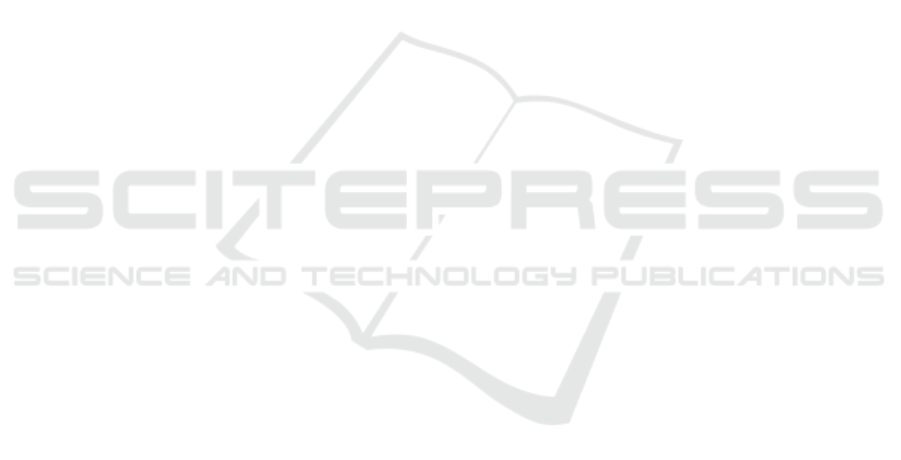
ducted study in the bank, MLP is implemented and
we obtained better accuracy by using RF. Obtained
results indicate that customer repayment behaviour
on other products along with other information types
need to be investigated further for fully understand the
SMEs risk factors.
6 FUTURE WORK
As experimental results indicated, adding behaviour
data on frequently preferred products improves per-
formance over using other information types alone.
Therefore, as a future work, it is aimed to enhance
product based features by adding new product types
which will be determined according to their usage
rate. Moreover, after information types which play an
important role for SMEs risk analysis are determined,
it is planned to observe customer behaviour for an in-
terval by shifting the observation point and accord-
ing to outputs, customer status will be updated. It is
expected that, forecasting customers who will fall in
NPL on next month is likely to be easier than forecast-
ing customers who will default after 6 months. There-
fore, enriching performance data adding behaviour
on next months will provide better analysis of SMEs
credit risk. It is planned that parameter tuning pro-
cess for each machine learning algorithm to be imple-
mented at production deployment stage, since up-to-
date data in high quantities will only be available on
the deployment database.
REFERENCES
Altman, E. I. and Sabato, G. (2005). Modeling credit risk
for smes: evidence from the us market.
Banasik, J., Crook, J. N., and Thomas, L. C. (1999). Not if
but when will borrowers default. Journal of the Oper-
ational Research Society, 50(12):1185–1190.
Breiman, L. (2001). Random forests. Mach. Learn.,
45(1):5–32.
Cortes, C. and Vapnik, V. (1995). Support-vector networks.
Mach. Learn., 20(3):273–297.
Cox, D. R. (1958). Two further applications of a model for
binary regression. Biometrika, 45(3/4):562–565.
Derelio
˘
glu, G. and G
¨
urgen, F. (2011). Knowledge discov-
ery using neural approach for smes credit risk analysis
problem in turkey. Expert Systems with Applications,
38(8):9313–9318.
Gaganis, C., Pasiouras, F., Spathis, C., and Zopounidis, C.
(2007). A comparison of nearest neighbours, discrim-
inant and logit models for auditing decisions. Intel-
ligent Systems in Accounting, Finance and Manage-
ment, 15(1-2):23–40.
Galindo, J. and Tamayo, P. (2000). Credit risk assessment
using statistical and machine learning: basic method-
ology and risk modeling applications. Computational
Economics, 15(1):107–143.
Harris, T. (2015). Credit scoring using the clustered sup-
port vector machine. Expert Systems with Applica-
tions, 42(2):741–750.
Hsieh, N.-C. and Hung, L.-P. (2010). A data driven en-
semble classifier for credit scoring analysis. Expert
systems with Applications, 37(1):534–545.
Huang, C.-L., Chen, M.-C., and Wang, C.-J. (2007). Credit
scoring with a data mining approach based on support
vector machines. Expert systems with applications,
33(4):847–856.
Kim, H. S. and Sohn, S. Y. (2010). Support vector ma-
chines for default prediction of smes based on tech-
nology credit. European Journal of Operational Re-
search, 201(3):838–846.
Menardi, G. and Torelli, N. (2013). The effect of train-
ing set selection when predicting defaulting small and
medium-sized enterprises with unbalanced data. The
Journal of Credit Risk, 9(4):47.
Noh, H. J., Roh, T. H., and Han, I. (2005). Prognostic per-
sonal credit risk model considering censored informa-
tion. Expert Systems with Applications, 28(4):753–
762.
Sarlija, N., Bensic, M., and Zekic-Susac, M. (2009). Com-
parison procedure of predicting the time to default in
behavioural scoring. Expert Systems with Applica-
tions, 36(5):8778–8788.
Thomas, L. C. (2000). A survey of credit and be-
havioural scoring: forecasting financial risk of lend-
ing to consumers. International journal of forecast-
ing, 16(2):149–172.
Thomas, L. C., Ho, J., and Scherer, W. T. (2001). Time
will tell: behavioural scoring and the dynamics of con-
sumer credit assessment. IMA Journal of Management
Mathematics, 12(1):89–103.
Wang, G., Hao, J., Ma, J., and Jiang, H. (2011). A compar-
ative assessment of ensemble learning for credit scor-
ing. Expert systems with applications, 38(1):223–230.
Wang, W. (2012). How the small and medium-sized en-
terprises owners credit features affect the enterprises-
credit default behavior? E3 Journal of Business Man-
agement and Economics., 3(2):090–095.
Zhou, L., Lai, K. K., and Yu, L. (2010). Least squares sup-
port vector machines ensemble models for credit scor-
ing. Expert Systems with Applications, 37(1):127–
133.