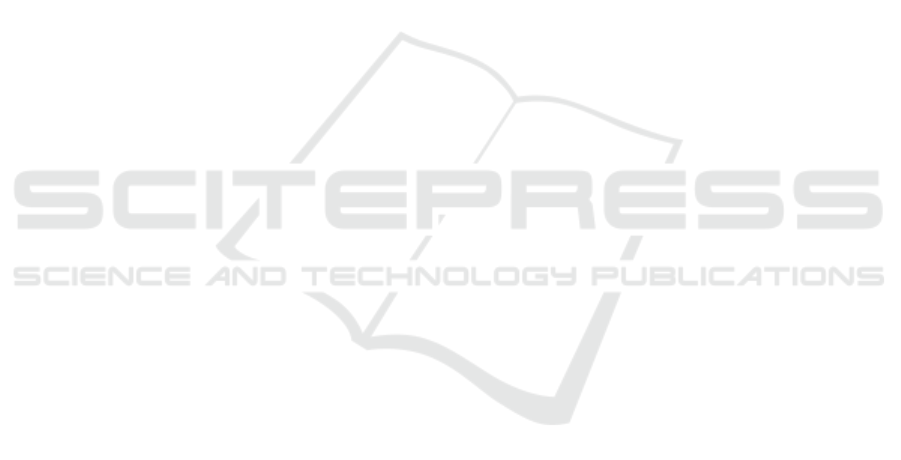
ponent types with numerical values. This affects the
storage of values in the data warehouse as well as the
algorithm that compares values. Another direction
is to strive for a joint dictionary bridging language-
specific component terms. This accelerates the inte-
gration process especially in companies with interna-
tional respect.
ACKNOWLEDGEMENTS
This work is funded by the Austrian Research Promo-
tion Agency (FFG) under grant 852658 (CODA). We
thank Walter Obenaus (Siemens Rail Automation) for
supplying us with test data.
REFERENCES
Bilenko, M. and Mooney, R. J. (2003). Adaptive duplicate
detection using learnable string similarity measures.
In Proceedings of the ninth ACM SIGKDD, pages 39–
48. ACM.
Bleiholder, J. and Naumann, F. (2009). Data fusion. ACM
Computing Surveys (CSUR), 41(1):1.
Cohen, W. W., Ravikumar, P., and Fienberg, S. E. (2003).
A comparison of string distance metrics for name-
matching tasks. In Proceedings of IJCAI-03, August
9-10, 2003, Acapulco, Mexico, pages 73–78.
Dai, W., Wardlaw, I., Cui, Y., Mehdi, K., Li, Y., and Long, J.
(2016). Data profiling technology of data governance
regarding big data: Review and rethinking. In In-
formation Technology: New Generations, pages 439–
450. Springer.
Dallachiesa, M., Ebaid, A., Eldawy, A., Elmagarmid, A.,
Ilyas, I. F., Ouzzani, M., and Tang, N. (2013). Nadeef:
a commodity data cleaning system. In Proceedings of
the 2013 ACM SIGMOD, pages 541–552. ACM.
Dasu, T. and Johnson, T. (2003). Exploratory data mining
and data cleaning: An overview. Exploratory data
mining and data cleaning, pages 1–16.
Fan, W. and Geerts, F. (2012). Foundations of data quality
management. Synthesis Lectures on Data Manage-
ment, 4(5):1–217.
Fan, W., Li, J., Ma, S., Tang, N., and Yu, W. (2010). To-
wards certain fixes with editing rules and master data.
Proceedings of the VLDB Endowment, 3(1-2):173–
184.
Gill, R. and Singh, J. (2014). A review of contemporary
data quality issues in data warehouse etl environment.
Journal on Today’s Ideas - Tomorrow’s Technologies.
Gottesheim, W., Mitsch, S., Retschitzegger, W., Schwinger,
W., and Baumgartner, N. (2011). Semgentowards a
semantic data generator for benchmarking duplicate
detectors. In DASFAA, pages 490–501. Springer.
Hellerstein, J. M. (2008). Quantitative data cleaning for
large databases. United Nations Economic Commis-
sion for Europe (UNECE).
Khayyat, Z., Ilyas, I. F., Jindal, A., Madden, S., Ouzzani,
M., Papotti, P., Quian
´
e-Ruiz, J.-A., Tang, N., and Yin,
S. (2015). Bigdansing: A system for big data cleans-
ing. In Proceedings of the 2015 ACM SIGMOD, pages
1215–1230. ACM.
Krishnan, S., Haas, D., Franklin, M. J., and Wu, E. (2016).
Towards reliable interactive data cleaning: a user sur-
vey and recommendations. In HILDA@ SIGMOD,
page 9.
Langer, P., Wimmer, M., Gray, J., Kappel, G., and Valle-
cillo, A. (2012). Language-specific model version-
ing based on signifiers. Journal of Object Technology,
11(3):4–1.
Leser, U. and Naumann, F. (2007). Informationsintegration
- Architekturen und Methoden zur Integration verteil-
ter und heterogener Datenquellen. dpunkt.verlag.
Liu, H., Kumar, T. A., and Thomas, J. P. (2015). Clean-
ing framework for big data-object identification and
linkage. In 2015 IEEE International Congress on Big
Data, pages 215–221. IEEE.
M
¨
uller, H. and Freytag, J.-C. (2005). Problems, methods,
and challenges in comprehensive data cleansing. Pro-
fessoren des Inst. f
¨
ur Informatik.
Naumann, F. (2014). Data profiling revisited. ACM SIG-
MOD Record, 42(4):40–49.
Papadakis, G., Alexiou, G., Papastefanatos, G., and
Koutrika, G. (2015). Schema-agnostic vs schema-
based configurations for blocking methods on homo-
geneous data. Proceedings of the VLDB Endowment,
9(4):312–323.
Rahm, E. and Do, H. H. (2000). Data cleaning: Prob-
lems and current approaches. IEEE Data Eng. Bull.,
23(4):3–13.
Runeson, P. and H
¨
ost, M. (2009). Guidelines for conduct-
ing and reporting case study research in software engi-
neering. Empirical software engineering, 14(2):131.
Salton, G. and Harman, D. (2003). Information retrieval.
John Wiley and Sons Ltd.
Sharma, S. and Jain, R. (2014). Modeling etl process for
data warehouse: an exploratory study. In In ACCT,
2014 Fourth International Conference on, pages 271–
276. IEEE.
Volkovs, M., Chiang, F., Szlichta, J., and Miller, R. J.
(2014). Continuous data cleaning. In 2014 IEEE 30th
ICDE, pages 244–255. IEEE.
Wang, J., Kraska, T., Franklin, M. J., and Feng, J. (2012).
Crowder: Crowdsourcing entity resolution. Proceed-
ings of the VLDB Endowment, 5(11):1483–1494.
Wimmer, M. and Langer, P. (2013). A benchmark for
model matching systems: The heterogeneous meta-
model case. Softwaretechnik-Trends, 33(2).
Using Signifiers for Data Integration in Rail Automation
179