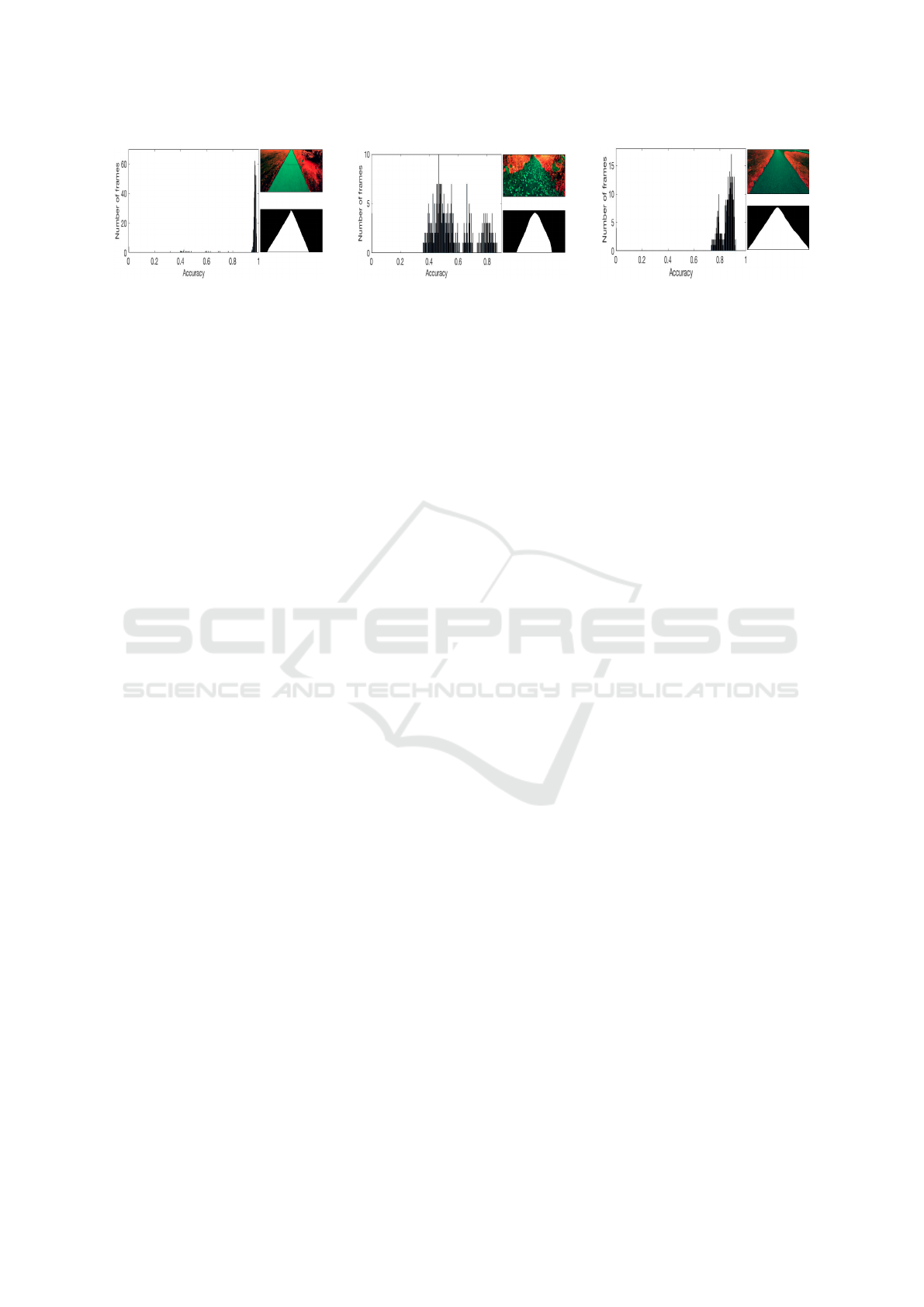
(a) Concrete (b) Dirt (c) Asphalt
Figure 4: The accumulative accuracy histogram charts along side their corresponding frame with highest accuracy and its
hand labeled ground truth mask of (a) concrete, (b) dirt and (c) asphalt datasets respectively. These histograms show the
distribution of the frames based on their computed accuracy in comparison to the ground truth dataset.
Emmanuel Mati-Amorim and Arjun Kaura who hand
labeled the test datasets.
