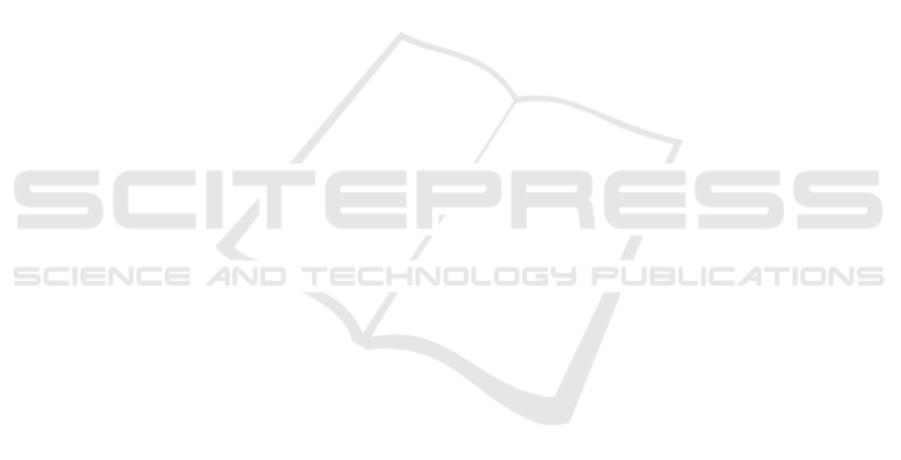
The inventory in process that must be maintained
at the maximum is 32 pairs per hour, which indicates
that if a greater value is conserved within the day, the
company begins to generate unnecessary
accumulation of the same one.
It is concluded that in order to maintain a
production rate that satisfies the maximum capacity
of the plant, every hour an inventory in process
equivalent to 64% of the total number of units
scheduled for production must be maintained.
For a manufacturing system to be optimal, the TH
production rate should not change over time but
remain constant, this is a clear indicator that resources
are coming to the system on time and supplying it is
efficient, where prevents waste generation.
The results of the model employed identify
companies with an efficient management of their
processes, and others that require support to analyze
and respond with data and facts scientifically proven
to eliminate the root causes of their problems.
ACKNOWLEDGEMENTS
The authors thank the National Footwear Chamber of
Ecuador and the Technical University of Ambato for
the support provided during the execution of this
work within the framework of the research project
called “Operational optimization based on a lean
dynamic system of alert of failures in the production
processes for the footwear industry”.
REFERENCES
Anagnostou, A. & Taylor, S., 2017. A distributed
simulation methodological framework for OR/MS
applications. Simulation Modelling Practice and
Theory, Volume 70, p. 101–119.
Cevikcan, E. & Kilic, H. S., 2016. Tempo rating approach
using fuzzy rule based system and westinghouse
method for the assessment of normal time..
International Journal of Industrial Engineering, 23(1),
pp. 49-67.
Cocuzza, S., Fornasiero, R. & Debei, S., 2012. Novel
automated production system for the footwear industry.
In: Heidelberg, ed. IFIP Advances in Information and
Communication Technology (IFIPAICT). Berlin:
Springer, pp. 542-549.
Glock, . C. H., Battini, D., Grosse, E. H. & Alessandro, P.,
2016. Human energy expenditure in order picking
storage assignment: A bi-objective method. Computers
& Industrial Engineerin, Volume 94, p. 147–157.
Goldratt, E. & Cox, J., 2014. The goal: a process of ongoing
improvement. 4th ed. Great Barrington: North River
Press.
Grimard, C., Marvel, J. & Standridge, C. R., 2005.
Validation of the re-design of a manufacturing work
cell using simulation. In Proceedings of the 37th
conference on Winter simulation , pp. 1386-1391.
Günay, N. S., Vayvay, Ö. & Şimşit, Z., 2014. Theory of
Constraints: A Literature Review. Procedia-Social and
Behavioral Sciences, Volume 150, pp. 930-936.
Hopp, W. J. & Spearman, M. L., 2011. Factory Physics.
Third ed. Long Grove: Waveland Press.
Johar, F., Nordin , S. Z. & Potts, C., 2016. Coordination of
production scheduling and vehicle routing problem
with due dates. In advances in industrial and applied
mathematics, 1750(1), p. 030035.
Krajewski , L., Malhotra, M. & Ritzman, . L., 2016.
Operations Management: Processes and Supply
Chains. 11 ed. Edinburgh: Pearson education.
Li, B., 2010. Research on the production scheduling
management system based on SOA. In: Lecture Notes
in Computer Science. Berlin: Springer, pp. 286-294.
Li, N., Zhang, L., Zhang, M. & Zheng, L., 2005. Applied
factory physics study on semiconductor assembly and
test manufacturing. In ISSM 2005, IEEE International
Symposium on Semiconductor Manufacturing, pp. 307-
310.
Mahapatra, S. & Amit Sahu, 2006. Application of Theory
of Constraints on Scheduling of Drum-buffer-rope
System. IOSR Journal of Mechanical and Civil
Engineering (IOSR-JMCE), pp. 15-20.
Marcelo, M. T. et al., 2016. Process improvement and
utilization of machines in the production area of a shoe
manufacturing company. In: Industrial Engineering
and Engineering Management (IEEM). Bali: IEEE.
Reyes Vasquez, J. P., Aldas Salazar, D. S., Morales
Perrazo, L. A. & García Carrillo , M. G., 2016.
Evaluation of the capacity for assembly in the footwear.
Ingeniería Industrial, 37(1), pp. 14-23 .
Reyes, J., Alvarez, K. & Vasquez, R., 2016. Dynamic
buffer management for raw material supply in the
footwear industry. Journal of Industrial and Intelligent
Information, 4(1), pp. 1-8.
Standridge, C. R., 2004. How factory physics helps
simulation. pp. 1103-1108.
Zangiacomi, A., Zhijian, L., Sacco, M. & Boër, C., 2007.
Process planning and scheduling for mass customised
shoe manufacturing. International Journal of Computer
Integrated Manufacturing, 17(7), pp. 613-621.
Zhang, J. & Wang, X., 2016. Multi-agent-based
hierarchical collaborative scheduling in re-entrant
manufacturing systems. International Journal of
Production Research, pp. 1-17.
SIMULTECH 2017 - 7th International Conference on Simulation and Modeling Methodologies, Technologies and Applications
254