.,
Spiliopoulou, M., and Stefanowski, J. (2014). Open
challenges for data stream mining research. SIGKDD
Explor. Newsl., 16(1):1–10.
Mohan, P., Padmanabhan, V. N., and Ramjee, R. (2008).
Nericell: rich monitoring of road and traffic conditi-
ons using mobile smartphones. In Proc. of the 6th
ACM conference on Embedded network sensor sys-
tems, pages 323–336.
Perera, C., Zaslavsky, A., Christen, P., and Georgakopoulos,
D. (2014). Context aware computing for the internet
of things: A survey. IEEE Communications Surveys
Tutorials, 16(1):414–454.
Suhr, J. K. and Jung, H. G. (2014). Sensor fusion-based
vacant parking slot detection and tracking. Intelli-
gent Transportation Systems, IEEE Transactions on,
15(1):21–36.
Winograd, T. (2001). Architectures for context. Hum.-
Comput. Interact., 16(2):401–419.
Wortmann, F., Fl
¨
uchter, K., et al. (2015). Internet of
things. Business & Information Systems Engineering,
57(3):221–224.
Extracting Knowledge from Stream Behavioural Patterns
423