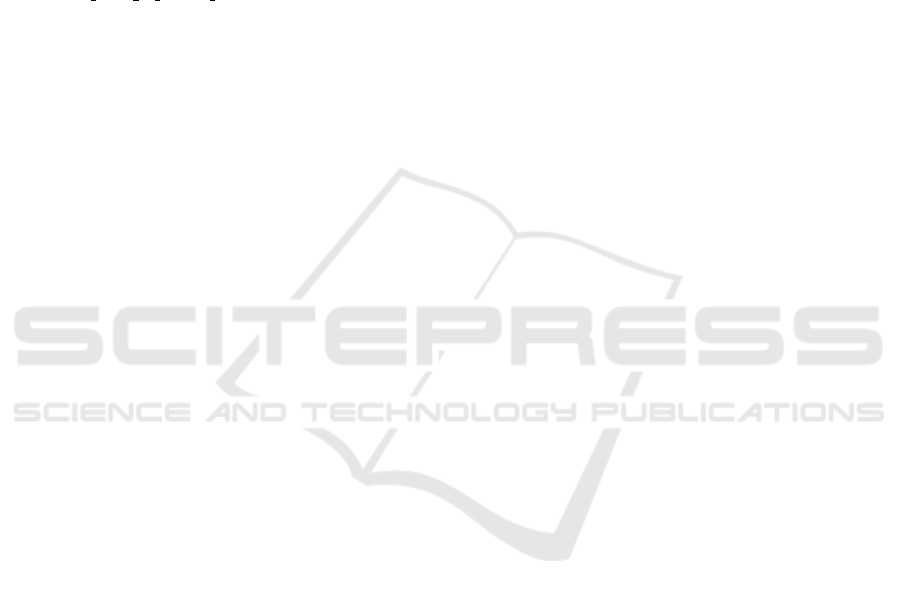
fined based on three different sources: non-ad URLs,
benign-ad URLs, and malicious-ad URLs. The results
show that by using the selected lexical-based features,
online advertisement detection accuracy is about 97%
in certain scenario.
REFERENCES
Adobe (2013). The State of Online Advertising.
https://www.adobe.com/aboutadobe/pressroom/pdfs/
Adobe State of Online Advertising.pdf. (Accessed
date September 2016).
Anderson, D. S., Fleizach, C., Savage, S., and Voelker,
G. M. (2007). Spamscatter: Characterizing internet
scam hosting infrastructure. Usenix Security, pages
1–14.
Andriatsimandefitra, R. and Tong, V. V. T. (2014). Cap-
turing android malware behaviour using system flow
graph. In International Conference on Network and
System Security, pages 534–541. Springer.
Baykan, E., Henzinger, M., and Marian, L. (2009). Purely
url-based topic classification. In Proceedings of the
18th international conference on World Wide Web,
pages 1109–1110. ACM.
Baykan, E., Henzinger, M., Marian, L., and Weber, I.
(2011). A comprehensive study of features and algo-
rithms for url-based topic classification. ACM Trans-
actions on the Web (TWEB), 5(3):15.
Bhagavatula, S., Dunn, C., Kanich, C., Gupta, M., and
Ziebart, B. (2014). Leveraging machine learning to
improve unwanted resource filtering. In Proceedings
of the 2014 Workshop on Artificial Intelligent and Se-
curity Workshop, pages 95–102. ACM.
Chen, C.-M., Guan, D., and Su, Q.-K. (2014). Feature
set identification for detecting suspicious urls using
bayesian classification in social networks. Informa-
tion Sciences, 289:133–147.
Choi, H., Zhu, B. B., and Lee, H. (2011). Detecting ma-
licious web links and identifying their attack types.
WebApps, 11:11–11.
Devi, M. I., Rajaram, D. R., and Selvakuberan, K. (2007).
Machine learning techniques for automated web page
classification using url features. In International Con-
ference on Computational Intelligence and Multime-
dia Applications, 2007, volume 2, pages 116–120.
