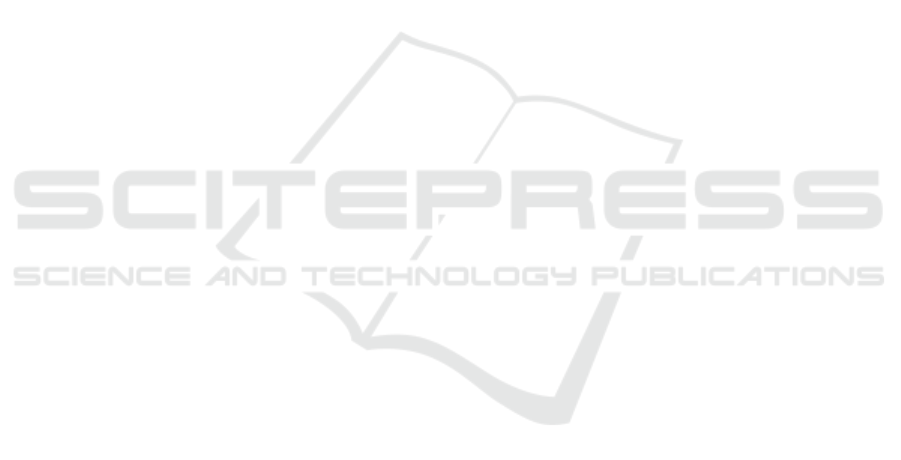
Nutt, D. (2014). The entropic brain: a theory of con-
scious states informed by neuroimaging research with
psychedelic drugs.
Choi, H. and Berger, J. (2013). Waax: Web audio api ex-
tension. In NIME, page 499–502.
Fitz, N. S. and Reiner, P. B. (2013). The challenge of craft-
ing policy for do-it-yourself brain stimulation. Jour-
nal of medical ethics, pages medethics–2013.
Flöel, A., Rösser, N., Michka, O., Knecht, S., and Breit-
enstein, C. (2008). Noninvasive brain stimulation im-
proves language learning. Journal of Cognitive Neu-
roscience, 20(8):1415–1422.
Fregni, F., Boggio, P. S., Nitsche, M., Bermpohl, F., An-
tal, A., Feredoes, E., Marcolin, M. A., Rigonatti, S. P.,
Silva, M. T., Paulus, W., et al. (2005). Anodal tran-
scranial direct current stimulation of prefrontal cortex
enhances working memory. Experimental brain re-
search, 166(1):23–30.
Friston, K. (2010). The free-energy principle: a uni-
fied brain theory? Nature Reviews Neuroscience,
11(2):127–138.
Gladwin, T. E., den Uyl, T. E., Fregni, F. F., and Wiers,
R. W. (2012). Enhancement of selective attention by
tdcs: interaction with interference in a sternberg task.
Neuroscience letters, 512(1):33–37.
Herff, C., Heger, D., Fortmann, O., Hennrich, J., Putze, F.,
and Schultz, T. (2014). Mental workload during n-
back task—quantified in the prefrontal cortex using
fnirs. Frontiers in human neuroscience, 7:935.
Hincks, S. W., Afergan, D., and Jacob, R. J. (2016). Us-
ing fnirs for real-time cognitive workload assessment.
In International Conference on Augmented Cognition,
pages 198–208. Springer.
Hincks, S. W., Bratt, S., Poudel, S., Phoha, V., Dennett,
D. C., Jacob, R. J. K., and Hirshfield, L. M. (2017).
Entropic brain-computer interfacing: Using fnirs and
eeg to measure attentional states in a bayesian frame-
work. In PhyCS.
Horvath, J. C., Forte, J. D., and Carter, O. (2015). Evidence
that transcranial direct current stimulation (tdcs) gen-
erates little-to-no reliable neurophysiologic effect be-
yond mep amplitude modulation in healthy human
subjects: a systematic review. Neuropsychologia,
66:213–236.
Huron, D. B. (2006). Sweet anticipation: Music and the
psychology of expectation. MIT press.
Mann, Y. (2015). Interactive music with tone.js. In Proceed-
ings of the 1st annual Web Audio Conference. Citeseer.
McKendrick, R., Parasuraman, R., and Ayaz, H. (2015).
