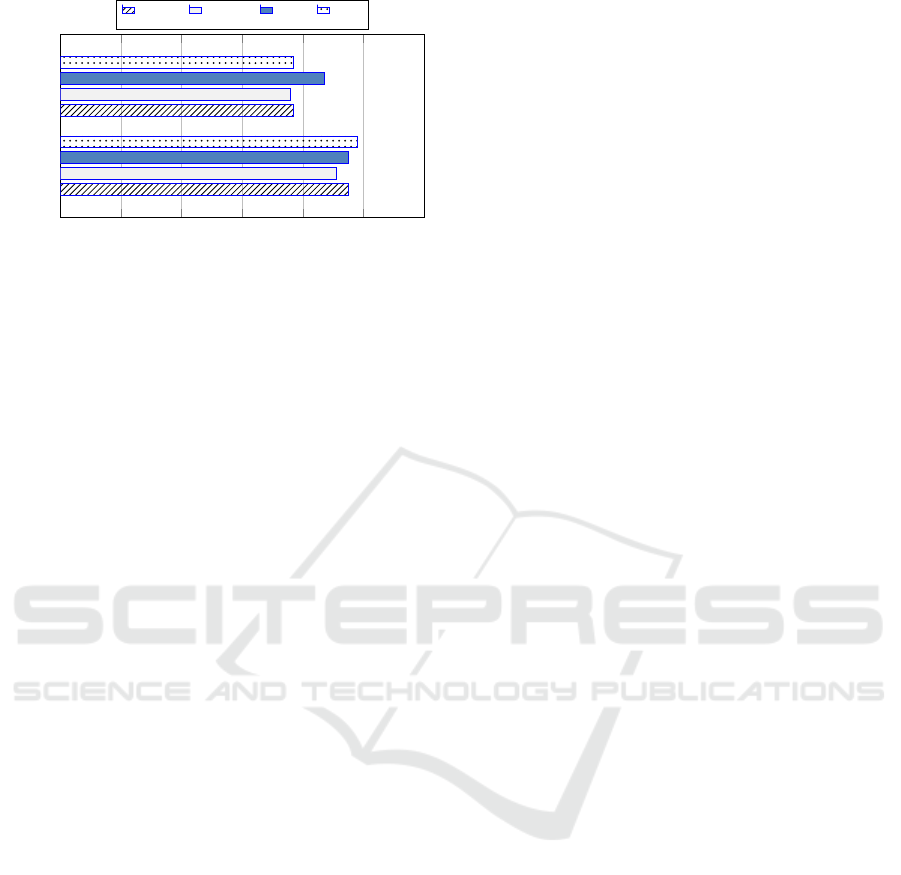
0.20 0.40
0.60
0.80 1.00
RF
FD
0.95
0.77
0.91
0.76
0.95
0.87
0.98
0.77
Value
Approaches
Accuracy
Sensitivity
F-score
AUC
Figure 3: Performance.
wants to introduce a novel frequency-domain-based
model that allows a fraud detection system to operate
proactively. The results obtained are interesting, since
it is necessary to consider that the state-of-the-art
competitor taken into account (i.e., Random Forests),
in addition to using both classes of transactions to
train its model also preprocesses the dataset by us-
ing a balancing technique (i.e., SMOTE). It should be
noted that the credit card context taken into account is
only one of the possible scenarios, since the proposed
approach can be used in any context characterized by
financial electronic transactions.
A possible future work could take into account
the definition of an hybrid approach of fraud detec-
tion that combines the characteristics of the canonical
non-proactive approaches with those of our proactive
approach.
ACKNOWLEDGEMENTS
This research is partially funded by Regione Sardegna
under project Next generation Open Mobile Apps
Development (NOMAD), Pacchetti Integrati di
Agevolazione (PIA) - Industria Artigianato e Servizi -
Annualit
`
a 2013.
REFERENCES
Attenberg, J. and Provost, F. J. (2010). Inactive learn-
ing?: difficulties employing active learning in prac-
tice. SIGKDD Explorations, 12(2):36–41.
