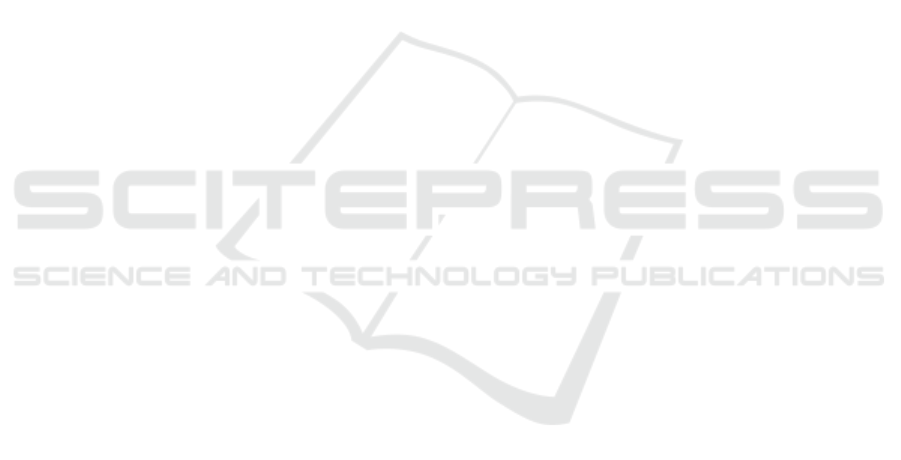
locations exposure), both locations should be consid-
ered.
All these previously mentioned particularities and
limitations of the present work, namely using rough
temporal scales and not considering a personalized
approach, might explain the lower sensitivity values
compared to the overall accuracy. Still, regarding the
interest for asthma self-management tools, the classi-
fication obtained can be used as geographical depen-
dent risk indicator, in spite of the above listed limita-
tions.
4 CONCLUSIONS
The classifier developed in this work allowed to fore-
cast asthma related admissions with good accuracy
levels. The reduced rate of false positive is important
if it is to be included in information and communi-
cation technology tools for patient self-management.
It can be used as a risk warning tool, to be combined
with individual monitoring factors. Despite all the en-
vironmental variables have been processed and ana-
lyzed in a GIS software, in the future a deeper analysis
using a GIS approach and considering other factors,
not considered in this work will improve the informa-
tion on the spatial distribution of asthma hospitaliza-
tions and their relationship with the environment.
ACKNOWLEDGEMENTS
This article was supported by the Project NORTE-
01-0145-FEDER-000016 (NanoSTIMA), financed
by the North Portugal Regional Operational Pro-
gramme (NORTE 2020), under the PORTUGAL
2020 Partnership Agreement, and through the
European Regional Development Fund (ERDF).
Hern
ˆ
ani Gonc¸alves is financed by a post-doctoral
grant (SFRH/BPD/69671/2010) from the Fundac¸
˜
ao
para a Ci
ˆ
encia e a Tecnologia (FCT), Portugal.
The MATLAB licenses used in this work were
supported by Portuguese funds through CMUP
UID/MAT/00144/2013, funded by the Portuguese
Foundation for Science and Technology (FCT -
Fundac¸
˜
ao para a Ci
ˆ
encia e a Tecnologia). The authors
wish to thank the Portuguese Ministry’s of Health Au-
thority for Health Services (Administrac¸
˜
ao Central do
Sistema de Sa
´
ude, ACSS) for providing access to na-
tional hospital admissions data and to Diogo Ayres
Sampaio by the initial preprocessing of the data.
REFERENCES
Akinbami, L. J., Lynch, C. D., Parker, J. D., and Woodruff,
T. J. (2010). The association between childhood
asthma prevalence and monitored air pollutants in
metropolitan areas, United States, 2001-2004. Envi-
ronmental Research, 110(3):294 – 301.
Ayres-Sampaio, D., Teodoro, A. C., Sillero, N., Santos, C.,
Fonseca, J., and Freitas, A. (2014). An investigation
of the environmental determinants of asthma hospital-
izations: An applied spatial approach. Applied Geog-
raphy, 47:10 – 19.
Bashshur, R. L., Shannon, G. W., Smith, B. R., Alverson,
D. C., Antoniotti, N., Barsan, W. G., Bashshur, N.,
Brown, E. M., Coye, M. J., Doarn, C. R., Ferguson,
S., Grigsby, J., Krupinski, E. A., Kvedar, J. C., Link-
ous, J., Merrell, R. C., Nesbitt, T., Poropatich, R.,
Rheuban, K. S., Sanders, J. H., Watson, A. R., We-
instein, R. S., and Yellowlees, P. (2014). The Em-
pirical Foundations of Telemedicine Interventions for
Chronic Disease Management. Telemedicine and e-
Health, 20(9):769–800.
Cruz-Correia, R., Fonseca, J., Amaro, M. J., Lima, L.,
Ara
´
ujo, L., Delgado, L., Grac¸a Castel-Branco, M.,
Costa-Pereira, A., Jo
˜
ao, R., and Correia, C. (2007).
Web-based or paper-based self- management tools for
Asthma patients’ opinions and quality of data in a ran-
domized crossover study. Studies in health technology
and informatics.
Delamater, P. L., Finley, A. O., and Banerjee, S. (2012). An
analysis of asthma hospitalizations, air pollution, and
weather conditions in los angeles county, california.
Science of The Total Environment, 425:110 – 118.
Farr, T. G., Rosen, P. A., Caro, E., Crippen, R., Duren, R.,
Hensley, S., Kobrick, M., Paller, M., Rodriguez, E.,
Roth, L., Seal, D., Shaffer, S., Shimada, J., Umland,
J., Werner, M., Oskin, M., Burbank, D., and Alsdorf,
D. (2007). The Shuttle Radar Topography Mission.
Reviews of Geophysics, 45(2):RG2004.
Ferreira-Magalh
˜
aes, M., Pereira, A. M., Sa-Sousa, A.,
Morais-Almeida, M., Azevedo, I., Azevedo, L. F., and
Fonseca, J. A. (2015). Asthma control in children is
associated with nasal symptoms, obesity, and health
insurance: a nationwide survey. Pediatric Allergy and
Immunology, 26(5):466–473.
Fonseca, J. A., Costa-Pereira, A., Delgado, L., Fernandes,
L., and Castel-Branco, M. G. (2006). Asthma patients
are willing to use mobile and web technologies to sup-
port self-management. Allergy, 61(3):389–390.
GINA (2016). Global strategy for asthma manage-
ment and prevention. Technical report, Global
Initiative for Asthma. http://ginasthma.org/2016-
gina-report-global-strategy-for-asthma-management-
and-prevention/.
Marcano Belisario, J. S., Huckvale, K., Greenfield, G., Car,
J., and Gunn, L. H. (2013). Smartphone and tablet
self management apps for asthma. In Marcano Belis-
ario, J. S., editor, Cochrane Database of Systematic
Reviews, number 11, page CD010013. John Wiley &
Sons, Ltd, Chichester, UK.
Moustris, K. P., Douros, K., Nastos, P. T., Larissi, I. K.,
Anthracopoulos, M. B., Paliatsos, A. G., and Priftis,
Forecasting Asthma Hospital Admissions from Remotely Sensed Environmental Data
129