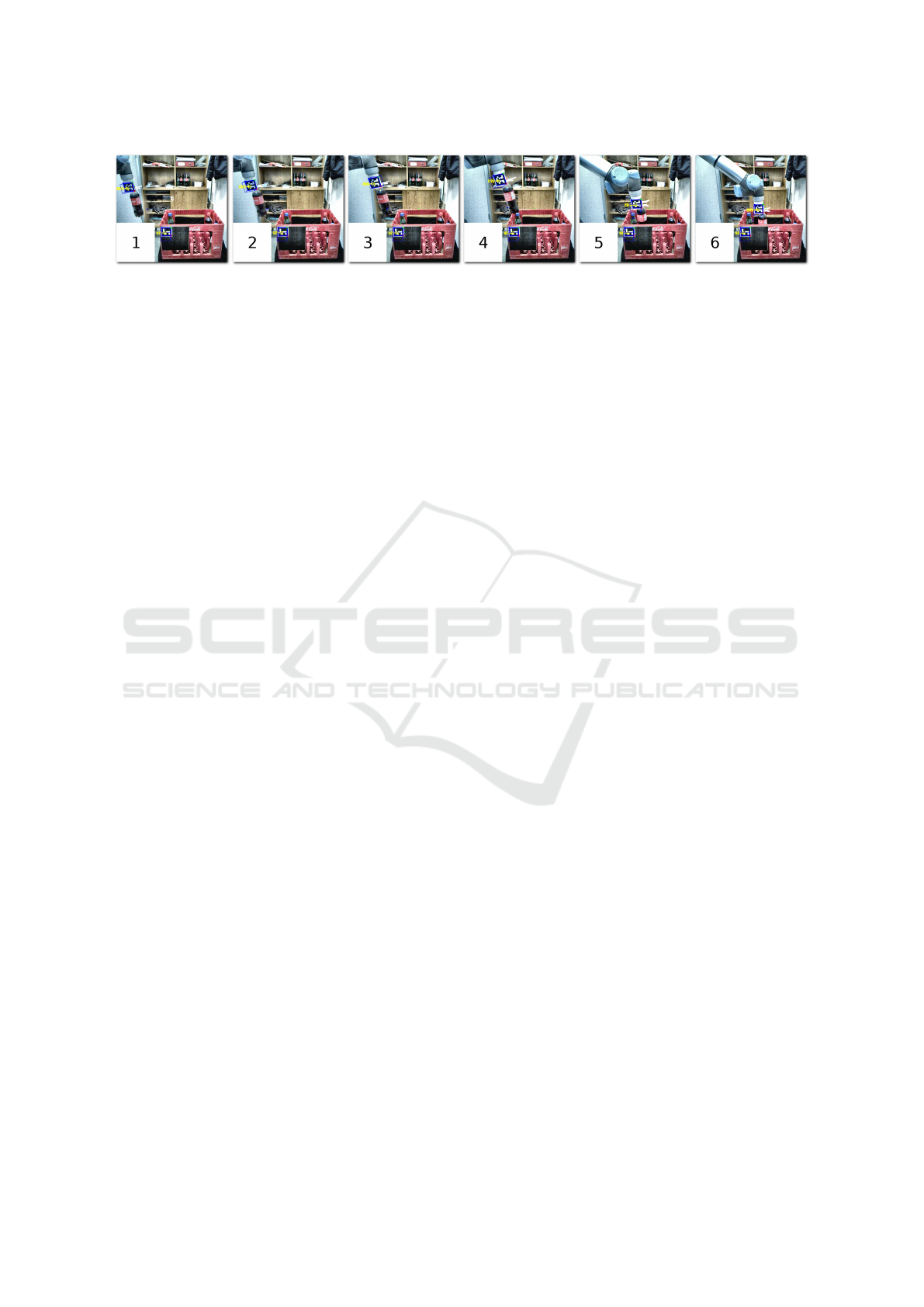
Figure 8: The full action sequence: visual servoing (1-4) and Peg-in-Hole (5-6).
REFERENCES
Berio, D., Calinon, S., and Leymarie, F. F. (2016). Learn-
ing dynamic graffiti strokes with a compliant robot.
In IEEE/RSJ Int. Conf. on Intelligent Robots and Sys-
tems, pages 3981–3986.
Botev, Z. I., Grotowski, J. F., Kroese, D. P., et al. (2010).