REFERENCES
Bar Hillel, A., Lerner, R., Levi, D., and Raz, G. (2014).
Recent progress in road and lane detection: a survey.
Machine Vision and Applications, pages 1–19.
Buehler, M., Iagnemma, K., and Singh, S. (2007). The 2005
DARPA Grand Challenge: The Great Robot Race,
volume 36. Springer Science & Business Media.
Cremean, L. B. and Murray, R. M. (2006). Model-based es-
timation of off-highway road geometry using single-
axis ladar and inertial sensing. In Proc. ICRA 2006.,
pages 1661–1666.
DARPA (2016). The Defense Advanced Research Projects
Agency (DARPA) website.
DeSouza, G. N. and Kak, A. C. (2002). Vision for mobile
robot navigation: A survey. IEEE PAMI, 24(2):237–
267.
Fischler, M. A. and Bolles, R. C. (1981). Random sample
consensus: a paradigm for model fitting with appli-
cations to image analysis and automated cartography.
Comm. ACM, 24(6):381–395.
Hern
´
andez, J. and Marcotegui, B. (2009). Filtering of ar-
tifacts and pavement segmentation from mobile lidar
data. In ISPRS Workshop Laserscanning 2009.
Inaba, M., Katoh, N., and Imai, H. (1994). Applications
of weighted voronoi diagrams and randomization to
variance-based k-clustering. In Proc. 10th Ann. Symp.
Comp. Geom., pages 332–339.
Kammel, S. and Pitzer, B. (2008). Lidar-based lane marker
detection and mapping. In 2008 IEEE Intell. Vehic.
Symp., pages 1137–1142.
kodak (2016). The Kodak camera website.
Kuan, D., Phipps, G., and Hsueh, A. C. (1988). Au-
tonomous robotic vehicle road following. IEEE PAMI,
10(5):648–658.
Liou, S.-P. and Jain, R. C. (1987). Road following using
vanishing points. Computer Vision, Graphics, and Im-
age Processing, 39(1):116–130.
MacQueen, J. et al. (1967). Some methods for classification
and analysis of multivariate observations. In Proc. 5th
Berkeley Symp. on Math. Stats. and Prob., volume 1,
pages 281–297. Oakland, CA, USA.
Micusik, B. and Pajdla, T. (2003). Estimation of omnidirec-
tional camera model from epipolar geometry. In IEEE
CVPR, volume 1, pages I–485.
Moghadam, P. and Dong, J. F. (2012). Road direction detec-
tion based on vanishing-point tracking. In IEEE/RSJ
IROS, pages 1553–1560.
Moghadam, P., Wijesoma, W. S., and Moratuwage, M.
(2010). Towards a fully-autonomous vision-based ve-
hicle navigation system in outdoor environments. In
ICARCV, pages 597–602.
Ogawa, T. and Takagi, K. (2006). Lane recognition us-
ing on-vehicle lidar. In IEEE Int. Vehic. Symp., pages
540–545.
Rasmussen, C. (2002). Combining laser range, color, and
texture cues for autonomous road following. In IEEE
ICRA, volume 4, pages 4320–4325.
Scaramuzza, D., Martinelli, A., and Siegwart, R. (2006). A
flexible technique for accurate omnidirectional cam-
era calibration and structure from motion. In IEEE
ICVS, pages 45–45.
Thrun, S., Montemerlo, M., Dahlkamp, H., Stavens, D.,
Aron, A., Diebel, J., Fong, P., Gale, J., Halpenny,
M., Hoffmann, G., et al. (2006). Stanley: The robot
that won the darpa grand challenge. J. Field Robot.,
23(9):661–692.
Torr, P. H. and Zisserman, A. (2000). Mlesac: A new robust
estimator with application to estimating image geom-
etry. CVIU, 78(1):138–156.
velodyne (2016). The Velodyne 3D Lidar website.
von Reyher, A., Joos, A., and Winner, H. (2005). A lidar-
based approach for near range lane detection. In IEEE
Intel. Vehic. Symp., pages 147–152.
Waxman, A., Moigne, J., and Srinivasan, B. (1985). Visual
navigation of roadways. In IEEE ICRA, volume 2,
pages 862–867.
Zhang, W. (2010). Lidar-based road and road-edge detec-
tion. In IEEE Int. Vehic. Symp., pages 845–848.
ICINCO 2017 - 14th International Conference on Informatics in Control, Automation and Robotics
430