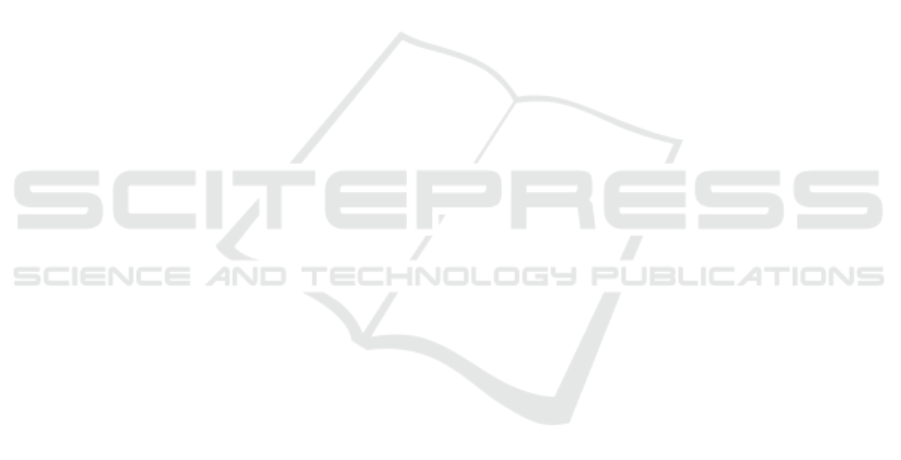
2.2 Data Collection
The speech FFRs of 22 (11F, 11M) normal-hearing
adult subjects (20-35 years) to four 100ms synthetic
English vowel stimuli with F0=100Hz
(F1:/a/=700Hz, /ɔ/=600Hz, /U/=500Hz, /u/=300Hz)
at four levels (55, 65, 75, 85 dBA) were recorded.
Repeated-measures ANOVAs were performed on
the RMS-amplitude of the spectral data for F0 and
then for the combination of its next five harmonics
(H2 to H6) in the eFFR, and for F1 and then for the
combination of F1 and one harmonic on either side
of it in the sFFR. Post-hoc pairwise comparisons
with Holm-Bonferonni corrections were also
performed.
This baseline dataset will be used with an
automatic classification task before proceeding to
speech FFRs collected from hearing impaired
subjects and implementing the full BCI.
3 RESULTS
Significant effects of level were found at F0 in the
eFFR and at F1 in the sFFR (p<0.01 in all cases).
The combined harmonics show effects of level
(p<0.001 in all cases), as well as significant pairwise
comparisons between most levels. Interestingly,
although F0 exhibits change in the eFFR across all
four of the vowel stimuli, its amplitude does not
grow consistently with increasing level, and it in fact
appears to saturate at 65 dBA and then decrease in
amplitude in all of the vowel stimuli except for /u/,
which exhibits strictly increasing growth.
The trend that emerges is one of increasing
spectral richness with increasing level via the growth
of the harmonics of F0 in the eFFR and via the
growth of the first formant and its related harmonics
in the sFFR.
Initial machine learning models aimed at
exploiting these trends appear promising. Using a
10-fold cross-validation technique, a support-vector
machine was trained and tested on a mix of features
from both the eFFR and sFFR. A mean vowel
classification accuracy of 80.5% was achieved when
the model was restricted to the 85dBA case, which is
comparable to the result reported by Sadeghian et al.
(2015). Additionally, a correlative algorithm that
was used to predict sound level across all vowel
categories yielded a 2.2-fold increase in
accuracy versus the null model, and a 79% reduction
in misclassifications of levels greater than 10dB
from the target. Since the spectra of within-subject
test-retest trials are highly correlated (ranging from
r=0.79 to r=0.96 across all vowels), we would
expect much higher classification accuracy on
models trained solely on individual subjects,
provided that enough individual data is recorded.
4 DISCUSSION
These findings suggest that effects of sound level
can be observed in the speech FFR of normal
hearing adults, both with respect to the neural
encoding of the envelope and the spectral fine
structure of the speech signal. Machine learning
techniques can be used to automatically classify
vowels and sound level, particularly in individual
subjects. This approach will be used to tune hearing
aids to maximize the separation between the
responses to different vowels and levels, with the
aim of improving perceptual discrimination and
loudness control in hearing aid users.
ACKNOWLEDGEMENTS
Funding provided by the Natural Sciences and
Engineering Research Council of Canada.
REFERENCES
Anderson S., Parbery-Clark A., White-Schwoch T.,
Drehob S., Kraus N. (2013). Effects of hearing loss on
the subcortical representation of speech cues. Journal
of the Acoustical Society of America, 133(5), 3030-
3038.
Billings, C. J., Tremblay, K. L., & Miller, C. W. (2011).
Aided cortical auditory evoked potentials in response
to changes in hearing aid gain. International Journal
of Audiology, 50(7), 459–467.
Dajani, H. R., Heffernan, B. P., & Giguere, C. (2013).
Improving hearing aid fitting using the speech-evoked
auditory brainstem response. In Proceedings of the
Annual International Conference of the IEEE
Engineering in Medicine and Biology Society
(EMBC), pp. 2812–2815.
Giguère C., & Smoorenburg G. F. (1999). Computational
modeling of outer haircell damage: Implications for
hearing aid signal processing. In Psychoacoustics,
Physiology, and Models of Hearing, edited by Dau T.,
Hohmann V., & Kollmeier B., World Scientific,
Singapore, 1999.
Kraus, N., & Anderson, S. (2012). Hearing Matters: cABR
May Improve Hearing Aid Outcomes. The Hearing
Journal, 65(11), 56.
Sadeghian, A., Dajani, H. R., & Chan, A. D. C. (2015).
Classification of speech-evoked brainstem responses
to English vowels. Speech Communication, 68, 69–84.