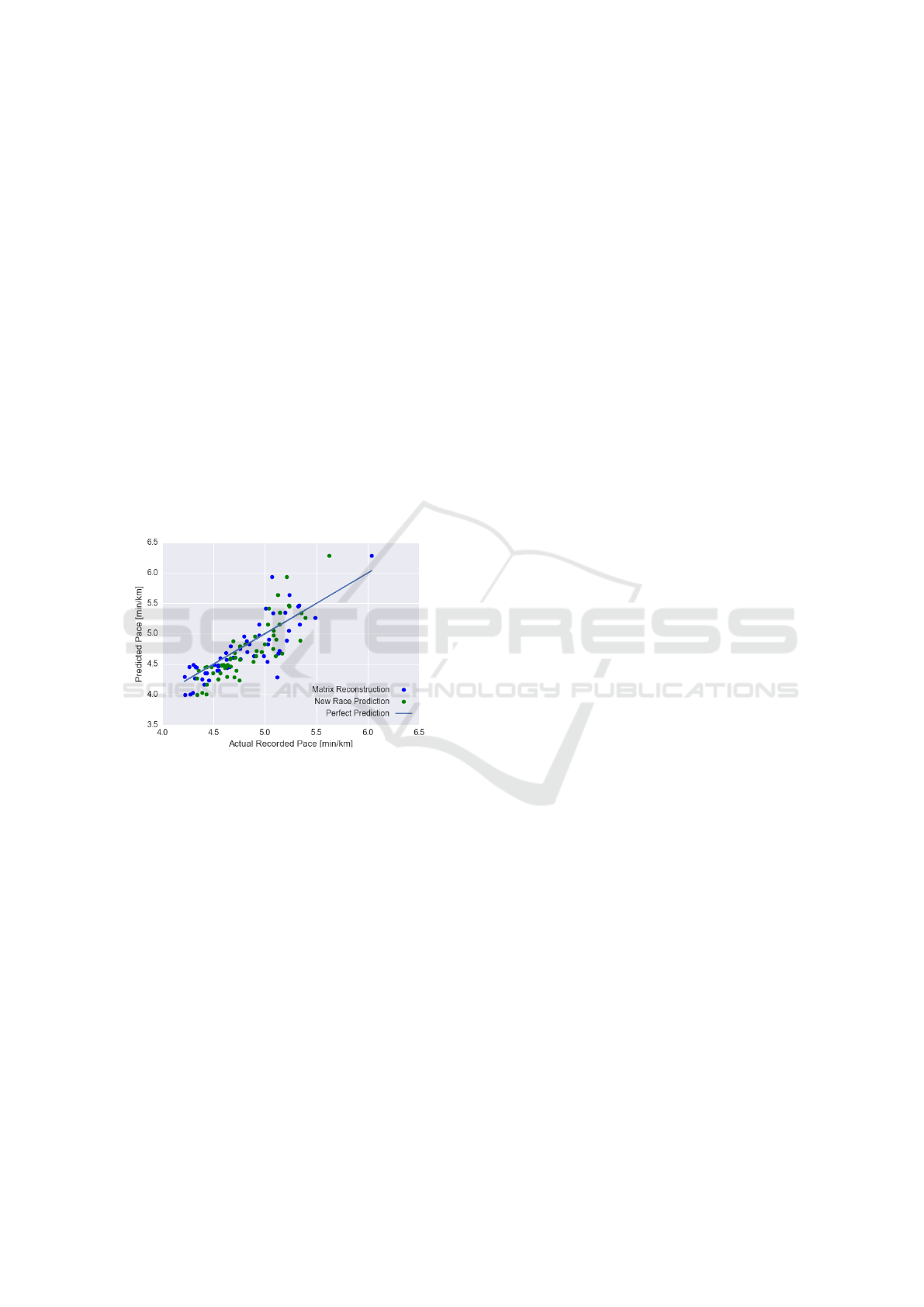
can highly differ from what was expected because of
an injury or simply because the athlete did not try to
achieve is best potential performance.
