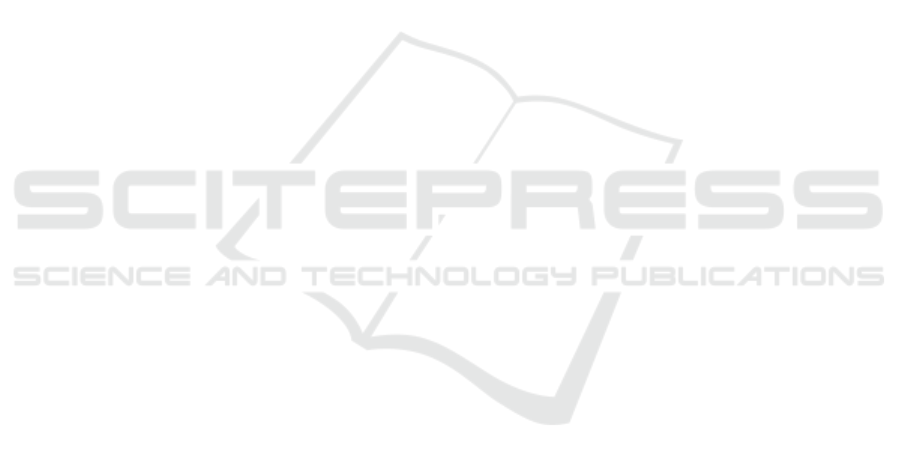
query in order to maximize accuracy, the trivial au-
tomatic mapping still guarantees interesting improve-
ments over the use of the plain search engine.
The method could be refined in many ways. In this
work we used simple and well-established procedures
to extract knowledge from hierarchically-organized
local documents and to expand search queries: the ex-
perimentation of more advanced techniques may lead
to improvements of the proposed general method.
While we focused on enhancing user’s queries, the
personalization system could also work on the out-
put of the underlying search engine, filtering or re-
ordering results better matching known user’s inter-
ests: this approach could be used in substitution of or
even in combination with query expansion, exploiting
the same user model. Finally, methods could be de-
vised to make use of local user’s documents alongside
other information sources, such as search or browsing
history.
REFERENCES
Carpineto, C. and Romano, G. (2012). A survey of auto-
matic query expansion in information retrieval. ACM
Computing Surveys (CSUR), 44(1):1.
Chapelle, O., Metlzer, D., Zhang, Y., and Grinspan, P.
(2009). Expected reciprocal rank for graded rele-
vance. In Proceedings of the 18th ACM conference on
Information and knowledge management, pages 621–
630. ACM.
Chirita, P.-A., Firan, C. S., and Nejdl, W. (2007). Person-
alized query expansion for the web. In Proceedings
of the 30th annual international ACM SIGIR confer-
ence on Research and development in information re-
trieval, pages 7–14. ACM.
Domeniconi, G., Moro, G., Pagliarani, A., and Pasolini, R.
(2017). On deep learning in cross-domain sentiment
classification. In Proceedings of the 9th International
Joint Conference on Knowledge Discovery, Knowl-
edge Engineering and Knowledge Management.
Domeniconi, G., Moro, G., Pasolini, R., and Sartori, C.
(2016). A comparison of term weighting schemes for
text classification and sentiment analysis with a super-
vised variant of tf.idf. In Data Management Technolo-
gies and Applications (DATA 2015), Revised Selected
Papers, volume 553, pages 39–58. Springer.
Gauch, S., Chaffee, J., and Pretschner, A. (2003). Ontology-
based personalized search and browsing. Web Intelli-
gence and Agent Systems: An international Journal,
1(3, 4):219–234.
Gauch, S., Speretta, M., Chandramouli, A., and Micarelli,
A. (2007). User profiles for personalized information
access. In The adaptive web, pages 54–89. Springer.
Ghorab, M. R., Zhou, D., OConnor, A., and Wade, V.
(2013). Personalised information retrieval: survey and
classification. User Modeling and User-Adapted In-
teraction, 23(4):381–443.
Jansen, B. J., Spink, A., and Saracevic, T. (2000). Real
life, real users, and real needs: a study and analysis
of user queries on the web. Information processing &
management, 36(2):207–227.
Liu, F., Yu, C., and Meng, W. (2002). Personalized web
search by mapping user queries to categories. In Pro-
ceedings of the eleventh international conference on
Information and knowledge management, pages 558–
565. ACM.
Micarelli, A. and Sciarrone, F. (2004). Anatomy and empir-
ical evaluation of an adaptive web-based information
filtering system. User Modeling and User-Adapted
Interaction, 14(2-3):159–200.
Pitkow, J., Sch
¨
utze, H., Cass, T., Cooley, R., Turnbull, D.,
Edmonds, A., Adar, E., and Breuel, T. (2002). Person-
alized search. Communications of the ACM, 45(9):50–
55.
Pretschner, A. and Gauch, S. (1999). Ontology based per-
sonalized search. In Tools with Artificial Intelligence,
1999. Proceedings. 11th IEEE International Confer-
ence on, pages 391–398. IEEE.
Salton, G. and Buckley, C. (1997). Improving retrieval per-
formance by relevance feedback. Readings in infor-
mation retrieval, 24(5):355–363.
Speretta, M. and Gauch, S. (2005). Personalized search
based on user search histories. In Web Intelligence,
2005. Proceedings. The 2005 IEEE/WIC/ACM Inter-
national Conference on, pages 622–628. IEEE.
Stamou, S. and Ntoulas, A. (2009). Search personalization
through query and page topical analysis. User Model-
ing and User-Adapted Interaction, 19(1-2):5–33.
Steichen, B., Ashman, H., and Wade, V. (2012). A compar-
ative survey of personalised information retrieval and
adaptive hypermedia techniques. Information Pro-
cessing & Management, 48(4):698–724.
Sugiyama, K., Hatano, K., and Yoshikawa, M. (2004).
Adaptive web search based on user profile constructed
without any effort from users. In Proceedings of the
13th international conference on World Wide Web,
pages 675–684. ACM.
Teevan, J., Dumais, S. T., and Horvitz, E. (2005). Per-
sonalizing search via automated analysis of interests
and activities. In Proceedings of the 28th annual in-
ternational ACM SIGIR conference on Research and
development in information retrieval, pages 449–456.
ACM.
Trajkova, J. and Gauch, S. (2004). Improving ontology-
based user profiles. In Proceedings of RIAO 2004,
pages 380–390.
Zhou, D., Lawless, S., and Wade, V. (2012). Improving
search via personalized query expansion using social
media. Information retrieval, 15(3-4):218–242.