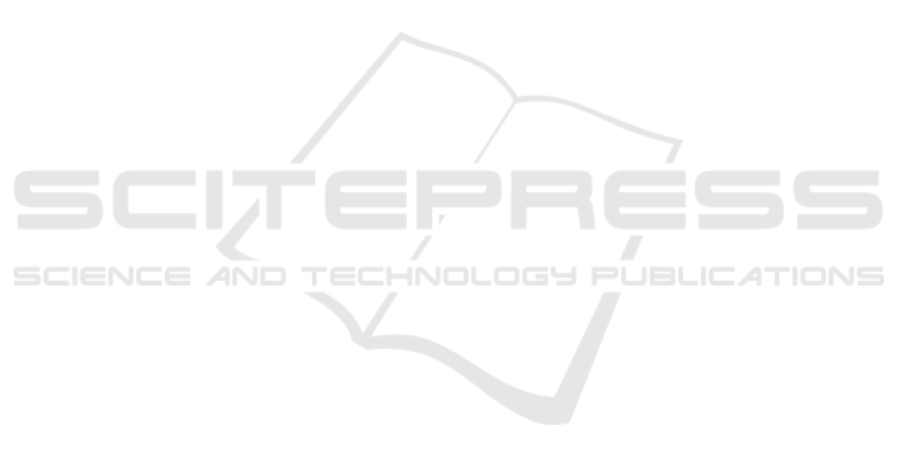
solutions presented in the Pareto front are more
optimistic than the dominated solutions.
The various solutions which belong to the front of
Pareto are optimistic solutions. However, the
decision-maker is provided by the search of the best
compromise solution between the goals, which is
included on the Pareto Front. The CPLEX tool uses
the dynamic mixed-integer programming (MIP) as a
search method, the balance optimality, and feasibility
MIP emphasis. Indeed, the relaxation solution
obtained by a Branch and Cut algorithm through a
deterministic parallel mode, uses up to 4 threads.
The definition of Pareto optimality is similar to
that of efficiency, and a Pareto optimal point in the
criterion space is often considered the same as a non-
dominated point. Therefore, a solution is considered
as Pareto optimal, in a multi-objective minimization
problem, if there exists no other feasible solution
which would decrease some criteria without causing
a simultaneous increase in at least one criterion. This
set also called Pareto front helps the decision maker
to identify the best compromise solution by an
elimination of inferior ones. Then, the retained
solution as elite is the one which has the best
compromise between all the objectives.
5 DISCUSSION AND COMMENTS
According to the tests achieved for our work, giving
the priority to the first objective by minimizing the
total cost is much better than giving the executing
priority of the total duration which perfectly complies
with the third objective that respects the arriving at a
time window. Therefore, we note that the first
objective significantly improves the results as shown
in the Appendix.
For real case problems, the mathematical
formulation is not always reliable for a user, since we
cannot consider fixed rules for all possible
alternatives and treated cases associated with each
customer. According to the experts of a transit
company, the choice of transportation mode is
depends on the several factors, such as customer
requirements, the nature, and characteristics of the
goods, that can be a major condition for the selection
of the mode's problem. There are several features of
the goods, such as the expensive, bulky or perishable
goods, also the goods category or type such as
dangerous, fragile, light, stackable or non-stackable
goods, etc.
On the basis of the promising findings presented in
this paper, further research will be needed to solve
more optimization constraints. Artificial intelligence
approaches to the issue are still required. (Bouamama,
2010) proposed a multi-agent approach based on a
dynamic, distributed Practical Swarm Optimization
algorithm, which is proven to be useful for hard
optimization problems. (Mathlouthi and Bouamama,
2015) proposed two new approaches, a centralized
and distributed honey-bee optimization, enhanced by
a new parameter called local optimum detector. These
two approaches are applied to solve the maximal
constraint satisfaction problems.
6 CONCLUSIONS AND FUTURE
WORKS
This paper presents a new mathematical model in
order to solve multi-modal transport problems that
satisfy multiple objectives according to several
criteria. The proposed multi-objective model is
defined by three objectives, the minimization of the
total cost, and the total duration of an itinerary while
respecting the arriving of goods to a customer at the
time window.
In fact, the following conclusions can be drawn
as: Firstly, an improvement over the literature model
was achieved in order to find feasible solutions.
Secondly, a validation of our proposed model by
implementation. Thirdly, we summarized our work
by computation's test and experimentation results in
order to prove the efficiency of our model.
This paper presents a decision method based on a
mathematical model which plays a significant role in
resolving the transportation problem. Although there
has been a fruitful development of models and
solution techniques to solve this problem by a
relevant decision in transport networks, many future
pieces of research prospects are still missing, such as
the following:
There are still opportunities for integrating
problems that can be solved separately, by using a
multi-criteria analysis approach.
The development of robust, or dynamic
approaches used to solve a planning problem.
The consideration of several types of
products, around on the corresponding product’s
cluster with the same characteristics, since the
choice of the transport mode depends on the
product volume and the value associated with
each product.
The activation or cancellation of a goal according
to customer wishes by adding weights to each
objective, according to the client's need.
A Multi-objective Mathematical Model for Problems Optimization in Multi-modal Transportation Network
357