4.2 Results for Predictions on New
Races
Race time prediction accuracy is measured for new
athletes on new races. For this purpose, it is necessary
to know at least N race times for each athlete and that
the routes itinerary is known to compute their features
(distance and cumulative elevation gain).
A few race results can be used to generate ath-
letes vectors using (5) and route features can give race
vectors through the regression model given by (4).
Pace can then be predicted on known races, and com-
pared to actual race records. In this case, race vec-
tors are unknown and are estimated from route prop-
erties. Therefore, the root mean square error increases
to about 26 seconds per kilometer. Figure 4 shows
race pace predictions versus actual records.
Figure 4: Predictions versus observed race times scatter plot
with known vectors or with estimated ones.
5 CONCLUSION
This paper provides tools that can be used to predict
race times. This is of interest for athlete preparation,
for workout route planning and for race events organi-
zation. The same tools can also serve to compare dif-
ferent athlete performances and to track athlete level
over time.
Experiments show that the methodology is appli-
cable to real data and gives meaningful results. This
work will be continued in different directions. First,
only the two most commonly used route features were
used (distance and cumulative positive elevation gain)
but any function of the elevation profile could lead to
better predictive performances. Other route parame-
ters such as ground type and weather conditions may
also prove to improve time prediction.
Then, race vector elements were assumed to be
a linear function of the race features. Other nonlin-
ear regression models could improve the accuracy as
well. Two different approaches can be pursued.
First, domain knowledge was not considered in
this work. Most probably, accuracy could bene-
fit from well-established physiological or empirical
models; for instance, the relationship between aver-
age race pace and distance has been modeled in other
works by hyperbolic law, power law or nomogram
(P
´
eronnet and Thibault, 1989; Garc
´
ıa-Manso et al.,
2012; Coquart et al., 2015).
A second path to be taken would be to discover
more complex relationships between route features
and race pace through the data itself using model fit-
ting techniques. This approach will probably require
a larger amount of race data.
REFERENCES
Bauer, C. (2013). On the (in-) accuracy of gps measures
of smartphones: a study of running tracking applica-
tions. In Proceedings of International Conference on
Advances in Mobile Computing & Multimedia, page
335. ACM.
Coquart, J. B., Mercier, D., Tabben, M., and Bosquet, L.
(2015). Influence of sex and specialty on the predic-
tion of middle-distance running performances using
the mercier et al.s nomogram. Journal of sports sci-
ences, 33(11):1124–1131.
Ekstrand, M. D., Riedl, J., and Konstan, J. A. (2011).
Collaborative filtering recommender systems. Foun-
dations and Trends in Human-Computer Interaction,
4:175–243.
Garc
´
ıa-Manso, J., Mart
´
ın-Gonz
´
alez, J., Vaamonde, D.,
and Da Silva-Grigoletto, M. (2012). The limitations
of scaling laws in the prediction of performance in
endurance events. Journal of theoretical biology,
300:324–329.
Gemulla, R., Nijkamp, E., Haas, P. J., and Sismanis, Y.
(2011). Large-scale matrix factorization with dis-
tributed stochastic gradient descent. In KDD.
Jain, P., Netrapalli, P., and Sanghavi, S. (2013). Low-rank
matrix completion using alternating minimization. In
Proceedings of the forty-fifth annual ACM symposium
on Theory of computing, pages 665–674. ACM.
James, G., Witten, D., Hastie, T., and Tibshirani, R. (2013).
An introduction to statistical learning. volume 112,
chapter 5, pages 176–186. Springer.
Noakes, T. D., Myburgh, K. H., and Schall, R. (1990). Peak
treadmill running velocity during the v o2 max test
predicts running performance. Journal of sports sci-
ences, 8(1):35–45.
P
´
eronnet, F. and Thibault, G. (1989). Mathematical analy-
sis of running performance and world running records.
Journal of Applied Physiology, 67(1):453–465.