Bhattacharyya, S., Jha, S., Tharakunnel, K. K., and West-
land, J. C. (2011). Data mining for credit card fraud:
A comparative study. Decision Support Systems,
50(3):602–613.
Bowyer, K. W., Chawla, N. V., Hall, L. O., and Kegelmeyer,
W. P. (2011). SMOTE: synthetic minority over-
sampling technique. CoRR, abs/1106.1813.
Breiman, L. (2001). Random forests. Machine Learning,
45(1):5–32.
Brown, I. and Mues, C. (2012). An experimental compari-
son of classification algorithms for imbalanced credit
scoring data sets. Expert Syst. Appl., 39(3):3446–
3453.
Chatterjee, A. and Segev, A. (1991). Data manipulation
in heterogeneous databases. ACM SIGMOD Record,
20(4):64–68.
Donmez, P., Carbonell, J. G., and Bennett, P. N. (2007).
Dual strategy active learning. In ECML, volume 4701
of Lecture Notes in Computer Science, pages 116–
127. Springer.
Duhamel, P. and Vetterli, M. (1990). Fast fourier trans-
forms: a tutorial review and a state of the art. Signal
processing, 19(4):259–299.
Faraggi, D. and Reiser, B. (2002). Estimation of the area un-
der the roc curve. Statistics in medicine, 21(20):3093–
3106.
Gao, J., Fan, W., Han, J., and Yu, P. S. (2007). A general
framework for mining concept-drifting data streams
with skewed distributions. In Proceedings of the Sev-
enth SIAM International Conference on Data Min-
ing, April 26-28, 2007, Minneapolis, Minnesota, USA,
pages 3–14. SIAM.
Japkowicz, N. and Stephen, S. (2002). The class imbal-
ance problem: A systematic study. Intell. Data Anal.,
6(5):429–449.
Lessmann, S., Baesens, B., Seow, H., and Thomas, L. C.
(2015). Benchmarking state-of-the-art classifica-
tion algorithms for credit scoring: An update of re-
search. European Journal of Operational Research,
247(1):124–136.
Phua, C., Lee, V. C. S., Smith-Miles, K., and Gayler, R. W.
(2010). A comprehensive survey of data mining-based
fraud detection research. CoRR, abs/1009.6119.
Pozzolo, A. D., Caelen, O., Borgne, Y. L., Waterschoot, S.,
and Bontempi, G. (2014). Learned lessons in credit
card fraud detection from a practitioner perspective.
Expert Syst. Appl., 41(10):4915–4928.
Pozzolo, A. D., Caelen, O., Johnson, R. A., and Bontempi,
G. (2015). Calibrating probability with undersampling
for unbalanced classification. In IEEE Symposium Se-
ries on Computational Intelligence, SSCI 2015, Cape
Town, South Africa, December 7-10, 2015, pages
159–166. IEEE.
Smith, S. W. et al. (1997). The scientist and engineer’s
guide to digital signal processing.
Sorournejad, S., Zojaji, Z., Atani, R. E., and Monadjemi,
A. H. (2016). A survey of credit card fraud detection
techniques: Data and technique oriented perspective.
CoRR, abs/1611.06439.
Vinciotti, V. and Hand, D. J. (2003). Scorecard construc-
tion with unbalanced class sizes. Journal of Iranian
Statistical Society, 2(2):189–205.
Wang, H., Fan, W., Yu, P. S., and Han, J. (2003). Mining
concept-drifting data streams using ensemble classi-
fiers. In Getoor, L., Senator, T. E., Domingos, P. M.,
and Faloutsos, C., editors, Proceedings of the Ninth
ACM SIGKDD International Conference on Knowl-
edge Discovery and Data Mining, Washington, DC,
USA, August 24 - 27, 2003, pages 226–235. ACM.
SECRYPT 2017 - 14th International Conference on Security and Cryptography
342