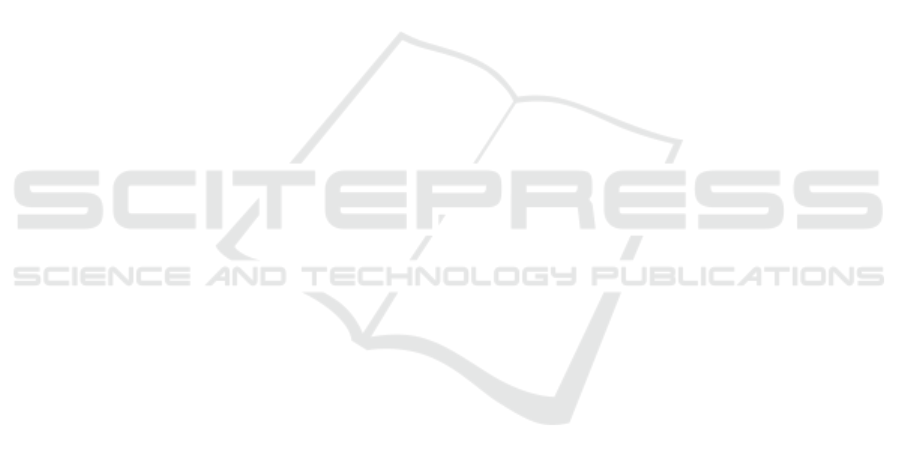
5 CONCLUSION
In this paper, a new controlling strategy PID based
on PSO algorithm was designed in order to control
the muscle force during stimulation sessions. this de-
veloped method is used to compute automatically the
stimulus pulse amplitude for each pulse applied to the
muscle. Also, using experimental data, the PSO algo-
rithm was explored to identify and provide an excel-
lent mathematical model that can simulate perfectly
the muscle response and as a result improved the con-
trol system. With regard to our current results, we
can conclude that designed control method based on
optimization approach can enhance performances of
control FES systems.
REFERENCES
Alfi, A. and Modares, H. (2011). System identification and
control using adaptive particle swarm optimization.
Applied Mathematical Modelling, 35(3):1210–1221.
Ben Hmed, A., Bakir, T., Binczak, S., and Sakly, A.
(2016). Model free control for muscular force by func-
tional electrical stimulation using pulse width modu-
lation. In Control Engineering & Information Tech-
nology(CEIT), 2016 4th International Conference on.
IEEE.
Ben Hmed, A., Bakir, T., Sakly, A., and Binczak, S. (2015).
Controlling muscular force by functional electrical
stimulation using intelligent pid. In Sciences and
Techniques of Automatic Control and Computer En-
gineering (STA), 2015 16th International Conference
on, pages 75–79. IEEE.
Bickel, C. S., Gregory, C. M., and Dean, J. C. (2011). Mo-
tor unit recruitment during neuromuscular electrical
stimulation: a critical appraisal. European journal of
applied physiology, 111(10):2399.
Bouallègue, S., Haggège, J., Ayadi, M., and Benrejeb, M.
(2012). Pid-type fuzzy logic controller tuning based
on particle swarm optimization. Engineering Appli-
cations of Artificial Intelligence, 25(3):484–493.
Ding, J., Chou, L.-W., Kesar, T. M., Lee, S. C., John-
ston, T. E., Wexler, A. S., and Binder-Macleod,
S. A. (2007). Mathematical model that predicts the
force–intensity and force–frequency relationships af-
ter spinal cord injuries. Muscle & nerve, 36(2):214–
222.
Ding, J., Wexler, A. S., and Binder-Macleod, S. A. (2000).
Development of a mathematical model that predicts
optimal muscle activation patterns by using brief
trains. Journal of applied Physiology, 88(3):917–925.
Ding, J., Wexler, A. S., and Binder-Macleod, S. A. (2003).
Mathematical models for fatigue minimization dur-
ing functional electrical stimulation. Journal of Elec-
tromyography and Kinesiology, 13(6):575–588.
Doll, B. D., Kirsch, N. A., and Sharma, N. (2015). Op-
timization of a stimulation train based on a pre-
dictive model of muscle force and fatigue. IFAC-
PapersOnLine, 48(20):338–342.
Ferrarin, M., D’acquisto, E., Mingrino, A., and Pedotti,
A. (1996). An experimental pid controller for knee
movement restoration with closed loop fes system. In
Engineering in Medicine and Biology Society, 1996.
Bridging Disciplines for Biomedicine. Proceedings of
the 18th Annual International Conference of the IEEE,
volume 1, pages 453–454. IEEE.
Jaen-Cuellar, A. Y., de J. Romero-Troncoso, R., Morales-
Velazquez, L., and Osornio-Rios, R. A. (2013). Pid-
controller tuning optimization with genetic algorithms
in servo systems. International Journal of Advanced
Robotic Systems, 10(9):324.
Kurosawa, K., Futami, R., Watanabe, T., and Hoshimiya, N.
(2005). Joint angle control by fes using a feedback er-
ror learning controller. IEEE Transactions on Neural
Systems and Rehabilitation Engineering, 13(3):359–
371.
Law, L. A. F. and Shields, R. K. (2006). Predicting human
chronically paralyzed muscle force: a comparison of
three mathematical models. Journal of applied physi-
ology, 100(3):1027–1036.
Li, Z., Hayashibe, M., Andreu, D., and Guiraud, D. (2015).
Real-time closed-loop fes control of muscle activation
with evoked emg feedback. In Neural Engineering
(NER), 2015 7th International IEEE/EMBS Confer-
ence on, pages 623–626. IEEE.
Liu, C.-L., Yu, C.-h., Chen, S.-C., and Chen, C.-H. (2005).
Evaluation of closed-loop control system for restoring
standing and sitting functions by functional electrical
stimulation. Biomedical Engineering: Applications,
Basis and Communications, 17(01):19–26.
Nagaraj, B. and Vijayakumar, P. (2011). A comparative
study of pid controller tuning using ga, ep, pso and
aco. Journal of Automation Mobile Robotics and In-
telligent Systems, 5:42–48.
Precup, R.-E., David, R.-C., Petriu, E. M., Preitl, S., and
R
˘
adac, M.-B. (2014). Novel adaptive charged sys-
tem search algorithm for optimal tuning of fuzzy
controllers. Expert Systems with Applications,
41(4):1168–1175.
Qiu, S., He, F., Tang, J., Xu, J., Zhang, L., Zhao, X., Qi, H.,
Zhou, P., Cheng, X., Wan, B., et al. (2014). Intelli-
gent algorithm tuning pid method of function electri-
cal stimulation using knee joint angle. In Engineering
in Medicine and Biology Society (EMBC), 2014 36th
Annual International Conference of the IEEE, pages
2561–2564. IEEE.
Türk¸sen, Ö. and Tez, M. (2016). An application of nelder-
mead heuristic-based hybrid algorithms: Estimation
of compartment model parameters. International
Journal of Artificial Intelligence, 14(1):112–129.
Yochum, M., Bakir, T., Lepers, R., and Binczak, S. (2012).
Estimation of muscular fatigue under electromyostim-
ulation using cwt. IEEE Transactions on Biomedical
Engineering, 59(12):3372–3378.
A Novel Strategy for Adjusting Current Pulse Amplitude of FES-Systems with PID based on PSO Algorithm Method to Control the Muscle
Force
669