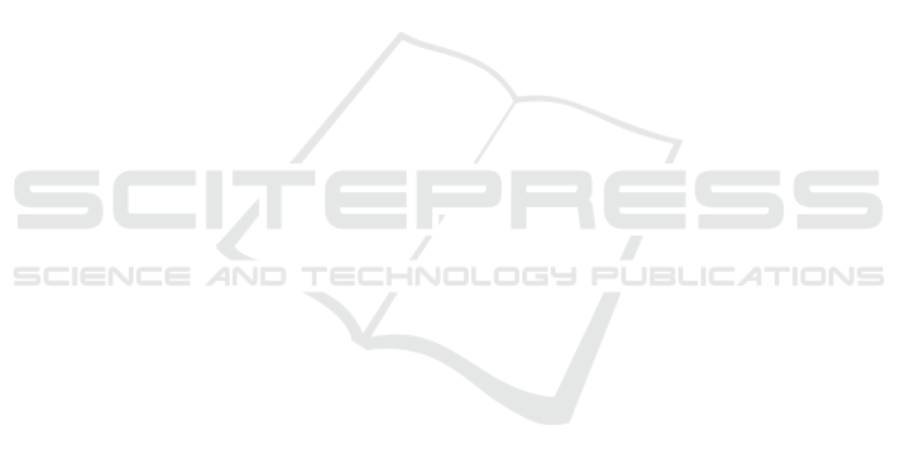
Similar to the level 1 privacy (i.e. P
1
and P
2
), both
P
3
, P
4
6= 0. Thus the final privacy levels can be quan-
tified as -
P
3
= P
3
+ P
4
= H(X
P
1
|X
K
◦
, Z
◦
) + H(X
P
2
|X
K
◦
, Z
◦
)
(3)
On comparing the values of P
1
, P
2
and P
3
from
Eqn. 1, Eqn. 2 and Eqn. 3 respectively, we can repre-
sent a ordinal relationship among them as -
P
1
<P
2
<P
3
This relationship follows from the facts that P
1
=
0 and H(X
P
2
|X
K
◦
, Z
◦
) is a positive quantity. The max-
imum amount of privacy gets preserved when we im-
plement appropriate sanitization procedures on both
the databases, whereas the total privacy attains the
lower bound of 0 (i.e. no privacy is preserved) when
none of the databases are sanitized. Hence this re-
lation also vindicates the notion that a sanitization
mechanism facilitates in preserving the user’s privacy.
5 CONCLUSION AND FUTURE
SCOPES
In our work, we have attempted to formally quantify
the achievable privacy levels in the lieu of attribute
based linking attacks involving micro-databases. We
have taken into consideration the various possibilities
in which an adversary may try to learn sensitive in-
formation about an individual and provided the ap-
propriate levels of privacy in each case. Addition-
ally, we have computed the privacy levels for three
distinct cases based on the application of a sanitiza-
tion mechanism on the micro-database. Our findings
theoretically confirm the intuitive notion that a sani-
tization procedure assists in preserving the privacy of
the database respondents.
Privacy breaches in micro-databases primarily oc-
cur due to the existence of multiple attribute based
links among the records of the databases. Although
our work successfully models this setting, the only
constraint of our work is related to the number of
available micro-databases (to the adversary). More
specifically speaking, we have assumed that an ad-
versary is able to perform the linking based attacks
on the basis of two micro-databases. Modifying our
framework for incorporating more than two micro-
databases is a natural extension of our work. Finally
we would like to experimentally evaluate our frame-
work on real-life datasets, which would provide em-
pirical validation of our study.
REFERENCES
Dalenius, T. (1977). Towards a methodology for statistical
disclosure control. Statistik Tidskrift, 15(429-444):2–
1.
Dwork, C. (2006). Differential privacy. In Proceedings
of the 33rd International Conference on Automata,
Languages and Programming - Volume Part II,
ICALP’06, pages 1–12, Berlin, Heidelberg. Springer-
Verlag.
Fung, B. C. M., Wang, K., Chen, R., and Yu, P. S. (2010).
Privacy-preserving data publishing: A survey of re-
cent developments. ACM Comput. Surv., 42(4):14:1–
14:53.
Hansell, S. (2006). Aol removes search data on vast group
of web users. Technical report, New York Times.
Li, N., Li, T., and Venkatasubramanian, S. (2007).
t-closeness: Privacy beyond k-anonymity and l-
diversity. In 2007 IEEE 23rd International Confer-
ence on Data Engineering, pages 106–115.
Machanavajjhala, A., Kifer, D., Gehrke, J., and Venkitasub-
ramaniam, M. (2007). L-diversity: Privacy beyond
k-anonymity. ACM Trans. Knowl. Discov. Data, 1(1).
Malin, B. and Sweeney, L. (2004). How (not) to pro-
tect genomic data privacy in a distributed network:
Using trail re-identification to evaluate and design
anonymity protection systems. J. of Biomedical In-
formatics, 37(3):179–192.
Narayanan, A. and Shmatikov, V. (2006). How to
break anonymity of the netflix prize dataset. CoRR,
abs/cs/0610105.
Rebollo-Monedero, D., Forne, J., and Domingo-Ferrer, J.
(2010). From t-closeness-like privacy to postrandom-
ization via information theory. IEEE Trans. on Knowl.
and Data Eng., 22(11):1623–1636.
Samarati, P. and Sweeney, L. (1998). Generalizing data
to provide anonymity when disclosing information
(abstract). In Proceedings of the Seventeenth ACM
SIGACT-SIGMOD-SIGART Symposium on Principles
of Database Systems, PODS ’98, pages 188–, New
York, NY, USA. ACM.
Sankar, L., Rajagopalan, S. R., and Poor, H. V.
(2013). Utility-privacy tradeoffs in databases: An
information-theoretic approach. IEEE Transactions
on Information Forensics and Security, 8(6):838–852.
Sweeney, L. (1997). Weaving technology and policy to-
gether to maintain confidentiality. The Journal of Law,
Medicine & Ethics, 25(2-3):98–110.
Sweeney, L. (2005). Statement before the privacy and
integrity advisory committee of the department of
homeland security. Technical report, Department of
Homeland Security.
Xiao, X. and Tao, Y. (2006). Anatomy: Simple and effec-
tive privacy preservation. In Proceedings of the 32Nd
International Conference on Very Large Data Bases,
VLDB ’06, pages 139–150. VLDB Endowment.
SECRYPT 2017 - 14th International Conference on Security and Cryptography
436