IEEE.
Hall, M., Frank, E., Holmes, G., Pfahringer, B., Reutemann,
P., and Witten, I. H. (2009). The weka data min-
ing software: an update. ACM SIGKDD explorations
newsletter, 11(1):10–18.
Hern
´
andez, I., Rivero, C. R., and Ruiz (2014). Cala: an un-
supervised url-based web page classification system.
Knowledge-Based Systems, 57:168–180.
Hern
´
andez, I., Rivero, C. R., Ruiz, D., and Corchuelo,
R. (2016). Cala: Classifying links automatically
based on their url. Journal of Systems and Software,
115:130–143.
Jover, R. P., Murynets, I., and Bickford, J. (2015). Detecting
malicious activity on smartphones using sensor mea-
surements. In International Conference on Network
and System Security, pages 475–487. Springer.
Kan, M.-Y. and Thi, H. O. N. (2005). Fast webpage clas-
sification using url features. In Proceedings of the
14th ACM international conference on Information
and knowledge management, pages 325–326. ACM.
Krammer, V. (2008). An effective defense against intrusive
web advertising. In Sixth Annual Conference on Pri-
vacy, Security and Trust, 2008, pages 3–14. IEEE.
Le, A., Markopoulou, A., and Faloutsos, M. (2011).
Phishdef: Url names say it all. In INFOCOM, 2011
Proceedings IEEE, pages 191–195. IEEE.
Li, Z., Alrwais, S., Xie, Y., Yu, F., and Wang, X. (2013).
Finding the linchpins of the dark web: a study on topo-
logically dedicated hosts on malicious web infrastruc-
tures. In Security and Privacy (SP), 2013 IEEE Sym-
posium on, pages 112–126. IEEE.
Li, Z., Zhang, K., Xie, Y., Yu, F., and Wang, X. (2012).
Knowing your enemy: understanding and detecting
malicious web advertising. In Proceedings of the 2012
ACM conference on Computer and Communications
Security, pages 674–686. ACM.
Lin, M.-S., Chiu, C.-Y., Lee, Y.-J., and Pao, H.-K. (2013).
Malicious url filteringa big data application. In big
data, 2013 IEEE international conference on, pages
589–596. IEEE.
Ma, J., Saul, L. K., Savage, S., and Voelker, G. M. (2009).
Beyond blacklists: learning to detect malicious web
sites from suspicious urls. In Proceedings of the 15th
ACM SIGKDD international conference on Knowl-
edge discovery and data mining, pages 1245–1254.
ACM.
Ma, J., Saul, L. K., Savage, S., and Voelker, G. M. (2011).
Learning to detect malicious urls. ACM Transac-
tions on Intelligent Systems and Technology (TIST),
2(3):30.
Mamun, M. S. I., Rathore, M. A., Lashkari, A. H.,
Stakhanova, N., and Ghorbani, A. A. (2016). Detect-
ing malicious urls using lexical analysis. In Interna-
tional Conference on Network and System Security,
pages 467–482. Springer.
Netscape (2007). DMOZ Open Director Project.
http://www.dmoz.org. (Accessed date September
2016).
OpenDNS (2007). PhishTank. http://www.phishtank.com.
(Accessed date September 2016).
Orr, C. R., Chauhan, A., Gupta, M., Frisz, C. J., and Dunn,
C. W. (2012). An approach for identifying javascript-
loaded advertisements through static program analy-
sis. In Proceedings of the 2012 ACM workshop on
Privacy in the electronic society, pages 1–12. ACM.
PageFair (2015). Pagefair and adobe 2015 ad blocking
report. https://pagefair.com/blog/2015/ad-blocking-
report/. (Accessed date September 2016).
Pradeepthi, K. and Kannan, A. (2014). Performance study
of classification techniques for phishing url detection.
In 2014 Sixth International Conference on Advanced
Computing (ICoAC), pages 135–139. IEEE.
A Lightweight Online Advertising Classification System using Lexical-based Features
493