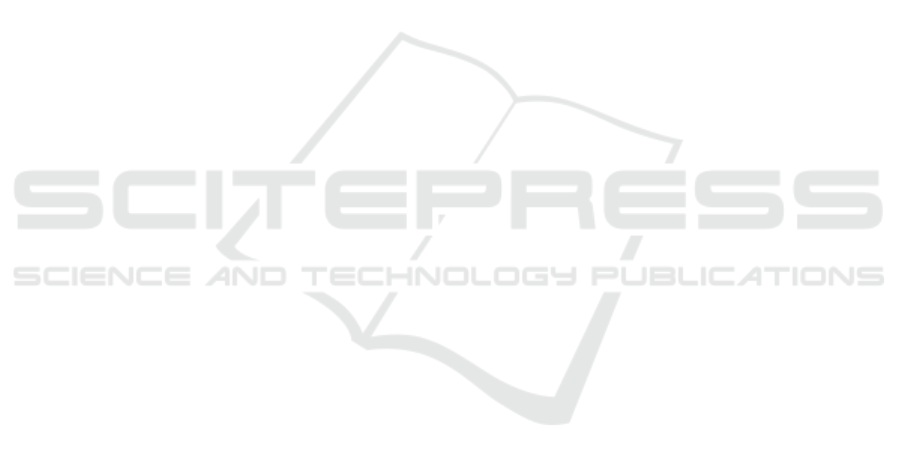
gram analysis and dynamic artificial neural network.
Expert Systems with Applications.
Gid
´
ofalvi, G. and Elkan, C. (2001). Using news articles to
predict stock price movements. Department of Com-
puter Science and Engineering, University of Califor-
nia, San Diego.
Gilbert, E. and Karahalios, K. (2010). Widespread worry
and the stock market. In ICWSM, pages 59–65.
Gruhl, D., Guha, R., Kumar, R., Novak, J., and Tomkins, A.
(2005). The predictive power of online chatter. In Pro-
ceedings of the eleventh ACM SIGKDD international
conference on Knowledge discovery in data mining,
pages 78–87. ACM.
Kimoto, T., Asakawa, K., Yoda, M., and Takeoka, M.
(1990). Stock market prediction system with modu-
lar neural networks. In Neural Networks, 1990., 1990
IJCNN International Joint Conference on, pages 1–6.
IEEE.
Li, X., Wang, C., Dong, J., Wang, F., Deng, X., and
Zhu, S. (2011). Improving stock market prediction
by integrating both market news and stock prices. In
Hameurlain, A., Liddle, S., Schewe, K.-D., and Zhou,
X., editors, Database and Expert Systems Applica-
tions, volume 6861 of Lecture Notes in Computer Sci-
ence, pages 279–293. Springer Berlin Heidelberg.
Lin, M.-C., Lee, A. J. T., Kao, R.-T., and Chen, K.-T.
(2008). Stock price movement prediction using repre-
sentative prototypes of financial reports. ACM Trans.
Manage. Inf. Syst., 2(3):19:1–19:18.
Liu, Y., Huang, X., An, A., and Yu, X. (2007). Arsa:
a sentiment-aware model for predicting sales perfor-
mance using blogs. In Proceedings of the 30th annual
international ACM SIGIR conference on Research and
development in information retrieval, pages 607–614.
ACM.
Lo, A. W. and MacKinlay, A. C. (1988). Stock market
prices do not follow random walks: Evidence from a
simple specification test. Review of financial studies,
1(1):41–66.
Malkiel, B. G. (2003). The efficient market hypothesis
and its critics. The Journal of Economic Perspectives,
17(1):59–82.
Mao, H., Counts, S., and Bollen, J. (2011). Predicting fi-
nancial markets: Comparing survey, news, twitter and
search engine data. arXiv preprint arXiv:1112.1051.
Mao, Y., Wei, W., and Wang, B. (2013). Twitter volume
spikes: analysis and application in stock trading. In
Proceedings of the 7th Workshop on Social Network
Mining and Analysis, page 4. ACM.
Mao, Y., Wei, W., Wang, B., and Liu, B. (2012). Correlating
s&p 500 stocks with twitter data. In Proceedings of
the First ACM International Workshop on Hot Topics
on Interdisciplinary Social Networks Research, Hot-
Social ’12, pages 69–72, New York, NY, USA. ACM.
Markou, M. and Singh, S. (2003). Novelty detection: a re-
view?part 1: statistical approaches. Signal processing,
83(12):2481–2497.
Mishne, G. and de Rijke, M. (2006). Capturing global mood
levels using blog posts. In AAAI Spring Symposium:
Computational Approaches to Analyzing Weblogs’06,
pages 145–152.
Mittal, A. and Goel, A. (2012). Stock prediction using twit-
ter sentiment analysis.
Mittermayer, M.-A. and Knolmayer, G. (2006). Text mining
systems for market response to news: A survey. Insti-
tut f
¨
ur Wirtschaftsinformatik der Universit
¨
at Bern.
Oliveira, N., Cortez, P., and Areal, N. (2013). Some ex-
periments on modeling stock market behavior using
investor sentiment analysis and posting volume from
twitter. In Proceedings of the 3rd International Con-
ference on Web Intelligence, Mining and Semantics,
WIMS ’13, pages 31:1–31:8, New York, NY, USA.
ACM.
Porshnev, A., Redkin, I., and Shevchenko, A. (2013). Im-
proving prediction of stock market indices by analyz-
ing the psychological states of twitter users. HSE
Working papers WP BRP 22/FE/2013, National Re-
search University Higher School of Economics.
Qian, B. and Rasheed, K. (2007). Stock market predic-
tion with multiple classifiers. Applied Intelligence,
26(1):25–33.
Rao, T. and Srivastava, S. (2012). Twitter sentiment anal-
ysis: How to hedge your bets in the stock markets.
CoRR, abs/1212.1107.
Ruiz, E. J., Hristidis, V., Castillo, C., Gionis, A., and
Jaimes, A. (2012). Correlating financial time series
with micro-blogging activity. In Proceedings of the
Fifth ACM International Conference on Web Search
and Data Mining, WSDM ’12, pages 513–522, New
York, NY, USA. ACM.
Samant, R. M. and Rao, S. (2011). The effect of noise in
automatic text classification. In Proceedings of the In-
ternational Conference & Workshop on Emerging
Trends in Technology, ICWET ’11, pages 557–558,
New York, NY, USA. ACM.
Schumaker, R. P. and Chen, H. (2006). Textual analysis
of stock market prediction using financial news. In
Americas Conference on Information Systems.
Sprenger, T. O., Tumasjan, A., Sandner, P. G., and Welpe,
I. M. (2013). Tweets and trades: The information con-
tent of stock microblogs. European Financial Man-
agement.
Wilson, T., Hoffmann, P., Somasundaran, S., Kessler, J.,
Wiebe, J., Choi, Y., Cardie, C., Riloff, E., and Pat-
wardhan, S. (2005). Opinionfinder: A system for sub-
jectivity analysis. In Proceedings of HLT/EMNLP on
Interactive Demonstrations, pages 34–35. Association
for Computational Linguistics.
Yang, Y. (1995). Noise reduction in a statistical approach to
text categorization. In Proceedings of the 18th annual
international ACM SIGIR conference on Research and
development in information retrieval, pages 256–263.
ACM.
Zhang, X., Fuehres, H., and Gloor, P. A. (2011). Predicting
stock market indicators through twitter ”i hope it is
not as bad as i fear”. Procedia-Social and Behavioral
Sciences, 26:55–62.