Kernel density estimation via diffusion. The Annals of
Statistics, 38(5):2916–2957.
Cherubini, A., Chaumette, F., and Oriolo, G. (2008). A
position-based visual servoing scheme for following
paths with nonholonomic mobile robots. In IEEE/RSJ
Int. Conf. on Intelligent Robots and Systems, pages
1648–1654.
Detry, R., Kraft, D., Kroemer, O., Bodenhagen, L., Peters,
J., Kr
¨
uger, N., and Piater, J. (2011). Learning grasp
affordance densities. Paladyn Journal of Behavioral
Robotics, 2(1).
Dietrich, F., Buchholz, D., Wobbe, F., Sowinski, F., Raatz,
A., Schumacher, W., and Wahl, F. M. (2010). On con-
tact models for assembly tasks: Experimental investi-
gation beyond the peg-in-hole problem on the exam-
ple of force-torque maps. In IEEE/RSJ Int. Conf. on
Intelligent Robots and Systems, pages 2313–2318.
Gams, A. and Petri
ˇ
c, T. (2016). On-line modifications of
robotic trajectories: Learning, coaching and force vs.
position feedback. In Int. Conf. on Robotics in Alpe-
Adria Danube Region, pages 20–28. Springer.
Gams, A., Petric, T., Nemec, B., and Ude, A. (2014). Learn-
ing and adaptation of periodic motion primitives based
on force feedback and human coaching interaction.
In IEEE-RAS Int. Conf. on Humanoid Robots, pages
166–171.
Garrido-Jurado, S., noz Salinas, R. M., Madrid-Cuevas, F.,
and Mar
´
ın-Jim
´
enez, M. (2014). Automatic generation
and detection of highly reliable fiducial markers under
occlusion. Pattern Recognition, 47(6):2280 – 2292.
Graf, B., Reiser, U., H
¨
agele, M., Mauz, K., and Klein, P.
(2009). Robotic home assistant care-o-bot 3 - product
vision and innovation platform. In IEEE Workshop on
Advanced Robotics and its Social Impacts.
Gratal, X., Romero, J., and Kragic, D. (2011). Virtual visual
servoing for real-time robot pose estimation. IFAC
Proceedings Volumes, 44(1):9017–9022.
Jørgensen, J. A. and Petersen, H. G. (2008). Usage of sim-
ulations to plan stable grasping of unknown objects
with a 3-fingered Schunk hand. In IEEE Int. Conf.
on Intelligent Robots and Systems, Workshop - Robot
simulators: available software, scientific applications
and future trends.
Kashiri, N., Laffranchi, M., Tsagarakis, N. G., Margan,
A., and Caldwell, D. G. (2014). Physical interac-
tion detection and control of compliant manipulators
equipped with friction clutches. In IEEE Int. Conf. on
Robotics and Automation, pages 1066–1071.
Kyrki, V., Kragic, D., and Christensen, H. I. (2004).
New shortest-path approaches to visual servoing. In
IEEE/RSJ Int. Conf. on Intelligent Robots and Sys-
tems, pages 349–354.
Lin, L. L., Yang, Y., Song, Y. T., Nemec, B., Ude, A., Rytz,
J. A., Buch, A. G., Kr
¨
uger, N., and Savarimuthu, T. R.
(2014). Peg-in-hole assembly under uncertain pose
estimation. In Proc. of the 11th World Congress on In-
telligent Control and Automation, pages 2842–2847.
Mainprice, J., Hayne, R., and Berenson, D. (2015). Predict-
ing human reaching motion in collaborative tasks us-
ing inverse optimal control and iterative re-planning.
In IEEE Int. Conf. on Robotics and Automation, pages
885–892.
Schwarz, M., Schulz, H., and Behnke, S. (2015). RGB-
D object recognition and pose estimation based on
pre-trained convolutional neural network features. In
IEEE Int. Conf. on Robotics and Automation, pages
1329–1335.
Sørensen, L. C., Buch, J. P., Petersen, H. G., and Kraft, D.
(2016). Online action learning using kernel density
estimation for quick discovery of good parameters for
peg-in-hole insertion. In 13th Int. Conf. on Informatics
in Control, Automation and Robotics.
Zuiderveld, K. (1994). Contrast limited adaptive histogram
equalization. In Graphics gems IV, pages 474–485.
Academic Press Professional, Inc.
Applying Peg-in-Hole Actions with a Service Robot
231