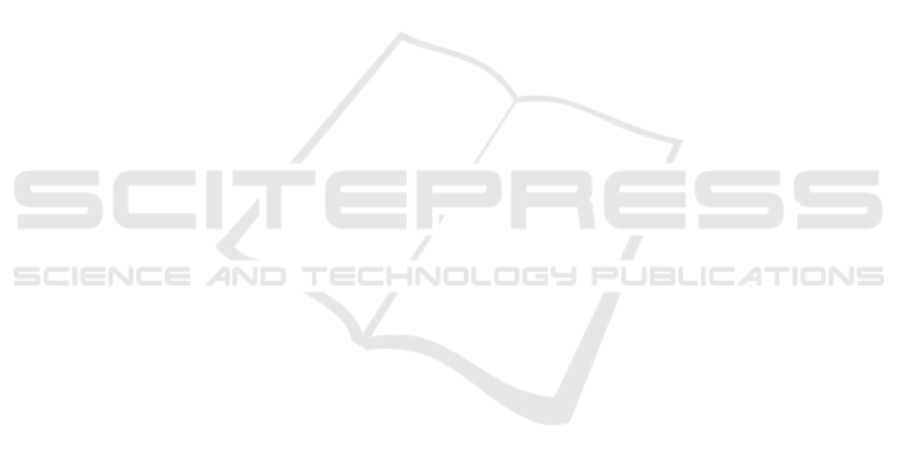
smartphone. Both ensure him a full tracking of his
personal activity data. He has a Twitter account and
he is follower of many sportive man, personal coach,
instructor because he wants to stay well informed
about all news in the field.
Lucy
is always watching her weight. She likes to
be in fit and to eat biologic food, she likes read about
nutrient information on food, herbs’ properties, cos-
metics’ use and so on. To accomplish her goal, she is
used to adopt a series of mobile apps to track about
food, sports and to stay informed about these top-
ics. On Twitter, for instance, she follows famous ac-
tresses, personal trainers and nutritionists to get well
informed about these topics.
Steven
is a student. He spends a lot of time stay-
ing sit and working on the laptop. He is worried about
assuming a right posture then he had bought a pin able
to give a vibration whenever his posture is wrong or
he is staying to long. He has a Twitter account and he
is follower of healthy products in general: from pos-
ture, to food, to sports and so on. He does not like to
practice sports.
By following the interest assortativity approach,
we found out ’health’ like a common macro-interest,
pushing on this topic of interest, we are able to sug-
gest friendship between these components supporting
their owners in achieving their goals, however they
look like truly different. In fact,
Francis
and
Lucy
have never considered the importance of a right pos-
ture for the wellbeing; conversely,
Steven
does not
take care enough about sports and food, while he is a
very sedentary person. What the new approach allows
is the establishment of friendship between this series
of devices to allow the full accomplishment of per-
sonal goals of everybody, suggesting them new ones
and novel ways to accomplish the same results.
This is discussed in the next section, in which we
show that, in the cases like those described above,
the knowledge typically used to establish a connec-
tion between objects would produce unsatisfactory re-
sults. For example, by considering proximity (i.e., the
fact that the objects meet each other a given number
of times with a sufficient frequency), we should con-
clude that there is no reason to connect the above ob-
jects. Indeed, probably, the above objects never meet.
1.2 Structure of the Paper
The plan of this paper is as follows. In Section 2,
we present our approach to chose links in a IoT envi-
ronment. Section 3 describes the preliminary experi-
mentation carried out to study the effectiveness of our
technique. Section 4 deals with literature related to
our work. Finally, in Section 5, we draw our conclu-
sions.
2 DISCOVERING GOOD LINKS
According to the Internet-of-Thing paradigm, an en-
tity on a network has to be notified of the availabil-
ity of desirable services or devices on the network in
order to form a link. Typically, the fact that two ob-
jects get in touch somewhere and sometimes (maybe
because the corresponding owners meet in a certain
location) is enough to trigger (with a given thresh-
old) the establishment of a link between the two ob-
jects (Union, 2005; Zhang et al., 2011; Evangelos A
et al., 2011). This property is called proximity. The
aim of this section is to identify possible enhanced
ways to discover potentially beneficial links. To do
this, preliminarily, we consider which are the candi-
date properties (existing in the literature) we can use
to build a more complex model. They are: (i) prox-
imity, (ii) homogeneity, i.e., they are the same kind of
object created by the same manufacturer; (iii) owner-
ship, i.e., they belong to the same user; (iv) friendship,
i.e., owners are mutual friends in a social network.
Arguing that the decision regarding the insertion
of a link between two objects could rely on a mix of
the above properties, we define a decision function to
decide whether a link between two objects ⟨x,y⟩ has
to be inserted or not.
Observe that all the above properties give us some
information about the direct relationship between two
objects. Our proposal aims to use also some indirect
knowledge coming from the social network of own-
ers to support the computation of the above decision
function (Buccafurri et al., 2016b). In addition, de-
spite the classical selection criteria that are based on
the sole proximity, we use all the above direct prop-
erties. Therefore, we introduce two measures, which
we combine to compute the aimed decision function.
These are:
1. T
dir
x,y
, which derives from the direct knowledge
about objects and owners, and
2. T
ind
x,y
, which encodes some indirect knowledge.
As indirect knowledge we exploit a recently
proven property occurring in online social networks,
called interest assortativity (Buccafurri et al., 2016a).
According to this result, it is possible to have a mea-
sure of the correlation between a given human interest
and the presence of links between humans.
To exploit the above indirect property, we need to
match objects to humans, in such a way that interests
are someway preserved. To do this, we define a taxon-
omy on top of the properties and/or aims of objects.
Discovering Good Links Between Objects in the Internet of Things
103