Wearable functional near infrared spectroscopy (fnirs)
and transcranial direct current stimulation (tdcs):
expanding vistas for neurocognitive augmentation.
Frontiers in systems neuroscience, 9:27.
Medeiros, L. F., de Souza, I. C. C., Vidor, L. P., de Souza,
A., Deitos, A., Volz, M. S., Fregni, F., Caumo, W.,
and Torres, I. L. (2012). Neurobiological effects of
transcranial direct current stimulation: a review.
Moëll, B., Kollberg, L., Nasri, B., Lindefors, N., and
Kaldo, V. (2015). Living smart—a randomized con-
trolled trial of a guided online course teaching adults
with adhd or sub-clinical adhd to use smartphones to
structure their everyday life. Internet Interventions,
2(1):24–31.
Nitsche, M. A., Boggio, P. S., Fregni, F., and Pascual-
Leone, A. (2009). Treatment of depression with tran-
scranial direct current stimulation (tdcs): a review. Ex-
perimental neurology, 219(1):14–19.
Nitsche, M. A., Cohen, L. G., Wassermann, E. M., Priori,
A., Lang, N., Antal, A., Paulus, W., Hummel, F., Bog-
gio, P. S., Fregni, F., et al. (2008). Transcranial direct
current stimulation: state of the art 2008. Brain stim-
ulation, 1(3):206–223.
Piper, S. K., Krueger, A., Koch, S. P., Mehnert, J., Haber-
mehl, C., Steinbrink, J., Obrig, H., and Schmitz, C. H.
(2014). A wearable multi-channel fnirs system for
brain imaging in freely moving subjects. Neuroimage,
85:64–71.
Raichle, M. E., MacLeod, A. M., Snyder, A. Z., Powers,
W. J., Gusnard, D. A., and Shulman, G. L. (2001). A
default mode of brain function. Proceedings of the
National Academy of Sciences, 98(2):676–682.
Rogers, C. (2012). Web audio api. s Draft [online specifi-
cation], Version, 1.
Shih, Y.-N., Huang, R.-H., and Chiang, H.-Y. (2012).
Background music: Effects on attention performance.
Work, 42(4):573–578.
Shiozawa, P., Fregni, F., Benseñor, I. M., Lotufo, P. A.,
Berlim, M. T., Daskalakis, J. Z., Cordeiro, Q., and
Brunoni, A. R. (2014). Transcranial direct current
stimulation for major depression: an updated system-
atic review and meta-analysis. International Journal
of Neuropsychopharmacology, 17(9):1443–1452.
Solovey, E., Schermerhorn, P., Scheutz, M., Sassaroli, A.,
Fantini, S., and Jacob, R. (2012). Brainput: enhanc-
ing interactive systems with streaming fnirs brain in-
put. In Proceedings of the SIGCHI conference on
Human Factors in Computing Systems, pages 2193–
2202. ACM.
Treacy Solovey, E., Afergan, D., Peck, E. M., Hincks, S. W.,
and Jacob, R. J. (2015). Designing implicit interfaces
for physiological computing: guidelines and lessons
learned using fnirs. ACM Transactions on Computer-
Human Interaction (TOCHI), 21(6):35.
Yuksel, B. F., Oleson, K. B., Harrison, L., Peck, E. M.,
Afergan, D., Chang, R., and Jacob, R. J. (2016). Learn
piano with bach: An adaptive learning interface that
adjusts task difficulty based on brain state. In Proceed-
ings of the 2016 CHI Conference on Human Factors
in Computing Systems, pages 5372–5384. ACM.
Zaehle, T., Sandmann, P., Thorne, J. D., Jäncke, L., and
Herrmann, C. S. (2011). Transcranial direct current
stimulation of the prefrontal cortex modulates work-
ing memory performance: combined behavioural and
electrophysiological evidence. BMC neuroscience,
12(1):1.
Zander, T. O., Brönstrup, J., Lorenz, R., and Krol, L. R.
(2014). Towards bci-based implicit control in human–
computer interaction. In Advances in Physiological
Computing, pages 67–90. Springer.
PhyCS 2017 - 4th International Conference on Physiological Computing Systems
64