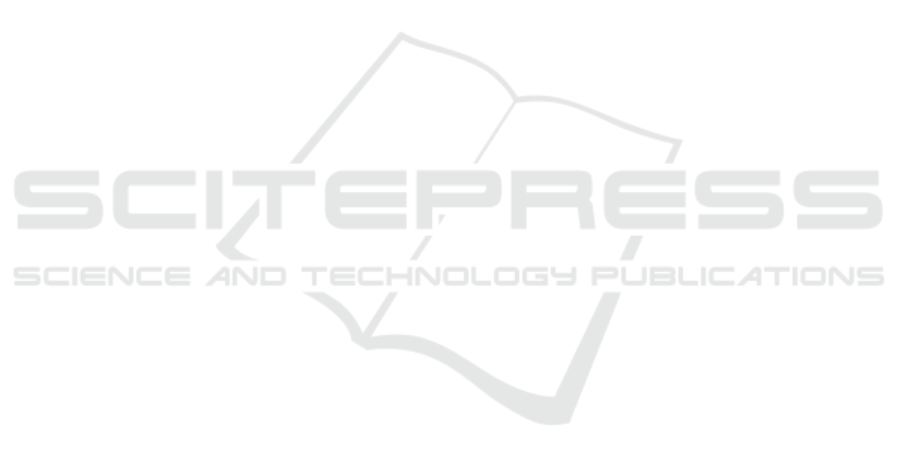
7 CONCLUSION
Data publishing promises significant progress for
emergence and improvement of new services. How-
ever, to mitigate privacy leakages due to poor
anonymization procedures, there is a strong need for
publishers to have a practical and precise metric to
assess the data anonymity level prior to publishing
datasets. In this paper, we propose the Semantic Dis-
crimination Rate which is a new practical metric for
getting fine grained measurement of the anonymity
level of an anonymized dataset. It enables to tackle
the de-anonymization issue from the attacker’s per-
spective, and to precisely compute the attacker’s ca-
pacity according to any existing anonymity attacks.
Illustration of that metric is given over some clas-
sical anonymization techniques (t-closeness and l-
diversity), and proves that t-closeness is not as privacy
protective as it was originally claimed to be as it can
behave worse than l-diversity.
REFERENCES
Abril, D., Navarro-Arribas, G., and Torra, V. (2010). To-
wards semantic microaggregation of categorical data
for confidential documents. In International Confer-
ence on Modeling Decisions for Artificial Intelligence,
pages 266–276. Springer.
Domingo-Ferrer, J. and Torra, V. (2008). A critique of k-
anonymity and some of its enhancements. In Avail-
ability, Reliability and Security, 2008. ARES 08. Third
International Conference on, pages 990–993. IEEE.
Erola, A., Castell
`
a-Roca, J., Navarro-Arribas, G., and
Torra, V. (2010). Semantic microaggregation for the
anonymization of query logs. In International Confer-
ence on Privacy in Statistical Databases, pages 127–
137. Springer.
Hsu, J., Gaboardi, M., Haeberlen, A., Khanna, S., Narayan,
A., Pierce, B. C., and Roth, A. (2014). Differential
privacy: An economic method for choosing epsilon.
In Computer Security Foundations Symposium (CSF),
2014 IEEE 27th, pages 398–410. IEEE.
Lee, J. and Clifton, C. (2011). How much is enough?
choosing ε for differential privacy. In International
Conference on Information Security, pages 325–340.
Springer.
Li, N., Li, T., and Venkatasubramanian, S. (2007).
t-closeness: Privacy beyond k-anonymity and l-
diversity. In Data Engineering, 2007. ICDE 2007.
IEEE 23rd International Conference on, pages 106–
115. IEEE.
Machanavajjhala, A., Kifer, D., Gehrke, J., and Venkita-
subramaniam, M. (2007). l-diversity: Privacy beyond
k-anonymity. ACM Transactions on Knowledge Dis-
covery from Data (TKDD), 1(1):3.
Makhdoumi, A. and Fawaz, N. (2013). Privacy-utility trade-
off under statistical uncertainty. In Communication,
Control, and Computing (Allerton), 2013 51st Annual
Allerton Conference on, pages 1627–1634. IEEE.
Rebollo-Monedero, D., Forne, J., and Domingo-Ferrer, J.
(2010). From t-closeness-like privacy to postrandom-
ization via information theory. Knowledge and Data
Engineering, IEEE Transactions on, 22(11):1623–
1636.
Salamatian, S., Zhang, A., du Pin Calmon, F., Bhamidipati,
S., Fawaz, N., Kveton, B., Oliveira, P., and Taft, N.
(2013). How to hide the elephant-or the donkey-in
the room: Practical privacy against statistical infer-
ence for large data. In GlobalSIP, pages 269–272.
Samarati, P. (2001). Protecting respondents identities in mi-
crodata release. Knowledge and Data Engineering,
IEEE Transactions on, 13(6):1010–1027.
Sankar, L., Rajagopalan, S. R., and Poor, H. V.
(2013). Utility-privacy tradeoffs in databases: An
information-theoretic approach. IEEE Transactions
on Information Forensics and Security, 8(6):838–852.
Shannon, C. E. (2001). A mathematical theory of commu-
nication. ACM SIGMOBILE Mobile Computing and
Communications Review, 5(1):3–55.
Sondeck, L., Laurent, M., and Frey, V. (2017). Discrimina-
tion rate: an attribute-centric metric to measure pri-
vacy. Annals of Telecommunications journal DOI:
10.1007/s12243-017-0581-8.
SECRYPT 2017 - 14th International Conference on Security and Cryptography
294