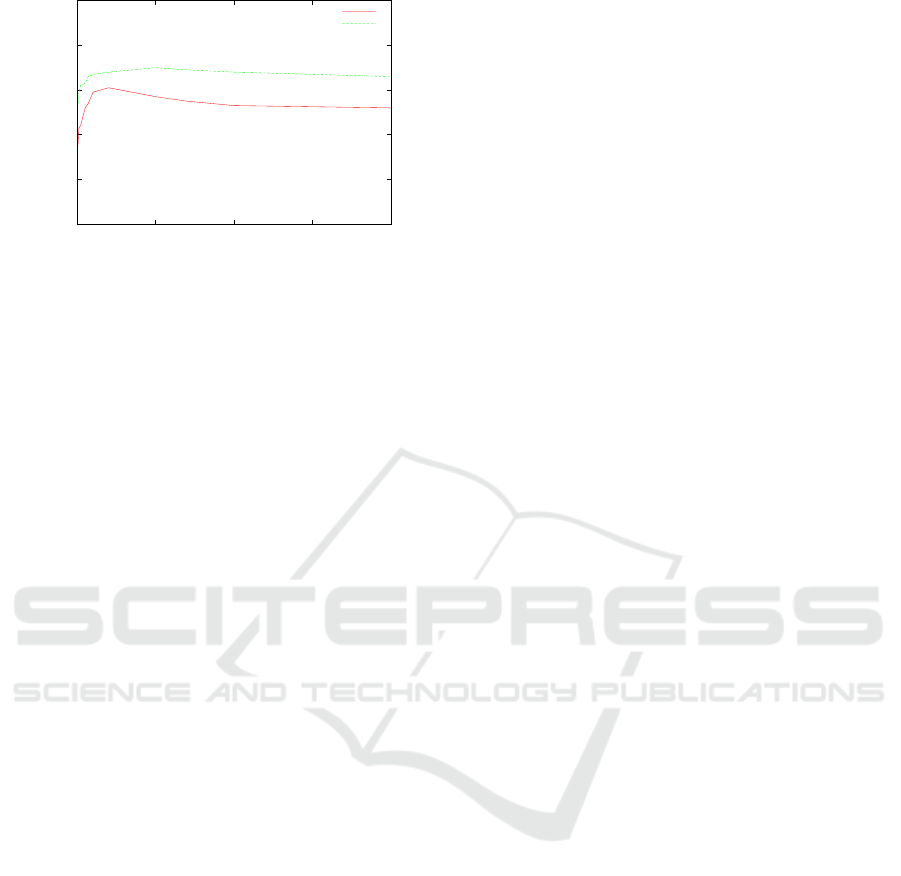
0
0.2
0.4
0.6
0.8
1
0 50000 100000 150000 200000
F-score
Users’ Number
REMSA
RepC
Figure 2: F-score results for RepC and REMSA with vary-
ing the number of users.
In Eq.10, Tw
i
is the number of shared tweets of
the user i and m is the user having the maximum num-
ber of shared tweets.
The simulation results, are shown in Figure 2.
The latter shows that RepC outperforms REMSA
whatever the number of users.
The F-score values for RepC increase as far as the
number of users increases. In fact, by increasing the
number of users, direct and indirect trust values, con-
sidered to compute reputation values, increase leading
to a rise in the authentication success trust rate. Then
reputation values are more accurate.
However, the accuracy of REMSA reaches its best
values for a number of users standing within the range
[5000, 20000], decreasing again when the number of
users exceeds 50000. In fact, considering only neigh-
bours of a user to compute its reputation instead of
the whole network, generates a decrease of precision
and recall values and consequently a decline of the F-
score. This is not the case of RepC which respects the
objectivity property of reputation and keeps produc-
ing correct results with an increasing of the number
of users.
7 CONCLUSION
In this paper, we have proposed a new reputation man-
agement algorithm RepC based on a trust network
generated in previous works. Our algorithm classi-
fies an OSN users into clusters by their trust simi-
larity such that most trustworthy users belong to the
same cluster. The evaluation results, based on the real
social network Twitter, show that our algorithm can
generate high quality results.
As forthcoming works, we plan to simulate a
fuzzy version of RepC and compare between the hard
and fuzzy methods.
REFERENCES
Chen, D., Chang, G., Sun, D., Li, J., Jia, J., and Wang, X.
(2011). Trm-iot: A trust management model based
on fuzzy reputation for internet of things. Computer
Science and Information Systems, (20):1207–1228.
Christianson, B. and Harbison, W. S. (1996). Why isn’t trust
transitive? In Proceedings of the Security Protocols
Workshop, Cambridge, United Kingdom, pages 171–
176.
Davies, D. L. and Bouldin, D. W. (1979). A cluster separa-
tion measure. IEEE Trans. Pattern Anal. Mach. Intell.,
1(2):224–227.
Day, W. H. E. (1992). Complexity theory: An introduction
for practitioners of classification, chapter 6, pages
199–235. World Scientific Publishing.
Dunn, J. C. (1974). Well separated clusters and optimal
fuzzy-partitions. Journal of Cybernetics, 4:95–104.
Hamdi, S., Ganc¸arski, A. L., Bouzeghoub, A., and Yahia,
S. B. (2016). Tison: Trust inference in trust-oriented
social networks. ACM Trans. Inf. Syst., 34(3):17.
Hamdi, S., Ganc¸arski, A. L., Bouzeghoub, A., and
BenYahia, S. (June 25-27, 2012). Iris: A novel method
of direct trust computation for generating trusted so-
cial networks. In Proceedings of the 11th IEEE Inter-
national Conference on Trust, Security and Privacy
in Computing and Communications, TrustCom 2012,
Liverpool, United Kingdom, pages 616–623.
Hartigan, J. A. and Wong, M. A. (1979). A K-means clus-
tering algorithm. Applied Statistics, 28:100–108.
Heaney, M. T. (2014). Multiplex networks and interest
group influence reputation: An exponential random
graph model. Social Networks, 36:66–81.
Lee, J. and Oh, J. C. (2015). A node-centric reputation com-
putation algorithm on online social networks. Appli-
cations of Social Media & Social Network Analysis,
pages 1–22.
Portmann, E. K. (2012). The fora framework: A fuzzy
grassroots ontology for online reputation manage-
ment. PhD thesis, Faculty of Sciences, University of
Fribourg, Switzerland.
Vendramin, L., Campello, R. J. G. B., and Hruschka, E. R.
(2010). Relative clustering validity criteria: A com-
parative overview. Stat. Anal. Data Min., 3(4):209–
235.
Zhang, Y., Chen, H., and Wu, Z. (2006). A social network-
based trust model for the semantic web. In Proceed-
ings of the Third International Conference on Auto-
nomic and Trusted Computing, ATC’06, pages 183–
192, Berlin, Heidelberg. Springer-Verlag.
Reputation Management in Online Social Networks - A New Clustering-based Approach
473